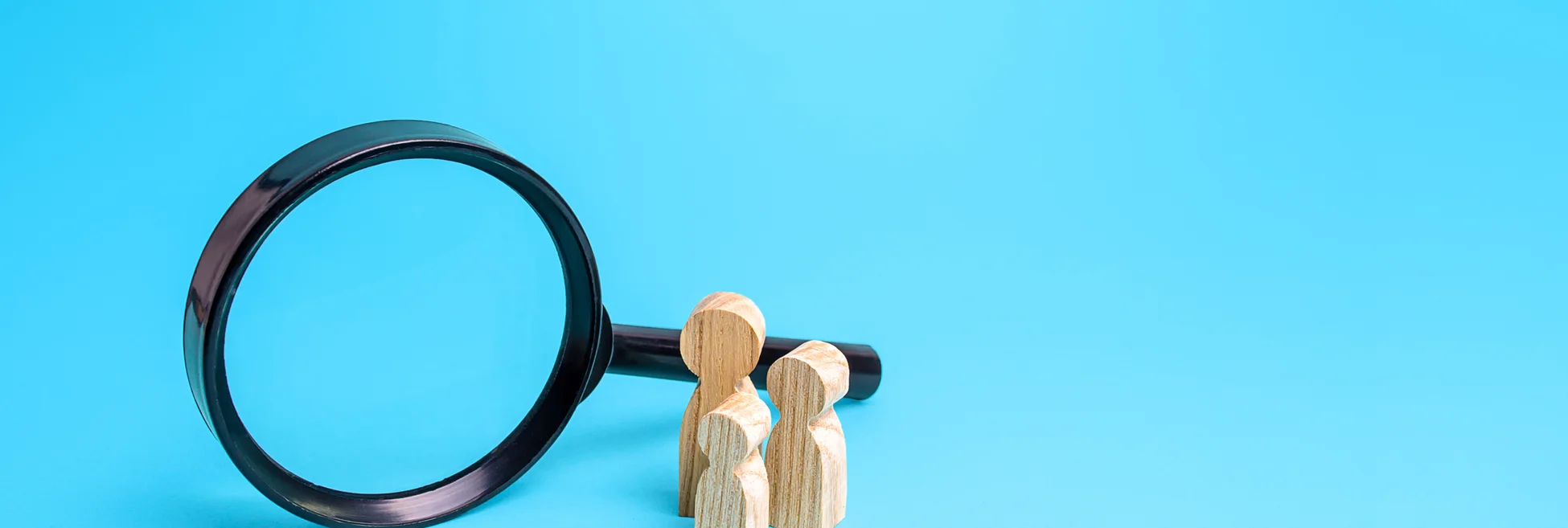
Academics use granular data for futures market predictions
Researchers at NYU’s Courant Institute of Mathematical Sciences are using granular futures data from BMLL for research on less-covered futures markets.
London-based analytics vendor BMLL Technologies is providing granular futures data to New York University’s Mathematics in Finance program. The academics want to run computations on market activity to understand the behaviors and impacts of trades, both buying and selling.
Professor Petter Kolm, a quantitative analyst who researches market microstructure and buy-side trading, leads the research team, which is part of NYU’s Courant Institute of Mathematical Sciences. Kolm’s team has previously focused on the equities market in their research, using artificial intelligence and deep learning to run computations.
Kolm tells WatersTechnology that he now wants to apply the same research methods to futures. “We were particularly interested in taking some of the experience that we have developed over the years in equities and applying it to other markets—specifically the futures market,” he says.
BMLL is providing NYU with futures data sourced from the Intercontinental Exchange (Ice), Eurex, and CME Group. The data includes equity indices, fixed income/government bonds, short-term interest rates, cryptocurrencies, commodities, and foreign exchange. BMLL’s futures data is marketed as Level-3 data, a category given to granular data, some of which is timestamped to the nanosecond. Researchers can analyze individual order behavior by looking at order fill probability, order resting time, and full order book with individual orders and messages. Level-1 data includes T+1 data, including bid and offer, midpoint price, and addressable traded volume, while Level-2 data offers the order book aggregated by price-plus-trade and average execution cost, and liquidity away from the midpoint.
NYU researchers will access the futures data and analytics libraries via file transfer protocol (FTP) from Data Lab, BMLL’s cloud-based Python environment. NYU’s production tool is the Greene supercomputer, which the university unveiled in 2020. Named for the street in Manhattan’s SoHo neighborhood, the computer was built by Lenovo and can do 4 quadrillion calculations per second.
Researchers across the university used the Greene supercomputer for artificial intelligence, virtual reality, climate modeling, computational chemistry and Covid-19 research. Kolm says the power of the computer is key for processing the data his team wants to use. “It’s very important to have a specialized infrastructure for this. PCs are not going to do this, laptops are not going to do it; you need these compute farms that have access to fast disk space, lots of GPUs and CPUs,” he says.
Predicting the future
Kolm and his team have previously published research on the impact of publicly available news on financial markets; a methodology for assigning a value for clean-up costs, the opportunity costs associated with the canceled portion of an order; and the use of deep learning and neural networks on extracting alpha from granular order book data.
There are both practical and academic questions the futures data can answer, Kolm says. While equities trade in the 30-plus exchanges, dark pools, and alternative trading systems in the US, futures trading is more consolidated, so the data can give a more complete picture of a market and make research results more conclusive.
“The dataset tells us a lot about the activity in the order books of the exchange—how people trade, when they trade, and so forth,” he says. “Using the dataset, we can estimate the cost of trading in these markets.” In particular, they can look to measure price impact.
Price impact is commonly referred to as the correlation between an order and the price of the asset involved in the trade. Buying tends to push the price higher while selling can lower the price. His team is looking to build a price impact model for the futures market with the granular data they now have access to.
Additionally, Kolm says limit orders placed on the bid and ask can reflect interest by market participants to trade in a certain direction. Predictive signals could be derived from that information to predict where markets could go in the short term.
Kolm’s team will look to train the same or similar algorithms used in their equities research on the futures data. “There’s been very little academic research released on these types of topics, which is perhaps surprising. But part of that is the lack of availability of this kind of data to academics,” he says.
In contrasting the research around equities, Kolm says the order book in equities could end up looking like the order book in futures, but there is also potential for discovery of new behaviors and new forms of predictability.
Elliot Banks, chief product officer at BMLL, says that while the number of venues in futures is smaller, the scale can be larger. The largest equity symbol may have a few million updates daily, but the largest futures contract may have something an order of magnitude larger than that, he says. This can present a challenge in dealing with futures data as researchers can spend long periods of time doing data engineering before being able to apply data to models.
Additionally, there are nuances present in futures not seen in equities. “If It’s a futures contract, I am buying something that is going to expire at a future point in time, such as the price of wheat, the S&P 500 or oil,” Banks says. “I might want to take a view on the future price over a longer period of time and I therefore have to roll that contract and make sure that I manage going from one contract to the next.”
This is referred to as rolling a futures contract and BMLL can work to identify what the underlying futures data is before transferring it to a dataset for an end-user or researcher. “It comes down to understanding what’s in the dataset, how to make that consistent across venues, how to engineer something of that petabyte scale and then put it into a format that users can actually go into, so they don’t have to do the data engineering but run their analysis straight away,” Banks says.
Kolm says additional time can be needed to collect and organize data from scratch for researchers, but with BMLL that time is saved. “Twenty years ago, many quants spoke about daily data as high-frequency data. At that time a lot of academic research was leveraging weekly or monthly data,” Kolm says. “Today, we’re going and looking at datasets that are timestamped down to the nanosecond.”
Last year, BMLL said it was supplying order book data to Paris-based Ecole Polytechnique. The researchers were also conducting research on market microstructure, and looking to use the data in models they built to understand the interactions of price discovery, trading behavior, and trading venue structure in a high-frequency trading context.
Further reading
Only users who have a paid subscription or are part of a corporate subscription are able to print or copy content.
To access these options, along with all other subscription benefits, please contact info@waterstechnology.com or view our subscription options here: http://subscriptions.waterstechnology.com/subscribe
You are currently unable to print this content. Please contact info@waterstechnology.com to find out more.
You are currently unable to copy this content. Please contact info@waterstechnology.com to find out more.
Copyright Infopro Digital Limited. All rights reserved.
As outlined in our terms and conditions, https://www.infopro-digital.com/terms-and-conditions/subscriptions/ (point 2.4), printing is limited to a single copy.
If you would like to purchase additional rights please email info@waterstechnology.com
Copyright Infopro Digital Limited. All rights reserved.
You may share this content using our article tools. As outlined in our terms and conditions, https://www.infopro-digital.com/terms-and-conditions/subscriptions/ (clause 2.4), an Authorised User may only make one copy of the materials for their own personal use. You must also comply with the restrictions in clause 2.5.
If you would like to purchase additional rights please email info@waterstechnology.com
More on Data Management
New working group to create open framework for managing rising market data costs
Substantive Research is putting together a working group of market data-consuming firms with the aim of crafting quantitative metrics for market data cost avoidance.
Off-channel messaging (and regulators) still a massive headache for banks
Waters Wrap: Anthony wonders why US regulators are waging a war using fines, while European regulators have chosen a less draconian path.
Back to basics: Data management woes continue for the buy side
Data management platform Fencore helps investment managers resolve symptoms of not having a central data layer.
‘Feature, not a bug’: Bloomberg makes the case for Figi
Bloomberg created the Figi identifier, but ceded all its rights to the Object Management Group 10 years ago. Here, Bloomberg’s Richard Robinson and Steve Meizanis write to dispel what they believe to be misconceptions about Figi and the FDTA.
SS&C builds data mesh to unite acquired platforms
The vendor is using GenAI and APIs as part of the ongoing project.
Aussie asset managers struggle to meet ‘bank-like’ collateral, margin obligations
New margin and collateral requirements imposed by UMR and its regulator, Apra, are forcing buy-side firms to find tools to help.
Where have all the exchange platform providers gone?
The IMD Wrap: Running an exchange is a profitable business. The margins on market data sales alone can be staggering. And since every exchange needs a reliable and efficient exchange technology stack, Max asks why more vendors aren’t diving into this space.
Reading the bones: Citi, BNY, Morgan Stanley invest in AI, alt data, & private markets
Investment arms at large US banks are taken with emerging technologies such as generative AI, alternative and unstructured data, and private markets as they look to partner with, acquire, and invest in leading startups.