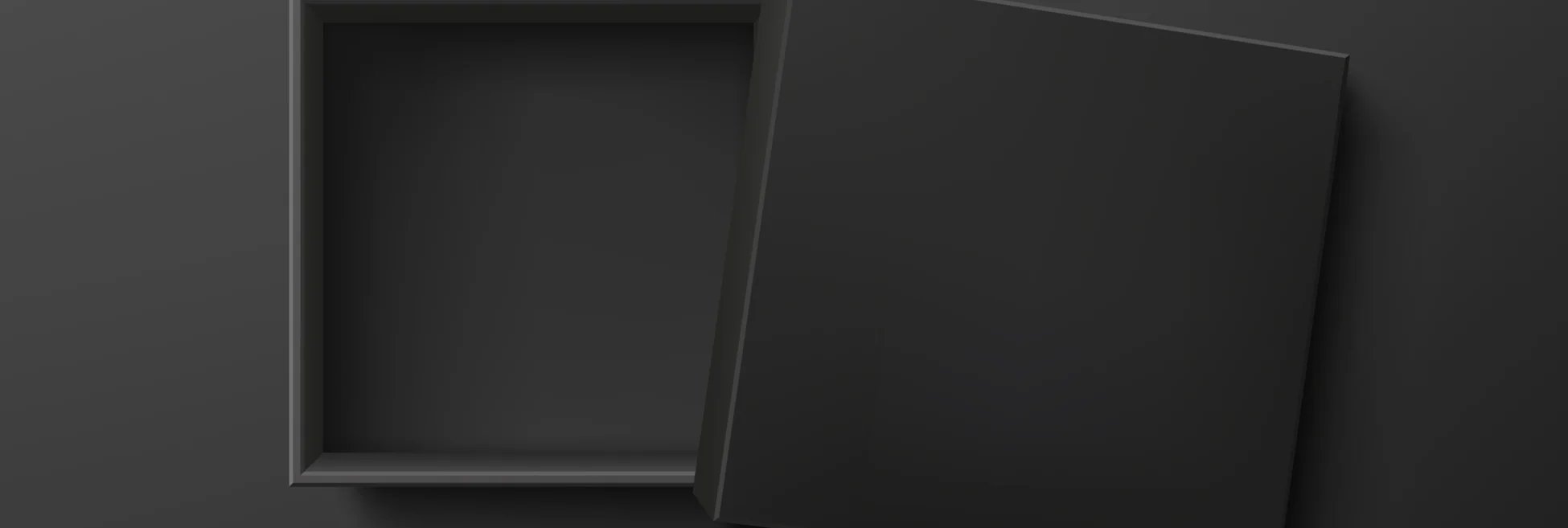
AI Explainability 'an Afterthought' at Banks, But Financial Theory Infusion Could Help
Eric Tham of the National University of Singapore said during the Innovation Exchange that explainability is an afterthought at banks when they develop their AI-driven models. Unsurprisingly, some bankers did not agree.
As financial firms increasingly turn to artificial intelligence for help with decision-making, and as these tools become increasingly sophisticated, ensuring that the users understand how the AI derives its outcome is becoming more difficult. And this idea of explainability is becoming top-of-mind for regulators.
Eric Tham, a senior lecturer at the National University of Singapore, contends, however, that it’s not explainability that is the greatest challenge for financial organizations using AI. He believes that there is a bigger problem: firms build platforms and use machine learning as a bolt-on tool, rather than ingraining machine learning into the system at the initial concept stage.
“We know the models in place are usually an afterthought, and [evaluated] largely on feature importance. Most [machine learning models] differ by how they’re obtained, the computation, the derivation, but it all goes down to the fact that they all highlight which feature is important. It still doesn’t quite explain why AI models work in finance,” said Tham, who was speaking at the inaugural WatersTechnology Innovation Exchange.
He said that what could help make AI more explainable is to “infuse” it with financial theory, and there are a few areas within financial theory that overlap with AI.
“If you recognize that AI is about discovering relationships, then we have to go into it a bit deeper,” he said. “What are these relationships in finance? AI does this in a data-driven manner; it allows you to find patterns in finance. But to understand it deeper, you have to understand financial theory.”
One example is in stochastic calculus, used in quantitative finance to calculate asset prices in the Black-Scholes model. Stochastic calculus—used to model systems that behave randomly—has been used since the early 1900s. In finance specifically, it has been applied in financial mathematics and economics since the 1970s to model the evolution in time of stock prices and bond interest rates.
The Girsanov theorem, which is part of stochastic calculus and is used in derivatives pricing, is similar to a kind of deep neural network known as a convolutional neural network (CNN), Tham said. They both use weighted sums to come up with an output.
“This weighted sum is very much similar to stochastic calculus in which it takes Girsanov theorem and takes a weighted probability distribution in order to derive asset prices. This is the key similarity between CNN and Girsanov theorem,” he said.
Contextual memory is another example. In recent behavioral research in finance, he said, prices are shown to work on contextual memory, meaning that the market reflects on events in the past.
With regard to the present Covid-19 situation, many investors and the general public compare it to previous recessions and the financial crisis. He said this brings to mind attention-based models—which are part of sequence-to-sequence models that takes a sequence of items and outputs another sequence of items—which is one of the latest advances in AI transformer models.
According to a tutorial on Google’s open-source machine-learning platform, TensorFlow, the core idea behind the transformer model is self-attention—the ability to attend to different positions of the input sequence to compute a representation of that sequence.
Tham said behavioral finance is similar to transformer models, which uses multi-headed attention to compute multiple attention weighted sums.
Tham, who has 15 years of experience working in risk management, quantitative analytics, and energy economics at various banks, fintech startups, and energy companies, noted that without infusing financial theory into AI models, it could lead to “another winter” for AI.
“To understand how AI works in finance, there’s a need to understand cross-disciplinary finance and how AI models work … Otherwise, AI will purely just be curve-fitting, and would just be trying to find relationships. That has been raised by many AI experts and the thought was that if it’s just curve-fitting, soon AI could enter another winter, unless [firms] infuse into AI models the understanding of financial theory, such as stochastic calculus and behavioral finance contextual memory. These are some of the fields in finance that could help explain how AI models work,” he said.
I Disagree, Good Sir
Kirill Petropavlov, director for AI innovation at Bank of Singapore, while speaking on a separate panel, did not agree with these assertions. He contended that financial services firms are under greater regulatory scrutiny than many other industries, much less the technology companies themselves.
In fact, because there is such a high bar for explainability in financial services, there is pressure on banks and asset managers to go slowly when building AI-driven tools, or to purchase software that is explainable.
“It’s not really right to say that we’re treating it as an afterthought. If you think about it, for the banks it’s actually much harder than for startups or fintechs, because we have accountability, and we’re regulated,” he said. “We’re not building black boxes, per se—we always know the factors. There’s quite often this misconception about it, that AI is something you just let run, [that you] let it train itself and then let it come up with a decision on its own.”
Petropavlov said that financial organizations cannot simply experiment with AI on customers and “hope for the best.”
“We have to do the really hard work to bring it to the point where we can say, ‘Okay, I know what it’s doing, I know why it is doing [that], and I know that it’s doing the right thing,’” he said.
Sumit Kumar, head of trade execution technology and lead architect for equities, Asia Pacific at Credit Suisse, agreed with Tham’s idea that sometimes banks do approach AI as an afterthought—but that’s the case for legacy tech.
He noted that there are many mature systems that underpin a bank’s tech stack that are not cloud native and were more likely to use robotic process automation rather than neural networks. It’s a different story when it’s a new project that is built from the ground up.
“In all honesty, that’s for the existing projects where we’re doing an enhancement; but when we start something from scratch, then the way it is approached is quite different,” Kumar said. “AI would be looked at as nothing more than glorified statistics. So effectively, the explainability part when you’re doing it from scratch is accounted for when you’re developing it. But then, the thing is that we have a huge amount of software exposure that’s running currently in production and you have to make it work together with that. That’s where the challenge comes [from].”
Further reading
Only users who have a paid subscription or are part of a corporate subscription are able to print or copy content.
To access these options, along with all other subscription benefits, please contact info@waterstechnology.com or view our subscription options here: http://subscriptions.waterstechnology.com/subscribe
You are currently unable to print this content. Please contact info@waterstechnology.com to find out more.
You are currently unable to copy this content. Please contact info@waterstechnology.com to find out more.
Copyright Infopro Digital Limited. All rights reserved.
As outlined in our terms and conditions, https://www.infopro-digital.com/terms-and-conditions/subscriptions/ (point 2.4), printing is limited to a single copy.
If you would like to purchase additional rights please email info@waterstechnology.com
Copyright Infopro Digital Limited. All rights reserved.
You may share this content using our article tools. As outlined in our terms and conditions, https://www.infopro-digital.com/terms-and-conditions/subscriptions/ (clause 2.4), an Authorised User may only make one copy of the materials for their own personal use. You must also comply with the restrictions in clause 2.5.
If you would like to purchase additional rights please email info@waterstechnology.com
More on Emerging Technologies
This Week: Startup Skyfire launches payment network for AI agents; State Street; SteelEye and more
A summary of the latest financial technology news.
Waters Wavelength Podcast: Standard Chartered’s Brian O’Neill
Brian O’Neill from Standard Chartered joins the podcast to discuss cloud strategy, costs, and resiliency.
SS&C builds data mesh to unite acquired platforms
The vendor is using GenAI and APIs as part of the ongoing project.
Chevron’s absence leaves questions for elusive AI regulation in US
The US Supreme Court’s decision to overturn the Chevron deference presents unique considerations for potential AI rules.
Reading the bones: Citi, BNY, Morgan Stanley invest in AI, alt data, & private markets
Investment arms at large US banks are taken with emerging technologies such as generative AI, alternative and unstructured data, and private markets as they look to partner with, acquire, and invest in leading startups.
Startup helps buy-side firms retain ‘control’ over analytics
ExeQution Analytics provides a structured and flexible analytics framework based on the q programming language that can be integrated with kdb+ platforms.
The IMD Wrap: With Bloomberg’s headset app, you’ll never look at data the same way again
Max recently wrote about new developments being added to Bloomberg Pro for Vision. Today he gives a more personal perspective on the new technology.
LSEG unveils Workspace Teams, other products of Microsoft deal
The exchange revealed new developments in the ongoing Workspace/Teams collaboration as it works with Big Tech to improve trader workflows.