AI Machines Tackle Data Overload for Human Overlords
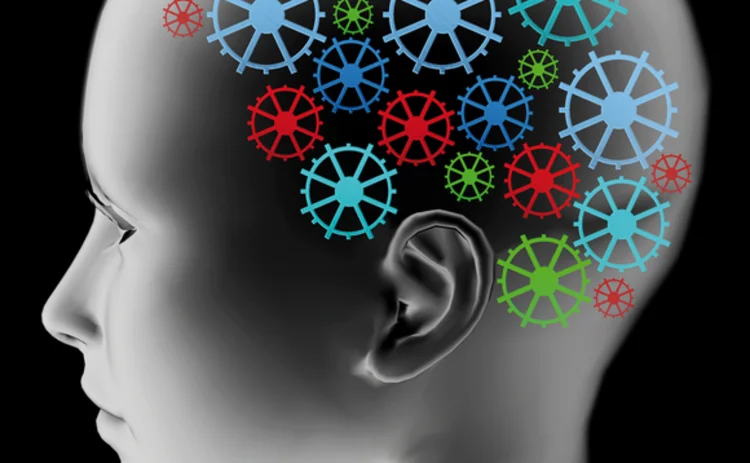
Not only is market data disseminated faster today than ever, but there is also more data than ever before, leading to more automation of the collection, storage and analysis of data—not just to support algorithmic trading, but also qualitative analysis by individuals. At a time when data volumes have grown beyond what human traders and analysts can keep track of manually, the industry is adopting new artificial intelligence tools to automate the extraction of insight from large datasets, including formats intended for human consumption—such as news, commentary and research.
Over recent years, the industry has spent much money and effort on generating, collecting and storing data, and on accessing the stored data, but is now placing greater focus on the “last mile” of Big Data—actually getting value out of data by effectively communicating insight, says Stuart Frankel, chief executive of artificial intelligence-based content generation technology provider Narrative Science.
“You can do all this underlying work that collects and gives you access to the data, but if you can’t get insight out of that data and ultimately communicate it to customers, partners or colleagues, that data is pretty useless. Companies—even three years ago [when Narrative Science was founded]—are suffering from data fatigue, and they’re not using most of the data they’re actually capturing,” Frankel says.
As such, to help financial advisors inform their clients of market activity that may impact their investments or trigger new ideas, Encinitas, Calif.-based idea generation and market scanning technology provider Trade Ideas is building a content generation tool—scheduled for release in the second half of this year—to turn its data tables into text commentary or bullet points that can be used in advisor research notes, to help maintain their relationship with customers through regularly-delivered, targeted content that would otherwise be time-consuming to produce on a frequent basis, says David Aferiat, managing partner of Trade Ideas.
Rather than simply passing on the data tables to clients, putting the data into a narrative context enables advisors to point out specific angles that are relevant to clients, instead of leaving it to clients to interpret the data, Aferiat says.
“Companies are starting to collect their own data to give them an advantage in the market, and we’re helping them turn that proprietary data into something more useful than just the raw data itself.” —Stuart Frankel, CEO, Narrative Science
Open to Interpretation
Similarly, data visualization tools only represent data in different ways, leaving the onus of extracting meaning and value on the audience, says Ron Shevlin, senior analyst at Aite Group, whereas narrative “allows the outputs to be more direct, in terms of saying, [for example], ‘here are the three most important things you need to know’.” But a combination of visualizations and text could prove more compelling than either alone, Shevlin says. While visualizations still require interpretation, people now have shorter attention spans and less appetite for reading lengthy portions of text, so combining graphics with text could be an effective way to communicate information, he adds.
In fact, Narrative Science sees opportunities to enhance charts and graphs by processing the same underlying data in its artificial intelligence engine to generate text to accompany charts, Frankel says. “That would allow someone to look at the information visually, but also—in a second or two—read a snippet of text that immediately tells them what they’re seeing and what they’re looking for in the graph,” he adds.
In addition to content delivered externally, artificial intelligence platforms can also help a firm’s own traders and analysts uncover insight they may otherwise miss, to support their trading decisions.
Narrative Science is already working to create a proprietary news feed and provide stock market analysis for a bulge-bracket firm’s institutional trading desk, and is also being enlisted by trading firms to turn data collected from surveys of a company’s customers into actionable investment analysis and recommendations for that company’s stock, using the vendor’s Quill platform, Frankel says. “Traders want to get more information, particularly proprietary information that others don’t have…. Companies are starting to collect their own data to give them an advantage in the market, and we’re helping them turn that proprietary data into something more useful than just the raw data itself,” he adds.
Likewise, investment analysts who typically use sophisticated financial models to make assessments of companies’ future earnings, can leverage IBM’s Watson supercomputer—which large investment banks, including Citi, are now exploring for potential use cases—to analyze large amounts of unstructured data to augment their structured financial analysis, says Likhit Wagle, global industry leader for banking and financial markets at IBM Global Business Services. This could include using sentiment analysis to determine the probability of the result predicted by the financial models, or identifying a new but obscure drug regulation change in China that could restrict a US pharmaceutical company’s market potential, which an analyst might miss, he adds.
“It won’t replace the financial analysis being done, but will substantially improve the quality of insights being drawn from that financial analysis by providing a view of the key messages coming out of the unstructured information, and the insights that can be drawn from the unstructured information,” Wagle says. “You may well find that the unstructured data is coming up with some conclusions or insights that contradict what the financial analysis shows—and that gives the analyst the opportunity to explore in more detail why that contradiction exists.”
An analyst without this insight would typically rely on gut feeling, prior experience, and to some extent, luck that they will come across a piece of information that may be particularly relevant to their analysis, all backed up by devoting significant amounts of time to researching large volumes of data, Wagle says, whereas Watson uses a cognitive learning capability to improve its outputs—for example, by focusing its responses or by broadening the scope of information it considers—based on the questions asked, how questions are asked, and on subsequent follow-up questions.
These artificial intelligence platforms are by no means intended to be a turnkey, black-box solution, Shevlin notes. Human expertise is still ultimately required to define what constitutes a “good” report—as opposed to a “bad” report—to set up a desirable output, he says. And while artificial intelligence platforms aim to automate and replicate an analyst’s ability to research and create useful insight and reports, they are still ultimately driven and shaped by qualitative human decisions and are intended to support, rather than replace, human analysts, he adds.
Only users who have a paid subscription or are part of a corporate subscription are able to print or copy content.
To access these options, along with all other subscription benefits, please contact info@waterstechnology.com or view our subscription options here: http://subscriptions.waterstechnology.com/subscribe
You are currently unable to print this content. Please contact info@waterstechnology.com to find out more.
You are currently unable to copy this content. Please contact info@waterstechnology.com to find out more.
Copyright Infopro Digital Limited. All rights reserved.
As outlined in our terms and conditions, https://www.infopro-digital.com/terms-and-conditions/subscriptions/ (point 2.4), printing is limited to a single copy.
If you would like to purchase additional rights please email info@waterstechnology.com
Copyright Infopro Digital Limited. All rights reserved.
You may share this content using our article tools. As outlined in our terms and conditions, https://www.infopro-digital.com/terms-and-conditions/subscriptions/ (clause 2.4), an Authorised User may only make one copy of the materials for their own personal use. You must also comply with the restrictions in clause 2.5.
If you would like to purchase additional rights please email info@waterstechnology.com
More on Emerging Technologies
This Week: Startup Skyfire launches payment network for AI agents; State Street; SteelEye and more
A summary of the latest financial technology news.
Waters Wavelength Podcast: Standard Chartered’s Brian O’Neill
Brian O’Neill from Standard Chartered joins the podcast to discuss cloud strategy, costs, and resiliency.
SS&C builds data mesh to unite acquired platforms
The vendor is using GenAI and APIs as part of the ongoing project.
Chevron’s absence leaves questions for elusive AI regulation in US
The US Supreme Court’s decision to overturn the Chevron deference presents unique considerations for potential AI rules.
Reading the bones: Citi, BNY, Morgan Stanley invest in AI, alt data, & private markets
Investment arms at large US banks are taken with emerging technologies such as generative AI, alternative and unstructured data, and private markets as they look to partner with, acquire, and invest in leading startups.
Startup helps buy-side firms retain ‘control’ over analytics
ExeQution Analytics provides a structured and flexible analytics framework based on the q programming language that can be integrated with kdb+ platforms.
The IMD Wrap: With Bloomberg’s headset app, you’ll never look at data the same way again
Max recently wrote about new developments being added to Bloomberg Pro for Vision. Today he gives a more personal perspective on the new technology.
LSEG unveils Workspace Teams, other products of Microsoft deal
The exchange revealed new developments in the ongoing Workspace/Teams collaboration as it works with Big Tech to improve trader workflows.