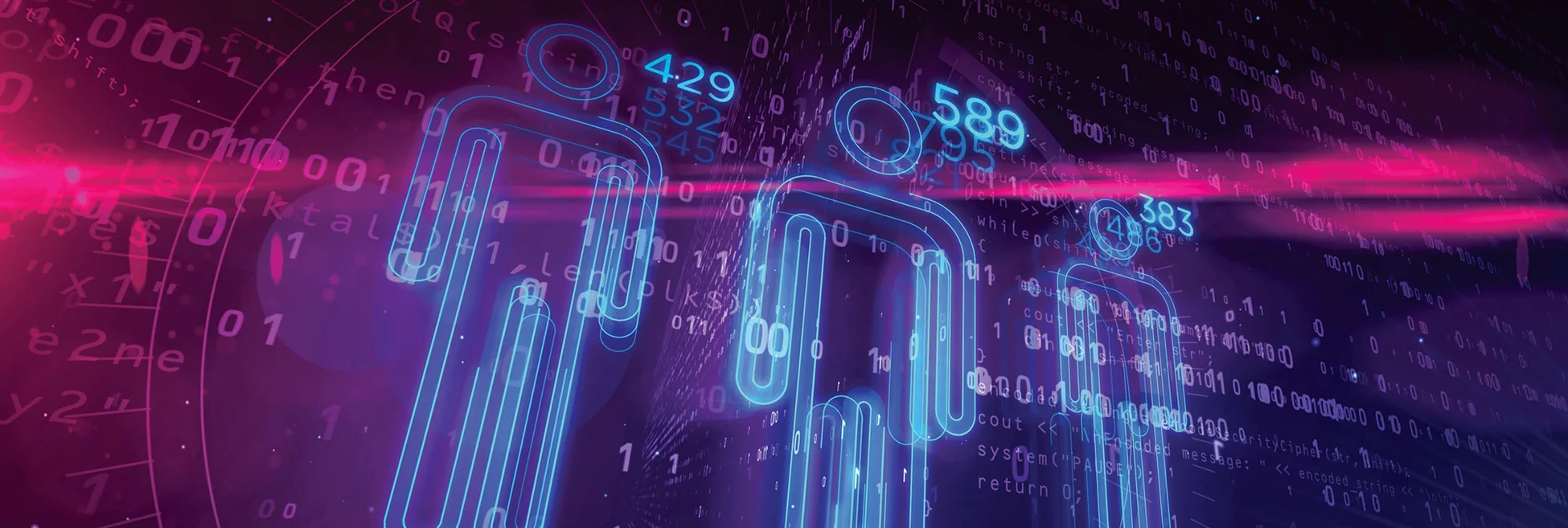
Alt Data Aims to Shake up Credit Scoring Business
Young firms, using machine-learning methods to scrape consumer info, challenge established agency model.
Need to know
- Today’s credit scoring industry—which is based on a person’s history of loan and mortgage payments—is too restrictive, some argue.
- New agencies are trying to widen the range of financial data that’s used to assess loan applicants. They hope to extend credit scoring to the unbanked, gig economy workers and young people with little credit history.
- These challengers use machine learning techniques to plow through financial transaction data, or to scrutinize online questionnaires.
- Machine learning is controversial in this area, as it could give rise to bias and discrimination in lending decisions.
- Nevertheless, large credit card companies such as Capital One and established agencies like Experian are showing interest in these new methods.
Custom dictates that lenders rate an individual’s ability to repay a loan by checking their credit history. It’s a simple concept that has been the bedrock of consumer lending since the Fair Isaac Corporation—now Fico—designed the first credit scoring algorithms 50 years ago.
Now, a new generation of upstarts are trying to topple this convention. Two challengers, Credit Kudos and Aire, are developing ways of collecting and analyzing a wider range of data on consumers. They claim their methods can help banks assess the creditworthiness of millions of would-be borrowers who slip through the credit scoring net.
“Traditional credit models are broadly based on the same facets—that’s the Fico-style model, where you’re looking at a combination of past borrowing, repayments, debt obligations, searching behavior and maybe some demographic information,” says Freddy Kelly, chief executive officer of Credit Kudos.
“That’s not a granular view of an individual’s financial standing; it can be out of date, it can be unfairly reflective, or it can be incomplete. If I’ve never borrowed before, I don’t have a credit file to speak of,” he adds.
Their methods may be novel, but Credit Kudos and Aire are starting to garner attention from the established players they seek to differentiate themselves from. Aire has attracted investment from the likes of retail credit-checking giant Experian, while Credit Kudos has worked with Capital One’s startup incubator, Growth Labs.
Aire is working with a major UK credit card provider, helping to boost acceptances for marginal declines; on affordability assessments with a short-term lender; and with a “leading” UK bank, where Aire is working to up the quality of customer data.
Credit Kudos says it’s working with a number of credit card companies and lenders. But the partnerships aren’t in a public stage yet, and Kelly is unwilling to share details.
The firms’ pitch to redefine credit scoring comes as bank risk managers scramble to reassess loan portfolios in light of Covid-19 and its paralyzing economic effects. Lenders are also having to cope with tough new accounting measures that force them to hold bigger provisions against bad loans.
Under the typical model of credit scoring, agencies crunch data on a consumer’s previous loans and credit card payments to rate their likelihood of repaying a future loan. Proponents say this method gives a reliable gauge of creditworthiness. Critics say it locks out consumers who haven’t built up a credit history. Data from Experian shows there are 5.8 million individuals with thin-to-nonexistent credit files in the UK alone.
To reach these unrepresented customers, Credit Kudos looks at a wide range of financial transaction data. The firm scours an individual’s full financial history—incomings, outgoings, overdraft usage—to try and gain a detailed understanding of their spending behavior and level of financial stability.
Aire uses a different approach. It gathers information from self-reported online questionnaires, and uses algorithms to compare and validate the data.
Both firms lean heavily on machine-learning techniques which, they say, improve the accuracy and fairness of their credit scores. But the method has drawbacks, experts say. Algos can exhibit bias, leading to discrimination in lending decisions. The simpler method of incumbent Fico is considered to be less prone to these risks.
Fico says its credit scores—which are used by the majority of lenders—are just one of a range of factors that banks should take into account when deciding who to lend money to.
“It’s true that if you don’t have credit, you will not have a credit report. But Fico scores were never designed to be used solely in underwriting decisions—lenders look at other information too,” says Sally Taylor, vice-president of scores, at Fico.
However, changes in working habits and the disruption of Covid have convinced some experts that it’s time to rethink the standard methods of credit scoring.
“Around the world, all of the credit scoring models have fallen down—they’re unable to deal with the new uncertainties that Covid has brought,” says Cris Conde, former chief executive officer of SunGard. “So we’re seeing any number of new approaches being tried, mostly with machine learning and using alternative datasets.”
Access (nearly) all areas
UK-based Credit Kudos is, in regulatory jargon, an account information services provider. This means the firm has access to Open Banking transaction data. Open Banking is a UK government initiative that requires banks to share customer data with third parties, as long as the customer has given permission.
With vast troves of data at its disposal, Credit Kudos is able to build a picture of customers’ financial activity. This forms the basis of its forecasts.
“The accuracy and quality of bank transaction data is almost second to none in terms of the insights it provides you,” says Kelly from Credit Kudos. “It’s a source of truth, with predictive power based on years of actual behavior.”
To create its customer forecasts, Credit Kudos compares the transaction data of a given applicant to its existing universe of customer profiles. If the new applicant’s activity looks similar to the spending behavior of the ‘good’ population on the database—individuals with a particular income-to-expenditure ratio, who show a propensity to accrue, and who exhibit promising balance trajectories over time—their score will likely be strong. And, Kelly adds, the continuous nature of Open Banking transaction statistics produces an up-to-the-minute portrait of an individual, against the more intermittent info used by Fico.
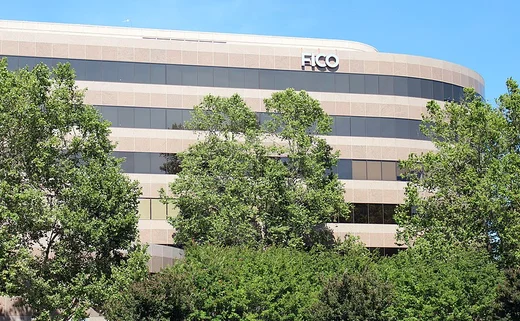
“A traditional credit file might change on a monthly basis, because you’re maybe searching for a loan or you’ve made a mortgage repayment. It’s a very broad-strokes measure; it’ll show you nothing unless you’re not repaying, and then it’ll show you a negative signal,” says Kelly. “It doesn’t give you an insight into the behavior of the customer. Whereas if you’re looking at bank transaction data, people are transacting every day, multiple times – you look at payments into the account, income fluctuations, that sort of thing.”
Credit agency Aire uses a different method to produce its scores. It evaluates loan applicants via an online Q&A which it dubs an interactive virtual interview, or IVI. Applicants—who don’t need a bank account to apply—complete an adaptive, multiple choice internet survey which poses a variety of questions on topics ranging from their employment history to income to qualifications.
By applying artificial-intelligence techniques to this information, the company forms a picture of the individual’s creditworthiness. The advantage of this approach, Aire says, is that it can evaluate wannabe borrowers who escape the usual credit scoring radar: young people, recent immigrants, and the unbanked.
As HP Bunaes, an expert in artificial intelligence and the founder of AI Powered Banking, says: “Artificial intelligence has the potential to make credit available to people who would be overlooked with the use of traditional credit scoring.”
So how does Aire identify survey respondents who have given false information—for example, by inflating their earnings? It compares their responses to a database of around half a million jobs and associated salaries, and assigns a probability to their stated income.
“From our dataset, we have built up statistical distributions around what we would expect jobs to pay given the various attributes people provide us. And on that basis, we can say how likely it is that somebody in that situation would be earning that amount of money,” says Thomas Turner, Aire’s data science manager.
Once anomalies have been flagged, the online questionnaire adapts on the fly. If a particular answer or set of answers appears dubious when compared to the dataset, more questions will be asked on points that seem problematic or inconsistent.
If Aire believes that a customer is reporting their income inaccurately, they aren’t prevented from applying for credit; rather, a number of measures are taken to reach a realistic conclusion.
“If we’re concerned that somebody may have inflated their income, we can, for the purposes of modelling their affordability, use our maximum likelihood estimation of what we think their income is likely to be,” Turner says. “Or we can just flag it to the lender. There is some validation that happens in the app: if people’s declared numbers fall outside of a fairly wide range, they would be asked to correct. But in terms of what the model does itself downstream, that isn’t communicated back to the applicant.”
Open to scrutiny
Communication with customers is a touchy subject in credit lending circles. As banks make more use of algos to make lending decisions, they face a growing risk of unintended bias and discrimination against social groups. Authorities in the US are introducing laws to punish lenders that allow these practices.
To guard against bias, regulators are forcing lenders to justify the decisions made by artificial-intelligence programs. EU rules also oblige lenders to explain to customers why they may have been turned down for a loan, while regulators apply similar expectations in the US, says a senior model risk expert at one of the country’s largest lenders.
He points out that, every time it accepts or declines a consumer for a loan, the bank has to produce a decision code, explaining why, which is then liable to be scrutinized by its supervisor in case the decision was subject to implicit or explicit bias.
“We’re closely tracking the potential of methods like this, but in terms of regulatory expectations, we somewhat have to stick to the tried and true,” he says.
In the case of Aire, which is based in the UK, Turner says customers are able to contact the firm if they want the process by which their score is calculated to be explained. And, mindful that its automated processes are likely to draw regulatory scrutiny, Aire has opened an office in Washington, DC to make it easier for the firm to familiarize federal regulators with its activities.
Looking under the hood of Aire’s credit engine, Turner says it uses simple predictive models like linear and logistic regression, wherever possible. More sophisticated machine-learning techniques are used to explore “more complex, nonlinear” relationships in the data, such as Gaussian mixture models, decision trees, random forests and gradient boosted trees. This mixture of approaches, he says, enables the firm to use different models for particular sub-populations. Deep learning is not currently used.
The best risk-adjusted return tends to be in the slightly higher-risk segments, and the use of AI and alternative data can be a really good way to identify the highest potential return
HP Bunaes, AI Powered Banking
About two miles east, in Credit Kudos’s London offices, supervised and unsupervised machine learning is used to anatomize large quantities of raw bank data. Differences in how transactions are labelled and categorized between institutions can result in “unclean” data, CEO Kelly says, and machine learning brings the information into a functional state.
An unsupervised learner can complete this task, Kelly says, with an “overlaid” rules-based approach. Natural language processing helps build up a “lexicon” for the firm to use—a “universe of proper nouns and labels that might be assigned to a bank transaction.”
Machine learning also comes in handy in cases where an applicant’s financial activity is not neat and regular—a gig economy worker or self-employed person, for example, who may not have the same sort of reliable in-out monthly payments as a salaried employee.
“You can find patterns over time, and build simple logistic regressions,” Kelly says. “And then there’s a variety of deep learning techniques we look at. Once we’ve got this consolidated, standardized and normalized view of a customer’s bank account, we build that into training steps and build a prediction.”
The ability of these methods to fill in the data gaps of an atypical workforce is an area where Cris Conde sees a big advantage. The incumbent credit scoring models “don’t deal well with the unbanked, they don’t deal well with gig workers,” he says.
“I’m a gig worker, and it’s pathetic what the models say for me,” he adds.
Fine-tuning
A potential drawback of using complex algorithms is that the machinery needs constant fine-tuning. Bunaes warns that firms operating in the space must evaluate and update their models to maintain accuracy.
“The model may work well in certain cases, and badly in others,” he says. “You have to monitor these models in-use, and retrain them often. Processes for calibration and improvement like A/B testing and champion/challenger should be used to improve them as the signal changes, as the population changes, as economic conditions change, and as you get more data that may be from parts of the population that you didn’t initialize your model on.”
Conde agrees that poor software architecture and lack of diligence could lead to a “drift” towards machine discrimination. The Fico-style approach, he says, has an advantage here: lenders see it as safe, and uncomplicated by the ins and outs of AI explainability and audit challenges and the need to describe gradient boost algorithms to board members. The orthodox method is not free of its own bias, Conde adds: there are overt biases towards certain types of customer in the traditional credit history approach used by big agencies, he argues, but the model is accepted by regulators and considered best practice.
But the prize offered by the challengers may sway banks. The credit scoring methods of the likes of Aire and Credit Kudos would allow lenders to evaluate the creditworthiness of a large and potentially lucrative segment of the population. Credit card providers are able to rake in higher fees from people with worse credit scores—and alternative agencies may be the gateway to this cohort.
“Banks tend to be risk-averse, and focus very much on the lowest-risk segments to minimize losses. But the best risk-adjusted return tends to be in the slightly higher-risk segments, and the use of AI and alternative data can be a really good way to identify the highest potential return,” says Bunaes.
Conde agrees that lenders are missing out on the much larger returns available in riskier population segments.
When evaluating firms such as Aire and Credit Kudos, lenders should be alert to the ways that their methods perform in periods of market stress, Conde says. But, he emphasizes, they will soon find that they have no alternative.
“The traditional approaches are not going to give acceptable answers; whether we like it or not, we have to jump into the pool,” he says. “There is simply no option—the loss ratios predicted by the traditional approaches are completely wrong. So what’s the alternative—don’t lend at all?”
Additional reporting by Tom Osborn
Only users who have a paid subscription or are part of a corporate subscription are able to print or copy content.
To access these options, along with all other subscription benefits, please contact info@waterstechnology.com or view our subscription options here: http://subscriptions.waterstechnology.com/subscribe
You are currently unable to print this content. Please contact info@waterstechnology.com to find out more.
You are currently unable to copy this content. Please contact info@waterstechnology.com to find out more.
Copyright Infopro Digital Limited. All rights reserved.
As outlined in our terms and conditions, https://www.infopro-digital.com/terms-and-conditions/subscriptions/ (point 2.4), printing is limited to a single copy.
If you would like to purchase additional rights please email info@waterstechnology.com
Copyright Infopro Digital Limited. All rights reserved.
You may share this content using our article tools. As outlined in our terms and conditions, https://www.infopro-digital.com/terms-and-conditions/subscriptions/ (clause 2.4), an Authorised User may only make one copy of the materials for their own personal use. You must also comply with the restrictions in clause 2.5.
If you would like to purchase additional rights please email info@waterstechnology.com
More on Data Management
New working group to create open framework for managing rising market data costs
Substantive Research is putting together a working group of market data-consuming firms with the aim of crafting quantitative metrics for market data cost avoidance.
Off-channel messaging (and regulators) still a massive headache for banks
Waters Wrap: Anthony wonders why US regulators are waging a war using fines, while European regulators have chosen a less draconian path.
Back to basics: Data management woes continue for the buy side
Data management platform Fencore helps investment managers resolve symptoms of not having a central data layer.
‘Feature, not a bug’: Bloomberg makes the case for Figi
Bloomberg created the Figi identifier, but ceded all its rights to the Object Management Group 10 years ago. Here, Bloomberg’s Richard Robinson and Steve Meizanis write to dispel what they believe to be misconceptions about Figi and the FDTA.
SS&C builds data mesh to unite acquired platforms
The vendor is using GenAI and APIs as part of the ongoing project.
Aussie asset managers struggle to meet ‘bank-like’ collateral, margin obligations
New margin and collateral requirements imposed by UMR and its regulator, Apra, are forcing buy-side firms to find tools to help.
Where have all the exchange platform providers gone?
The IMD Wrap: Running an exchange is a profitable business. The margins on market data sales alone can be staggering. And since every exchange needs a reliable and efficient exchange technology stack, Max asks why more vendors aren’t diving into this space.
Reading the bones: Citi, BNY, Morgan Stanley invest in AI, alt data, & private markets
Investment arms at large US banks are taken with emerging technologies such as generative AI, alternative and unstructured data, and private markets as they look to partner with, acquire, and invest in leading startups.