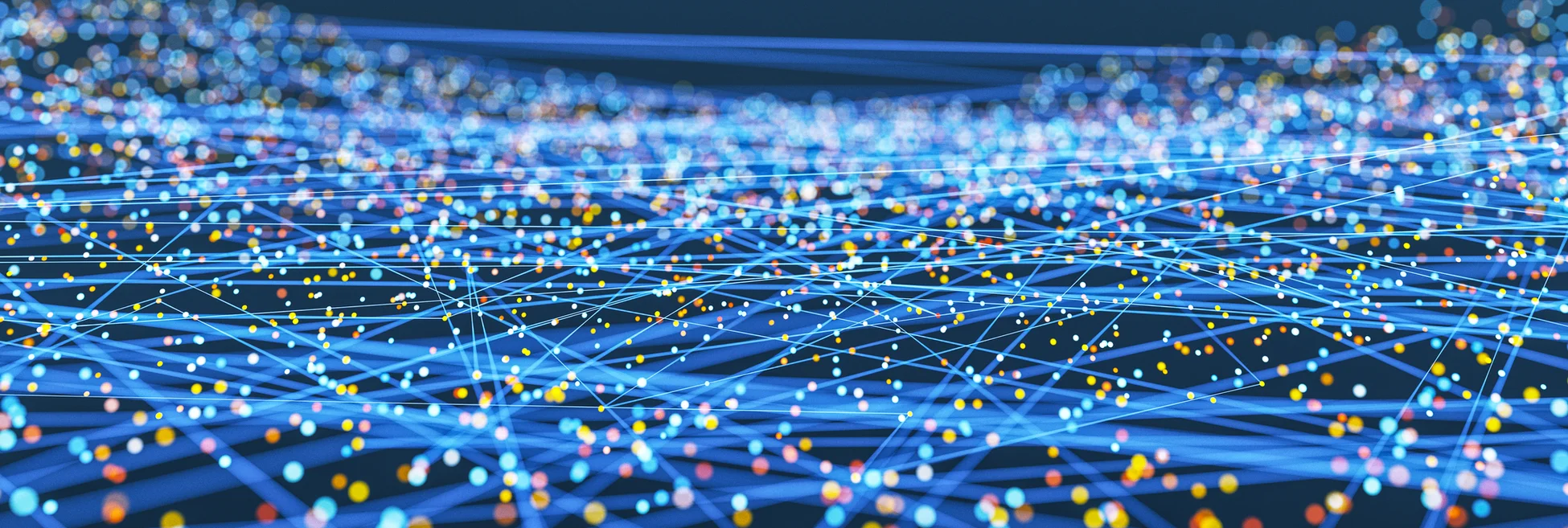
Alt-Data Difficulties Challenge Largest Asset Managers
A new study finds that while large asset managers are investing in big data analytics and alternative data, it’s a fraught process.
For many, the next great battleground in asset management will revolve around advanced analytics and alternative data. Asset managers are gearing up for the challenge, but it’s by no means a slam dunk, yet.
Earlier this year, UBS Asset Management formed the Quantitative Evidence and Data Science team (QED), which was spun out of the organization’s quantitative investment research team to help the manager better incorporate alternative datasets and perform advanced analytics using new technologies.
Suvrat Bansal, head of innovation and chief data officer at the asset manager, tells WatersTechnology that, through creating a dedicated unit that has a laser focus on the alternative data and machine learning spaces, and by combining that with its fundamental data, the firm can better explain the alpha that’s being created by a signal. But it’s not an easy process and it’s one of iteration. Part of the trick, he says, is building an engagement model to avoid sprawl and wasted energy.
“Without that kind of engagement and interpretability, you’re going to constantly go through a staggered failure-and-success [process] and that takes time,” says Bansal, “Obviously, there are more capabilities constantly evolving in terms of more data and more platforms, but what it comes down to is how you make it real for our portfolio managers and our researchers, and their ability to explain it to the clients, which is the hardest part and has been the hard part on the quant side. Can you take that and apply it equally on the alternative-data and machine-learning side—that’s the winning combo.”
By forming QED, UBS AM is farther along than most. Indeed, there appears to be no homogenous state as such, when it comes to the buy side’s exploration of machine learning and alternative data.
On November 7, data and analytics advisory firm Element22 released a report that benchmarks where 20 asset managers are in terms of incorporating alternative data and delivering advanced analytics. Those included in the study, which was sponsored by UBS and includes its asset-management arm, are hardly small fry—combined, they have almost $15 trillion under management, representing 20 percent of total global AUM. The inaugural report breaks the institutions into one of four buckets: two are just starting work in this sector, five are in the middle of their projects and two said they are breaking new ground, while the remainder are just starting their projects.
Predrag Dizdarevic, founding partner of Element22, describes advanced analytics as being able to deploy machine-learning algorithms for alpha generation, classification of client acquisition and retention; for business operations, using natural language processing (NLP) to feed into the machine learning platforms; and smart robotic process automation for managing the digital workforce, combining machine learning bots with automation bots; and to assist humans to get to the feedback mechanism of the process.
It tends to be a four-year process to get from the starting gun to breaking new ground. Dizdarevic says that even firms in the middle are simply trying new things, whether that be through proofs-of-concept or a production environment for a smaller process.
“Because of that I’d say there are a lot of misses compared to the hits; however, it’s not like they build a whole infrastructure that really doesn’t work,” he says. “I see more of the efforts fail and some of them succeed, but at some point, those [projects] that succeed open the eyes of everybody to the potential and then the major investment comes.”

Those nearing the end of the journey, he says, have a number of elements in common. They have embraced the cloud and big data analytics tools; have the ability to spin up sandboxes; can create specialized environments to test datasets and models; have the ability to use native services within the main cloud providers; and have robust, specialized teams of data scientists and analysts. Those who are most aggressively investing in pursuing advanced analytics and alternative data strategies are investing between two-to-three percent of annual revenues, according to the report.
Lessons Learned
The alternative-data market is vast and complex, as many on both the buy side and sell side are learning. Projects often sprawl. Costs climb. Interest is lost. Frustration sets in. Projects are dumped. Even still, there is a thirst for this wellspring of information—according to the survey, asset managers in the middle of their journey plan to increase their investment in alternative data by 240 percent over the next three years.
That number, though, set off alarm bells for UBS AM’s Bansal, who says that he hopes people are not going back to the same old philosophy of throwing a whole bunch of data at a wall to see what sticks. Rather, he says, firms need to be targeted and also they need to be constantly vigilant and willing to adjust, in order to succeed.
“What we do is, if you look at our trial data versus what we institutionalize, the ratio is 10 to 1—so we are trying 10 things in a very nimble way, largely through our research team, we go through weekly awarding processes…[and] unless there’s a conviction of that signal being useful on a regular basis, we don’t proceed with the institutionalization of that.”
Yet there are certain lessons that can only be learned through experience—which requires firms to be nimble and accepting of change, even if it doesn’t deliver what was initially anticipated. As an example, when QED started to look at filing footnotes by running NLP techniques over these statements, it first used that only for valuation. But, as it gained experienced, it realized that using NLP on these statements is very effective to capture information pertaining to sustainability and environmental, social and governance ratings for individual companies.
“So you start to look at the dataset with one mindset, but then if it works, as you start to share it, [then you adjust] and then maybe the second purpose becomes more institutional and the first purpose dies,” Bansal says.
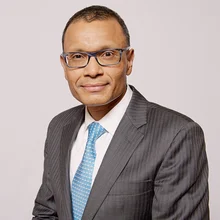
Finally, as it pertains to both advanced analytics and alternative data, it’s important to be self-critical and willing to move past failure. So, for example, in the past, everyone jumped into the idea of data lakes, but then 80 percent of the lake wasn’t being used; there was a solid plan for execution. Keeping the engagement model at the forefront is of prime importance.
“You have to make sure that you are constantly critiquing yourself in terms of the business outcomes you’re driving towards,” he says. “I think sometimes it becomes very easy to get attached to the next best technology and tooling and innovation idea—and there should be a healthy appetite for that but at a very low-cost basis with enough flexibility. …We have more demand than what we take on. There’s a flood of, ‘I need this. I need that. I want a sandbox.’ I think we are very disciplined around continuing with the path we have taken—constantly expand the funnel but with the same framework we have developed in the engagement model.”
Further reading
Only users who have a paid subscription or are part of a corporate subscription are able to print or copy content.
To access these options, along with all other subscription benefits, please contact info@waterstechnology.com or view our subscription options here: http://subscriptions.waterstechnology.com/subscribe
You are currently unable to print this content. Please contact info@waterstechnology.com to find out more.
You are currently unable to copy this content. Please contact info@waterstechnology.com to find out more.
Copyright Infopro Digital Limited. All rights reserved.
As outlined in our terms and conditions, https://www.infopro-digital.com/terms-and-conditions/subscriptions/ (point 2.4), printing is limited to a single copy.
If you would like to purchase additional rights please email info@waterstechnology.com
Copyright Infopro Digital Limited. All rights reserved.
You may share this content using our article tools. As outlined in our terms and conditions, https://www.infopro-digital.com/terms-and-conditions/subscriptions/ (clause 2.4), an Authorised User may only make one copy of the materials for their own personal use. You must also comply with the restrictions in clause 2.5.
If you would like to purchase additional rights please email info@waterstechnology.com
More on Emerging Technologies
This Week: Startup Skyfire launches payment network for AI agents; State Street; SteelEye and more
A summary of the latest financial technology news.
Waters Wavelength Podcast: Standard Chartered’s Brian O’Neill
Brian O’Neill from Standard Chartered joins the podcast to discuss cloud strategy, costs, and resiliency.
SS&C builds data mesh to unite acquired platforms
The vendor is using GenAI and APIs as part of the ongoing project.
Chevron’s absence leaves questions for elusive AI regulation in US
The US Supreme Court’s decision to overturn the Chevron deference presents unique considerations for potential AI rules.
Reading the bones: Citi, BNY, Morgan Stanley invest in AI, alt data, & private markets
Investment arms at large US banks are taken with emerging technologies such as generative AI, alternative and unstructured data, and private markets as they look to partner with, acquire, and invest in leading startups.
Startup helps buy-side firms retain ‘control’ over analytics
ExeQution Analytics provides a structured and flexible analytics framework based on the q programming language that can be integrated with kdb+ platforms.
The IMD Wrap: With Bloomberg’s headset app, you’ll never look at data the same way again
Max recently wrote about new developments being added to Bloomberg Pro for Vision. Today he gives a more personal perspective on the new technology.
LSEG unveils Workspace Teams, other products of Microsoft deal
The exchange revealed new developments in the ongoing Workspace/Teams collaboration as it works with Big Tech to improve trader workflows.