AML models face explainability challenges
Data gaps and potential biases must be accounted for in approaches to tackling money laundering.
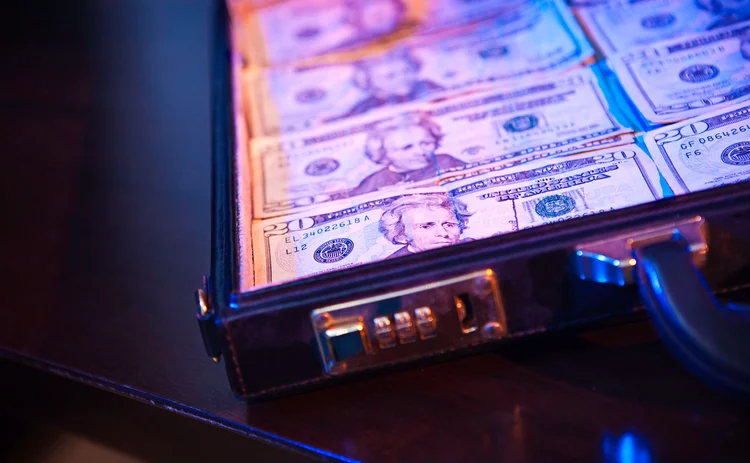
Two years after the US Federal Reserve gave its blessing to banks to pursue artificial intelligence-led approaches to combat financial crime, lenders fear the pendulum might be about to swing back the other way.
Banks have found great early success in piloting machine-learning techniques to spot suspicious transactions and identify weaknesses in existing controls, with their power to divine patterns in disparate datasets making them more effective than legacy rules-based systems that find too many false positives. But the approaches come with the added cost of having to explain to regulators and other stakeholders how the systems derive their results.
Banks are wrestling with demands from both regulators and stakeholders, such as bank management and internal auditors, that they are able to explain how systems came up with results, as well as any gaps in data. For example, say bank financial crime leads, models will uncover a gap that had existed in a historical dataset that manifests itself months after the new system has gone live without telling model managers how it arrived at that conclusion.
“We have knowledge to do that with a rules-based legacy platform. We do not know how to do that with machine-learning solutions—and you can’t tell regulators that you aren’t able to do a lookback,” said Jayati Chaudhury, global investment banking lead for AML transaction monitoring at Barclays, during a Risk.net webinar on June 30.
In recent examinations, banks say prudential regulators have been pushing them to demonstrate the explainability of ML models. A request for information by US regulators on the use of AI also included pointed questions on explainability, which has led to speculation that regulators may be considering imposing additional rules surrounding AI-based models.
ML approaches vary greatly in their complexity, but many banks have seen great promise in catching money launderers by training neural-networking algorithms to sift through vast datasets of transactions, and sniff out irregularities by divining non-linear correlations in methods that mimic the way the human brain operates. Models that make use of such techniques make millions of possible combinations before arriving at a result.
The idea is to use advanced analytics to find patterns that classical AML systems would miss: whereas older rules-based systems classify transactions according to pre-set criteria such as a counterparty’s age, occupation, and income, which are determined by analyzing existing data, neural networking uses advanced statistical techniques to detect anomalies in behavior. For example, a customer could have accounts in a bank’s corporate, correspondent banking, and institutional brokerage businesses. Analyzing the transaction activity among these different relationships may highlight suspicious activity that had not surfaced previously.
Given such decisions touch directly on customers, they carry heightened regulatory scrutiny, and the possibility of lawsuits and reputational damage if banks get them wrong.
“Monitoring for bias has been an important step for us to get comfortable when deploying these solutions,” said Patrick Dutton, senior vice president of financial crime and compliance at HSBC USA, during the webinar. “You need to monitor for particular variables that the model’s picking up on, maybe gender or nationality. We have found that data selection and variable selection is key.”
One single transaction can look perfectly legitimate but when you have a holistic view of the customer and see all the transactions the customer is making, you can detect anomalies
Jayati Chaudhury, Barclays
A report released last week by the Wolfsberg Group—a consortium of 13 large banks designed to formulate policies and standards and share best practice on combating financial crime—said that lenders needed to prove the effectiveness of their AML systems based on their compliance with existing laws, ability to provide useful information to authorities, and application of risk-based controls.
In parallel, US lawmakers have also been upping the ante for banks to improve the effectiveness of anti-money laundering systems. On June 30, the Financial Crimes Enforcement Network (FinCEN), a division of the US Treasury, issued the first set of national priorities mandated by the 2020 AML Act that entered force in the US on January 1.
Financial institutions will need to incorporate these priorities—targeting corruption, cyber crime, terrorist financing, fraud, transnational criminal activity, drug trafficking and human trafficking, and proliferation financing—into their risk-based AML programs, FinCEN said.
FinCEN has previously given its backing to banks to develop AML programs based on their own assessments, as well as those communicated by authorities in the form of national priorities. These programs are judged based on their overall effectiveness, as well as more narrow technical compliance with the US’s Bank Secrecy Act (BSA).
Banks see the opportunity for AI-based systems to satisfy these new standards being set down by government as well as their own internal stakeholders.
“Some good signs have come up in the last couple of months, which continue to push us this way. Guidance coming out of the US government has stated that BSA/AML models need to be flexible to deal with the changing threat environment and the fact that we need to be innovative and creative in our solutions,” said Dutton.
HSBC has used machine learning to segment individuals doing business with it via correspondent banking relationships into “pseudo customers” based on frequency of transaction, country of origin, or counterparty. The bank has developed around 400 characteristics that feed into its pseudo customer segments.
“By being able to segment the data, the number of segments that had a [suspicious activity report] went from 96% to 50%—so we can have better-targeted risk coverage across customers that are the most problematic,” said Dutton.
Machine learning is being used for surveillance when a customer has multiple relationships with a bank, as for example, through corporate, brokerage, and correspondent banking accounts. The algorithms can more easily detect anomalies across these relationships than would be possible by humans.
“Customers who are intending to misuse our financial systems are flying under the radar. One single transaction can look perfectly legitimate but when you have a holistic view of the customer and see all the transactions the customer is making, you can detect anomalies,” said Chaudhury.
Further reading
Only users who have a paid subscription or are part of a corporate subscription are able to print or copy content.
To access these options, along with all other subscription benefits, please contact info@waterstechnology.com or view our subscription options here: http://subscriptions.waterstechnology.com/subscribe
You are currently unable to print this content. Please contact info@waterstechnology.com to find out more.
You are currently unable to copy this content. Please contact info@waterstechnology.com to find out more.
Copyright Infopro Digital Limited. All rights reserved.
As outlined in our terms and conditions, https://www.infopro-digital.com/terms-and-conditions/subscriptions/ (point 2.4), printing is limited to a single copy.
If you would like to purchase additional rights please email info@waterstechnology.com
Copyright Infopro Digital Limited. All rights reserved.
You may share this content using our article tools. As outlined in our terms and conditions, https://www.infopro-digital.com/terms-and-conditions/subscriptions/ (clause 2.4), an Authorised User may only make one copy of the materials for their own personal use. You must also comply with the restrictions in clause 2.5.
If you would like to purchase additional rights please email info@waterstechnology.com
More on Emerging Technologies
This Week: Startup Skyfire launches payment network for AI agents; State Street; SteelEye and more
A summary of the latest financial technology news.
Waters Wavelength Podcast: Standard Chartered’s Brian O’Neill
Brian O’Neill from Standard Chartered joins the podcast to discuss cloud strategy, costs, and resiliency.
SS&C builds data mesh to unite acquired platforms
The vendor is using GenAI and APIs as part of the ongoing project.
Chevron’s absence leaves questions for elusive AI regulation in US
The US Supreme Court’s decision to overturn the Chevron deference presents unique considerations for potential AI rules.
Reading the bones: Citi, BNY, Morgan Stanley invest in AI, alt data, & private markets
Investment arms at large US banks are taken with emerging technologies such as generative AI, alternative and unstructured data, and private markets as they look to partner with, acquire, and invest in leading startups.
Startup helps buy-side firms retain ‘control’ over analytics
ExeQution Analytics provides a structured and flexible analytics framework based on the q programming language that can be integrated with kdb+ platforms.
The IMD Wrap: With Bloomberg’s headset app, you’ll never look at data the same way again
Max recently wrote about new developments being added to Bloomberg Pro for Vision. Today he gives a more personal perspective on the new technology.
LSEG unveils Workspace Teams, other products of Microsoft deal
The exchange revealed new developments in the ongoing Workspace/Teams collaboration as it works with Big Tech to improve trader workflows.