Are there limits to machine learning in trade surveillance?
Nick Wallis says that blending rules-based, machine learning and automation techniques can help overcome trade surveillance challenges.
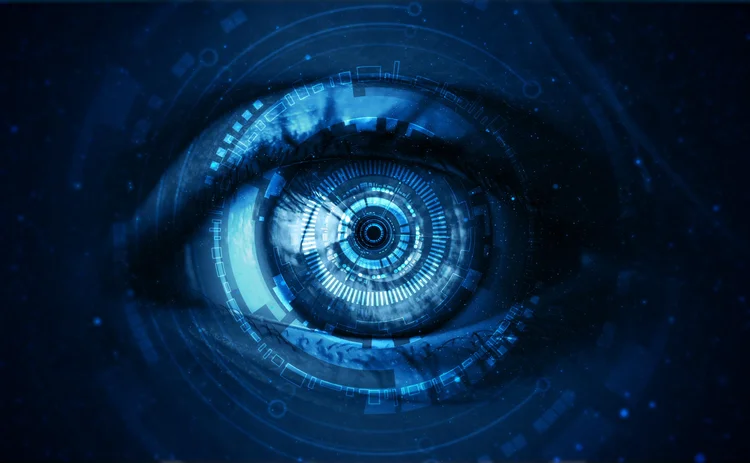
Compliance professionals face the daunting task of making sense of mounds of data, alerts, and shifting regulations. The power of AI and machine learning (ML), as evidenced by the text-generating software ChatGPT, has ignited imaginations as to how new tools can empower teams to achieve more accurate results more quickly. For compliance officers, the allure of ML is that it can address the problems inherent in most surveillance systems: They generate too many alerts and false positives, leading to the costly endeavor of humans having to investigate.
Yet reliance on AI/ML for alert generation comes with its own challenges. However, an alternative exists.
Challenge 1: Explainability
Regulators want explainability. Last year, the Bank of England and Financial Conduct Authority (FCA) issued a report noting that the “complexity of AI models can result in greater predictive accuracy, but can also make it harder to explain outputs.” These UK regulators stressed it was “vital” that financial firms use models they can explain. Likewise, the US Commodity Futures Trading Commission (CFTC) warned that licensed firms that use AI “remain responsible for all compliance requirements,” including making sure the AI system is working as intended and does not “generate or exacerbate systemic risk.”
The level of transparency in AI/ML models for trade surveillance varies depending on the application. For example, alerts may run through a supervised learning model that scores them based on how users previously tagged similar alerts. The result is a ranked order that allows analysts to focus on those with the higher probability score. To maintain explainability, the reasons for the initial alert remain visible. Importantly, ML is not used to generate the alerts but instead to score them.
In contrast, deep-learning ML models use multiple, hidden layers of calculations to make predictions. To work accurately, such a model requires lots of real or manufactured data, and enough examples of the specific type of manipulation it aims to identify. Unsupervised learning models will attempt to make associations not previously identified. Often the first versions of these models fail to work as planned and generate alerts without a ranking score or explanation.
Given the complexity, a compliance team must ask, “Can we explain our model to a regulator? Can we understand why our model flagged this trading behavior and not another one? Are we sure it’s not adding risk?”
Challenge 2: Overfitting
An AI/ML model must combine the right algorithm with training data representative of what the system will see when deployed. In contrast, rules-based systems—such as “return Z if X is greater than Y”—can “underfit” the data, potentially missing nuances. The AI/ML selling point is that it discovers hidden patterns that humans cannot see or anticipate when programming rules.
Yet AI/ML models run into the opposite problem, overfitting. According to IBM, “When the model memorizes the noise and fits too closely to the training set, the model becomes ‘overfitted,’ and it cannot generalize well to new data. If a model cannot generalize well to new data, then it will not be able to perform the classification or prediction tasks that it was intended for.”
This concern about what happens to AI/ML models when they face “new data” is especially relevant for financial markets. Historical data is plentiful, but new financial paradigms emerge all the time. For example, we have much less training data for financial market behavior in an era of high inflation, rising interest rates, supply chain disruptions, major power conflicts, and crypto volatility.
Challenge 3: Bias
Related to overfitting, the various types of bias in AI/ML models are well-known and studied. The Monetary Authority of Singapore (MAS), in a June 2022 information paper, gave an example of a credit score model that could be unintentionally biased against poor women, as it used historical data. The MAS urged financial institutions to analyze whether their models incorporated “FEAT Principles”: fairness, ethics, accountability, and transparency.
In trade surveillance, compliance teams should ask whether their surveillance alerts could be biased, such as against contrarian trades or smaller clients. If so, they might be missing larger, more impactful manipulative trading behavior or contributing to risk. Again, a machine-learning model lacking explainability may not necessarily allow for such close examination. The market is already aware of this problem. In an October 2022 industry survey from the Bank of England, the top identified ML-related risk was “biases in data, algorithms, and outcomes,” followed by “data quality and structure issues.”
Challenge 4: Finding ML talent
Even if a firm accepts these inherent challenges and deploys an AI/ML model, it faces another barrier: finding and hiring AI/ML talent to build and regularly retrain and retune models to fit dynamic needs. Regulators clearly state that the licensed firms are responsible for the ML models they deploy.
In addition to the complex task at hand, an AI/ML team is expensive. According to Indeed, the average base salary of a single machine-learning engineer working in London is about £72,195 ($87,300), which would certainly be much more for the finance industry. The costs quickly increase as more team members—engineers, data scientists and software developers—are added. No compliance system is “set it and forget it,” no matter how sophisticated it sounds.
An alternative approach
Considering the challenges outlined, a top concern of compliance officers remains: How can a market surveillance system return quality alerts while also meeting regulatory guidelines on explainability, and reducing overfitting and bias—and do so within budget?
An alternative approach is to mix rules-based, ML, and automation techniques while maintaining clear documentation at each step.
This approach starts with rules-based parameters tuned for each individual client and their market. This “casts a wide net” to collect a swath of candidate alerts. Then, only when appropriate an ML model—trained dynamically—can score alerts on relevant trading behaviors such as spoofing and layering, and tag them with justifications. Finally, robotic process automation sifts through alerts following the guidance of a trained surveillance analyst and flags only the most relevant for additional human investigation. This method reduces false positives while preserving candidate alerts for supervisory review, pattern, and practice analysis.
Every approach has its pros and cons. The key is to be aware of each method’s risks and responsibilities, and how to transparently explain decisions to internal business partners and regulators.
Nick Wallis is managing director for EMEA at Eventus
Only users who have a paid subscription or are part of a corporate subscription are able to print or copy content.
To access these options, along with all other subscription benefits, please contact info@waterstechnology.com or view our subscription options here: http://subscriptions.waterstechnology.com/subscribe
You are currently unable to print this content. Please contact info@waterstechnology.com to find out more.
You are currently unable to copy this content. Please contact info@waterstechnology.com to find out more.
Copyright Infopro Digital Limited. All rights reserved.
As outlined in our terms and conditions, https://www.infopro-digital.com/terms-and-conditions/subscriptions/ (point 2.4), printing is limited to a single copy.
If you would like to purchase additional rights please email info@waterstechnology.com
Copyright Infopro Digital Limited. All rights reserved.
You may share this content using our article tools. As outlined in our terms and conditions, https://www.infopro-digital.com/terms-and-conditions/subscriptions/ (clause 2.4), an Authorised User may only make one copy of the materials for their own personal use. You must also comply with the restrictions in clause 2.5.
If you would like to purchase additional rights please email info@waterstechnology.com
More on Emerging Technologies
This Week: Startup Skyfire launches payment network for AI agents; State Street; SteelEye and more
A summary of the latest financial technology news.
Waters Wavelength Podcast: Standard Chartered’s Brian O’Neill
Brian O’Neill from Standard Chartered joins the podcast to discuss cloud strategy, costs, and resiliency.
SS&C builds data mesh to unite acquired platforms
The vendor is using GenAI and APIs as part of the ongoing project.
Chevron’s absence leaves questions for elusive AI regulation in US
The US Supreme Court’s decision to overturn the Chevron deference presents unique considerations for potential AI rules.
Reading the bones: Citi, BNY, Morgan Stanley invest in AI, alt data, & private markets
Investment arms at large US banks are taken with emerging technologies such as generative AI, alternative and unstructured data, and private markets as they look to partner with, acquire, and invest in leading startups.
Startup helps buy-side firms retain ‘control’ over analytics
ExeQution Analytics provides a structured and flexible analytics framework based on the q programming language that can be integrated with kdb+ platforms.
The IMD Wrap: With Bloomberg’s headset app, you’ll never look at data the same way again
Max recently wrote about new developments being added to Bloomberg Pro for Vision. Today he gives a more personal perspective on the new technology.
LSEG unveils Workspace Teams, other products of Microsoft deal
The exchange revealed new developments in the ongoing Workspace/Teams collaboration as it works with Big Tech to improve trader workflows.