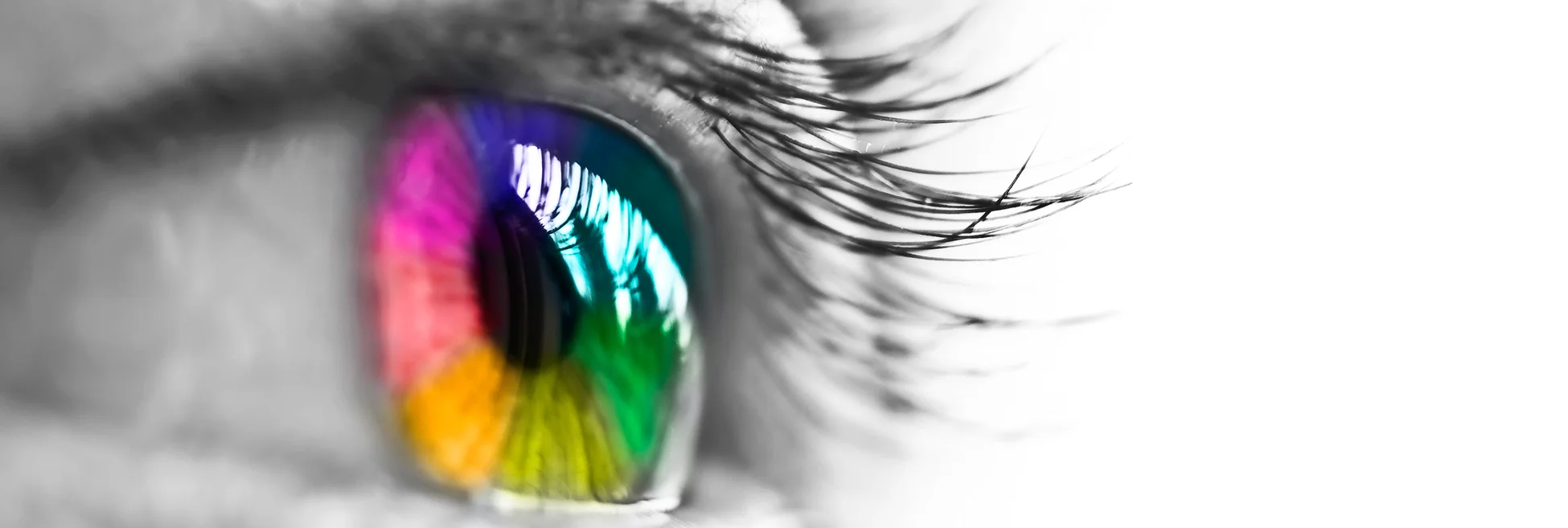
At the ‘Fringes of Realism’: Agent-Based Models Take Hold Among Quants
Agent-based modeling has taken root seemingly everywhere throughout the last decade, from theoretical physics, to military operations, to public health, to ride-sharing apps like Uber, and to a much lesser extent, finance. However, a year such as 2020—and all its ups and downs—could drive firms to the edge of this new frontier.
Need to know
- Agent-based modeling breaks away from stochastic, or mathematic, varieties of modeling and forecasting. Though more commonly used for military operations, epidemiology, urban planning and other concentrations, it is gaining traction among some quant trading shops.
- ABM potentially has huge implications for the way banks and asset managers of all stripes conduct stress-testing and mitigate their risk, as it seeks to simulate the rational and irrational behaviors of agents—individuals and groups—in their environments.
- However, the method is not without criticisms. Because it is rooted in physics, rather than math, ABM requires a different skill set than that of the typical economist and banker. And, because it aims to re-create the real, and often irrational, world from the bottom up, models’ outputs are essentially limitless, and can be seen as arbitrary or impossible to calibrate.
- Users note that as the world changes faster than it has before in shorter time periods, it serves as a useful tool that can help anticipate and manage black swans, financial crises, or rapid economic shifts.
Many things changed on September 11, 2001, and apart from the profound sense of loss, there was also stunned disbelief—a question of “How?”
In the aftermath, Justin Lyon enrolled in a graduate program at MIT, studying system dynamics, or, to put it very simply, how complex things—systems with many moving parts—act and change over time. There, he focused on simulating the growth of radical Islam—that is, predicting likelihoods of violence, insurgencies, and counterinsurgencies in tandem with potential consequences that could follow—in Afghanistan, using a technique called complex adaptive systems modeling. Operating under the theory that the universe is composed of highly complicated, in-flux, and interconnected systems, Lyon set out to determine how to model humans and the ways in which they interact in a way that acknowledges and adjusts for all the nuance that colors their rational and irrational behaviors.
Over the better part of the next two decades, Lyon worked as a contractor for a number of organizations, including the Bank of England, ExxonMobil, Microsoft, and the US Department of Defense, for which he ran a team of analysts supporting a general based in Iraq.
Five years after 9/11, but eight years before Lyon would start and head up his own company called Simudyne, the 2008 financial crisis rocked the global financial system, and marked a clear turning point in the prevailing views economists took toward markets—that they were inherently efficient and rational. They aren’t, and increasingly, it’s dangerous to believe so.
“All models are ultimately wrong because they aren’t the real world. But you want to have an ecosystem—a zoo of models,” says Lyon, echoing a term coined by Andrew Haldane of the Bank of England. “A zoo of models: a whole bunch of different, diverse species of models so that you’re not blindsided.”
When Lyon founded Simudyne, an enterprise simulation technology company, in 2016, his thesis was that people could make radically better decisions that ultimately lead to a safer world. People could do this, in part, by using agent-based modeling, which draws upon his earlier work modeling complex adaptive systems.
The range of possible financial applications for this emerging quant technique is vast. A small universe of whitepapers and research studies pertaining to agent-based modeling and finance has exploded in the last five years, including from Refinitiv, the Bank of England, the Securities and Exchange Commission, and Deloitte. They put forth cases for its use in areas such as high-frequency trading, execution, market-making, risk management, and predicting and understanding black swans—which, as the future begins to look less and less like the past, may fan the modeling method’s slow burn into a blaze.
.jpg.webp?itok=onINJjig)
Who, What, and Why
As a rudimentary definition, agent-based modeling is a bottom-up approach to simulating the real-world behavior of agents—a single trader on a small scale, the global financial system on a larger one—without basic assumptions that the agents in their environment behave in perfectly rational ways or have perfect information on which to base decisions.
An agent-based-model can be broken down into two components: one that is structural and one that is behavioral, says Krishnen Vytelingum, head of quantitative modeling at Simudyne. The structural piece is the environment where buyers and sellers interact, such as on an exchange, or an actual physical space, such as a city map within the ride-hailing app Uber, where riders and drivers meet. The structure constitutes the rules of the game—the mechanisms players use to conduct activities and interact—but the behavioral component accounts for what players could actually do, which can be anything from very simple, even random behavior, to extremely complicated behavior.
With traditional modeling methods used in finance—primarily stochastic factor modeling, which includes Monte Carlo simulations—you model what you observe. And a major shortcoming of traditional methods, says Vytelingum, is that they assure, dangerously, that the failure of one institution is independent from another. Because institutions are inherently connected and exposed to one another, the downfall of one behemoth likely dominoes to another, which can make it extremely difficult to model an accurate view of credit risk.
Stochastic simulations can be quite sophisticated from a mathematical standpoint, but are ultimately glorified endeavors in extrapolation, says Jean-Philippe Bouchaud, chairman and chief scientist of Paris-based quant fund Capital Fund Management (CFM) and a physicist by trade. Instead, by approaching the world through a physics lens, you try to model the behaviors that lead to outcomes.
“Traffic jams are really special,” he says. “You run into a traffic jam, and you don’t know why the traffic jam is there, when suddenly it just kind of vanishes into thin air.”
Given an open road, logical behavior should dictate that drivers will accelerate to the maximum allowed speed and continue that way until they exit the road or until something blocks the path, at which point they would brake to avoid a collision. In a perfect world, the driver would always stop, and cars would never crash.
But in the real world, says Bouchaud, maybe drivers have slightly different response times. Maybe some of them are distracted. Maybe some are prone to admiring landscapes or birds, or are prone to daydreaming. Maybe all of these things are true at once. It may only take a minute or two of lost concentration for a car’s velocity to drop enough that a procession of angry commuters forms.
“All of [these little events] can cascade into something major,” he says.
Of course, this is a simple example, but it’s translatable to the languages of financial crashes, economic crises, or a group of fireflies flashing in unison. All of these are examples of emergent phenomena—that is, collective behaviors—that are difficult to predict.
Ten years ago, CFM, which has more than $7 billion in assets under management, began using agent-based modeling to understand the firm’s own impact on the market when it placed market or limit orders. The firm created a fictitious market with market-makers and other types of traders interacting with a simulated version of the real firm, testing various strategies and their effects. The exercise was useful, Bouchaud says, because the leeway one has to conduct experiments using people’s real money is not exactly that much.
Bouchaud was most recently involved in what he calls an exciting new development for agent-based modeling in finance, using it to analyze how liquidity crises are generated sometimes spontaneously and seemingly for no reason, much like the idea of a traffic jam.
Lisa Borland, a senior machine-learning scientist at San Francisco-based hedge fund Cerebellum Capital, joined the financial world after receiving formal training as a theoretical physicist, trying to understand how macroscopic phenomena—think, for example, perfectly structured cloud formations—emerge. Finance offered her similarly complex problems to solve, and she became especially interested in studying the price formation process as one of those complex systems.
For instance, you could create a “toy system” containing a fictitious stock, for which there is a fixed supply. The agents trading the stock could be programmed with simple trading rules—some would trade on recent trends, others on the stock’s time series, and others based on the fundamental value of the stock. Then, you would let the simulation run. What you’d find is that, even though you don’t have a mathematical way of deriving it, the price would evolve and replicate in the same manner seen in real markets.
“They become great tools if you want to understand how changing the rules would affect the system,” Borland says.
Simudyne’s Vytelingum found something similar: Even when agents were of “zero intelligence,” meaning they bought and sold at random and didn’t abide by any trading rules, they produced an efficient market. One could take this to mean that traders don’t need to be extra smart or clever, or even efficient, to mimic the kind of rationality typically seen in the market.
But this finding also lends credence to a main criticism of agent-based models—that they aren’t calibrated to the real world because the models could be constructed in a multitude of ways that could each, potentially, give rise to the same phenomena.
.jpg.webp?itok=KVwt8zNf)
Predicting the Past to Predict the Future
As the 2008 financial crisis was taking hold in the US, theoretical physicist Mark Buchanan penned an opinion piece for the New York Times titled “This Economy Does Not Compute,” in which he described agent-based computer simulation as a “telescope of the mind” that “[multiplies] human powers of analysis and insight just as a telescope does our powers of vision.”
Almost an opposite approach from “what-if” testing—wherein the user identifies a possible event and, based on largely static conditions, looks for how it would play out—an agent-based model gives the user a range of its own possible scenarios, many of which the user likely hadn’t ever considered.
On one hand, it could push the boundaries of the ways in which organizations manage and mitigate risk. On the other, because these models are rooted in unpredictability, and the number of possible outcomes vast, one could argue these simulations are mostly arbitrary. In theory, agent-based modeling could teach firms to be prepared for anything, but in practice, they could certainly not be prepared for everything.
However, firms may have little choice but to try because increasingly, the future does not look so much like the past, says Jochen Leidner, Refinitiv’s director of research.
Last summer, Refinitiv partnered with Simudyne on a project to predict price movements using a combination of agent-based modeling, synthetic market data, and machine learning. The team, which included Leidner, Vytelingum and two others, worked on the premise that using the past—in this case, historical market data patterns—was a deeply flawed way to predict future price movements.
“Whie it’s entirely the right thing—so I’m saying nothing against back-testing at all—it is not alone sufficient to deal with the complexity of this world,” Leidner says. “You may also want to explore alternative futures that may not be based on the actual history, but on potential alternative histories—so not on the past as it happened, but on the past as it could have happened.”
This is a weird notion, he admits. After all, everyone agreed there’s only one past. So the idea was to create synthetic data that looked as if it could have been a possible past, in the sense that it shared certain properties that financial markets are known to exhibit, such as seasonality and regularity; these are otherwise known as stylized facts. Real historical market data notwithstanding, the team created purely random—made-up—data that, despite its randomness, structurally and distributionally obeyed the laws of finance as they know them, making it plausible.
“That means we can now no longer just rely on one history—the one that actually happened, the only thing that we really know for sure—but we can also explore the fringes of realism,” Leidner says. “And we can see how our models behave on these fringes and these fictionalized versions of the almost-past that could have happened but didn’t.”
While it may seem like this story about agent-based modeling is veering sharply into science-fiction territory, the concept Leidner describes has a very practical application: stress-testing your models in a world that’s changing at a faster pace than ever before.
Pandemics are sickening populations at an alarming rate. In the 21st century alone, outbreaks have included SARS, swine flu, MERS, Ebola, Zika, Dengue fever, and Covid-19, in addition to other epidemics with smaller reaches, and experts have warned that global viruses will become more common. At the same time, climate change has become a real, measurable threat with far-reaching implications and an ever-shortening time-frame to counteract it. And more recently, conventional forecasts failed to predict political upsets such as the 2016 election of President Donald Trump, and the UK’s decision to leave the EU.
“The world is increasingly running off-script, or deviating from just being a linear consequence of the last couple of years. … That is something that makes it hard to model [the world] just based on the past. And it could therefore be, and that’s our contention, that we may want to use synthetic data in a wisely-applied form to expand our toolkit for stress-testing our models that we may want to create for trading or compliance,” Leidner says.
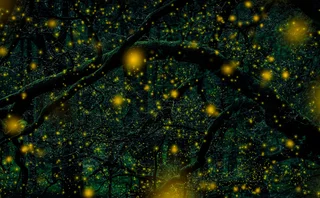
War Games
If, at the least, agent-based models aren’t yet useful at the event-prediction level, they can serve as a kind of war game, or tactical exercise, for banks and traditional asset managers that don’t yet utilize them. Though today the models are concentrated among a handful of quant funds, Barclays, which led a series A round of funding in Simudyne, is one example of an early adopter. C.S. Venkatakrishnan, Barclays’ global head of markets, has written about the bank’s projects with Simudyne’s software, but was unable to comment in time for publication.
CFM’s Bouchaud, who has been working in this field for the last 20 years, says physicists and financial quants are indeed already thinking up the next evolution of models, which he says would be to have an agent-based model that’s accurate enough to tell a trader whether they should buy Apple or PepsiCo, for examples.
“That would be great. But before we get there, I think that the mere fact of having these scenario generators is extremely important from a risk-control point of view,” Bouchaud says. “It is hard to guess the right direction in which stocks are going to go, but if you can avoid major events, that’s already something good.”
Only users who have a paid subscription or are part of a corporate subscription are able to print or copy content.
To access these options, along with all other subscription benefits, please contact info@waterstechnology.com or view our subscription options here: http://subscriptions.waterstechnology.com/subscribe
You are currently unable to print this content. Please contact info@waterstechnology.com to find out more.
You are currently unable to copy this content. Please contact info@waterstechnology.com to find out more.
Copyright Infopro Digital Limited. All rights reserved.
As outlined in our terms and conditions, https://www.infopro-digital.com/terms-and-conditions/subscriptions/ (point 2.4), printing is limited to a single copy.
If you would like to purchase additional rights please email info@waterstechnology.com
Copyright Infopro Digital Limited. All rights reserved.
You may share this content using our article tools. As outlined in our terms and conditions, https://www.infopro-digital.com/terms-and-conditions/subscriptions/ (clause 2.4), an Authorised User may only make one copy of the materials for their own personal use. You must also comply with the restrictions in clause 2.5.
If you would like to purchase additional rights please email info@waterstechnology.com
More on Data Management
New working group to create open framework for managing rising market data costs
Substantive Research is putting together a working group of market data-consuming firms with the aim of crafting quantitative metrics for market data cost avoidance.
Off-channel messaging (and regulators) still a massive headache for banks
Waters Wrap: Anthony wonders why US regulators are waging a war using fines, while European regulators have chosen a less draconian path.
Back to basics: Data management woes continue for the buy side
Data management platform Fencore helps investment managers resolve symptoms of not having a central data layer.
‘Feature, not a bug’: Bloomberg makes the case for Figi
Bloomberg created the Figi identifier, but ceded all its rights to the Object Management Group 10 years ago. Here, Bloomberg’s Richard Robinson and Steve Meizanis write to dispel what they believe to be misconceptions about Figi and the FDTA.
SS&C builds data mesh to unite acquired platforms
The vendor is using GenAI and APIs as part of the ongoing project.
Aussie asset managers struggle to meet ‘bank-like’ collateral, margin obligations
New margin and collateral requirements imposed by UMR and its regulator, Apra, are forcing buy-side firms to find tools to help.
Where have all the exchange platform providers gone?
The IMD Wrap: Running an exchange is a profitable business. The margins on market data sales alone can be staggering. And since every exchange needs a reliable and efficient exchange technology stack, Max asks why more vendors aren’t diving into this space.
Reading the bones: Citi, BNY, Morgan Stanley invest in AI, alt data, & private markets
Investment arms at large US banks are taken with emerging technologies such as generative AI, alternative and unstructured data, and private markets as they look to partner with, acquire, and invest in leading startups.