Banks Embrace the Use of Synthetic Data
Banks have long been using synthetic data to validate solutions, but tech advancements and regulatory pressure have established this practice as a crucial step in the development and testing of technologies.
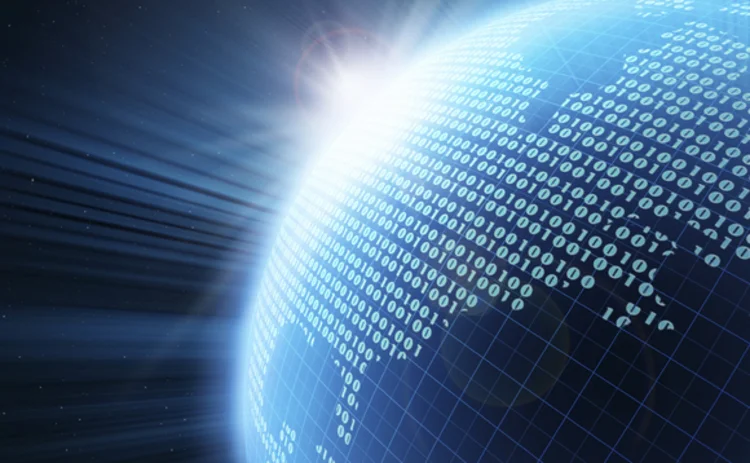
Synthetic data is playing an increasingly important part in testing solutions, particularly as pressure mounts on banks to harness newer, more sophisticated technologies while complying with a raft of regulation.
Although auto-generated data is not a new tool for many banks, it has proven particularly useful in recent cases where firms look to adopt new capabilities, similar to those of Netflix, Amazon or Google, that require vast amounts of data.
According to Giuseppe Nuti, head of algorithmic trading at UBS investment bank, replicating functionality that can, for example, recommend investment products to clients and understand user preferences involves the processing of huge volumes of data, a capability that big tech firms have in orders of magnitude more than investment banks.
In these cases, the synthetic data is used to compensate for the lack of testing data required to validate the technology to a comparable standard as that of Silicon Valley applications.
“We are talking tens if not hundreds of millions of users actively buying stuff or watching movies, versus a few hundred clients for a bank,” Nuti says. “Even in the biggest of investment banks and for the most successful of its desks, we are talking 200 to 300 active clients. The statistical difference is substantial—hence the need for synthetic data.”
Synthetic datasets are often based on vast amounts of reproduced historical data that includes insights or patterns that have already been identified. It is used to validate algorithms and AI-driven models, but only tests against predictable outcomes or previously determined answers. Yet, Nuti says, the data is a crucial component in evaluating a broad set of functionality and sits at the core of UBS’s development process.
“It is a necessary step that you need to take,” he says. “It doesn’t guarantee a solution because the world may not behave the way you thought it would, but it certainly ensures that if it does, you are able to pick that up,”
As one example, synthetic data has been incorporated into the development and testing of UBS’s recommendations engine, which is used to suggest trades to its asset managers and hedge funds and to identify potential clients. Algorithms are trained to analyze user behavior and provide automated suggestions based on their activity. Synthetic data comes into play when the technologies are used to test patterns and offer analysis before using the algorithms on real client data.
Data Protection
In many cases, the reason for using synthetic data is tied to compliance and the need to avoid using client data for testing solutions. As financial institutions are under increasing pressure to comply with global data protection and privacy laws, such as the General Data Protection Regulation (GDPR), they are having to take specific measures to adhere to cross-border data sharing and prevent client data getting into the hands of unauthorized users.
“When you have large reams of corporate client information such as we do, we have an obligation to respect the client around cross-border data sharing and there are very strict controls around that,” a senior data executive at a tier 1 bank says. “Instead, we are exploring how we can use synthetic data. So we can generate artificial data such as credit payments or whatever it may be and then use that for development use cases.”
The bank is currently in the early stages of research and development on testing synthetic data compliance use cases. According to the executive, the adoption of cloud technology or cloud environments will prove very useful in generating and storing vast amounts of synthetic data in a more on-demand and efficient way in the future.
However, they add, while synthetic can be very effective in testing technologies, it is not applicable everywhere. They say that for unpredictable anomaly detection, for instance, synthetic data can create its own issues.
“It doesn’t work for all types of use cases,” the data executive says. “It does help us when we are looking at historical pattern analysis, time series analysis and that sort of thing. If you are trying to do anomaly detection you don’t necessarily want to artificially generate data [for that use case] because it is almost as if you are planting an anomaly.”
Only users who have a paid subscription or are part of a corporate subscription are able to print or copy content.
To access these options, along with all other subscription benefits, please contact info@waterstechnology.com or view our subscription options here: http://subscriptions.waterstechnology.com/subscribe
You are currently unable to print this content. Please contact info@waterstechnology.com to find out more.
You are currently unable to copy this content. Please contact info@waterstechnology.com to find out more.
Copyright Infopro Digital Limited. All rights reserved.
As outlined in our terms and conditions, https://www.infopro-digital.com/terms-and-conditions/subscriptions/ (point 2.4), printing is limited to a single copy.
If you would like to purchase additional rights please email info@waterstechnology.com
Copyright Infopro Digital Limited. All rights reserved.
You may share this content using our article tools. As outlined in our terms and conditions, https://www.infopro-digital.com/terms-and-conditions/subscriptions/ (clause 2.4), an Authorised User may only make one copy of the materials for their own personal use. You must also comply with the restrictions in clause 2.5.
If you would like to purchase additional rights please email info@waterstechnology.com
More on Data Management
New working group to create open framework for managing rising market data costs
Substantive Research is putting together a working group of market data-consuming firms with the aim of crafting quantitative metrics for market data cost avoidance.
Off-channel messaging (and regulators) still a massive headache for banks
Waters Wrap: Anthony wonders why US regulators are waging a war using fines, while European regulators have chosen a less draconian path.
Back to basics: Data management woes continue for the buy side
Data management platform Fencore helps investment managers resolve symptoms of not having a central data layer.
‘Feature, not a bug’: Bloomberg makes the case for Figi
Bloomberg created the Figi identifier, but ceded all its rights to the Object Management Group 10 years ago. Here, Bloomberg’s Richard Robinson and Steve Meizanis write to dispel what they believe to be misconceptions about Figi and the FDTA.
SS&C builds data mesh to unite acquired platforms
The vendor is using GenAI and APIs as part of the ongoing project.
Aussie asset managers struggle to meet ‘bank-like’ collateral, margin obligations
New margin and collateral requirements imposed by UMR and its regulator, Apra, are forcing buy-side firms to find tools to help.
Where have all the exchange platform providers gone?
The IMD Wrap: Running an exchange is a profitable business. The margins on market data sales alone can be staggering. And since every exchange needs a reliable and efficient exchange technology stack, Max asks why more vendors aren’t diving into this space.
Reading the bones: Citi, BNY, Morgan Stanley invest in AI, alt data, & private markets
Investment arms at large US banks are taken with emerging technologies such as generative AI, alternative and unstructured data, and private markets as they look to partner with, acquire, and invest in leading startups.