Bloomberg Adds New NLP Capabilities to TOMS
The tool allows traders to search their own data to find trade information specific to them.
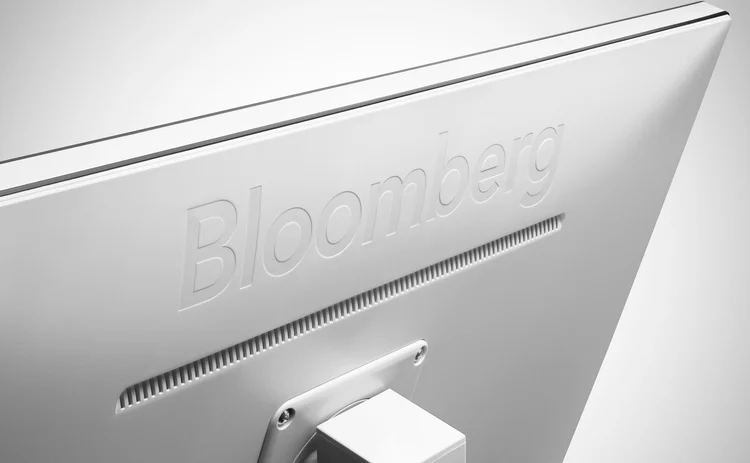
An eternal struggle for technology companies is preparing for long-term disruption while answering the day-to-day needs of customers. For Bloomberg’s sell-side solutions business, this meant carving out a group of engineers whose sole focus is building solutions that don’t necessarily meet the demands of clients today, but anticipates their needs in a few years as new technologies become democratized, regulations hit, and the world becomes more complex. At the same time, it’s the kind of forward-thinking move that can help future-proof the data and tech giant against cutting-edge startups.
As an example of how this unit tries to stay ahead of the curve, it developed a new search functionality that’s embedded into Bloomberg’s Trade Order Management Solutions (TOMS) Trade Analyzer.
This new question-answer interface is, at its core, an engine that heavily leverages natural language processing (NLP) and machine learning algorithms to deliver answers to questions that are unique to the needs of traders. It aims to reduce the number of clicks needed to search for and within certain datasets, such as querying trade histories with a buy-side customer or looking up missed trades during a given time period, Robert Simek, head of sell-side analytics at Bloomberg, tells WatersTechnology.
As soon as a trader or front-office user types in a question and gets the wrong answer, they’ll never use it again. The level of accuracy and understanding that we need for the product is a really high bar.
Robert Simek
Those arduous searches typically require going into a blotter and performing a host of manual tasks, such as adding and deleting columns, changing date ranges, and applying different filters. Alternatively, clients would often just export the data, or ask someone else on the desk to curate a report with the answers they sought. As a result, it could take hours or days for a trader to get an answer to what should be a simple question.
As much as an NLP model takes loads of training time and data to understand standard human language—as a simple example, how a month like August may be spelled out or abbreviated, but mean the same thing—the team spent just as long training it to understand phrases specific to the financial domain.
“There’s slang that’s used: What does a ‘completed trade’ mean? What does a ‘done trade’ mean? What does a ‘missed trade’ mean?” Simek says. “As soon as a trader or front-office user types in a question and gets the wrong answer, they’ll never use it again. The level of accuracy and understanding that we need for the product is a really high bar.”
From ideation to full production, the project was about a year in the making. The question-answer format is only the foundation, Simek emphasizes, as clients also want to use the interface to ask more analytical types of questions, such as wanting to compare the number of trades they do on platform A versus platform B.
Meeting that demand is where the team will take the project next. Because the machine-learning model learns over time, it can start to get a sense of patterns in questions asked, such as emerging trends, top bonds, or other topics that might require aggregation from multiple databases to get to an answer. As of today, the traditional database structure the tool currently utilizes requires too much time to answer more analytical questions.
Part of the remaining strategy, Simek says, is moving that data to more modern database structures that allow for more complex queries to yield an instant answer. Traditional relational databases are indexed on a single value—for example, the transaction ID. If a trader wants to ask a question of another column in the database, perhaps looking at counterparties in a particular sector, they’d ask, “Who are our top 5 accounts in the tech sector?,” then they would have to scan through every single record to find all the counterparties, group them, and then sum them. That’s why it takes too long, Simek says.
It’s similar to an Excel grid, he adds. To solve this problem you can use a non-relational database that can group together any data point that is represented in your dataset and make the data available much quicker then scanning all the records.
Today, as regular consumers, traders can easily use their mobile devices to answer questions by using a search engine like Google, but also access data related specifically to themselves. As apps become more interoperable, a cell phone can provide real-time info pertaining to an individual’s health and daily physical activity, for example.
At the enterprise level, traders are increasingly expecting that the same kind of functionality embedded into the tools they use every day are available in their professional tools. Simek says that although enterprise software has traditionally been built using a waterfall development process that has the software following specific written instructions, enterprise solution vendors increasingly have the engineering capacity to implement cutting-edge technologies like machine learning and more sophisticated NLP models. By working with end users, the adoption rate can more readily align with or even outpace the “diffusion of innovations” curve, Simek says.
“I think that gap today between the two [consumer tech and trading tech] is quite wide,” he says. “You’re on the train coming into work, using your phone, and you’re leveraging some of these modern technologies to search and find information,” he says. “Then you get to sit down and do your day job, and the software hasn’t caught up entirely. There’s various reasons for it. … But when you start to see this change, I think people get really excited about it.”
Further reading
Only users who have a paid subscription or are part of a corporate subscription are able to print or copy content.
To access these options, along with all other subscription benefits, please contact info@waterstechnology.com or view our subscription options here: http://subscriptions.waterstechnology.com/subscribe
You are currently unable to print this content. Please contact info@waterstechnology.com to find out more.
You are currently unable to copy this content. Please contact info@waterstechnology.com to find out more.
Copyright Infopro Digital Limited. All rights reserved.
As outlined in our terms and conditions, https://www.infopro-digital.com/terms-and-conditions/subscriptions/ (point 2.4), printing is limited to a single copy.
If you would like to purchase additional rights please email info@waterstechnology.com
Copyright Infopro Digital Limited. All rights reserved.
You may share this content using our article tools. As outlined in our terms and conditions, https://www.infopro-digital.com/terms-and-conditions/subscriptions/ (clause 2.4), an Authorised User may only make one copy of the materials for their own personal use. You must also comply with the restrictions in clause 2.5.
If you would like to purchase additional rights please email info@waterstechnology.com
More on Data Management
New working group to create open framework for managing rising market data costs
Substantive Research is putting together a working group of market data-consuming firms with the aim of crafting quantitative metrics for market data cost avoidance.
Off-channel messaging (and regulators) still a massive headache for banks
Waters Wrap: Anthony wonders why US regulators are waging a war using fines, while European regulators have chosen a less draconian path.
Back to basics: Data management woes continue for the buy side
Data management platform Fencore helps investment managers resolve symptoms of not having a central data layer.
‘Feature, not a bug’: Bloomberg makes the case for Figi
Bloomberg created the Figi identifier, but ceded all its rights to the Object Management Group 10 years ago. Here, Bloomberg’s Richard Robinson and Steve Meizanis write to dispel what they believe to be misconceptions about Figi and the FDTA.
SS&C builds data mesh to unite acquired platforms
The vendor is using GenAI and APIs as part of the ongoing project.
Aussie asset managers struggle to meet ‘bank-like’ collateral, margin obligations
New margin and collateral requirements imposed by UMR and its regulator, Apra, are forcing buy-side firms to find tools to help.
Where have all the exchange platform providers gone?
The IMD Wrap: Running an exchange is a profitable business. The margins on market data sales alone can be staggering. And since every exchange needs a reliable and efficient exchange technology stack, Max asks why more vendors aren’t diving into this space.
Reading the bones: Citi, BNY, Morgan Stanley invest in AI, alt data, & private markets
Investment arms at large US banks are taken with emerging technologies such as generative AI, alternative and unstructured data, and private markets as they look to partner with, acquire, and invest in leading startups.