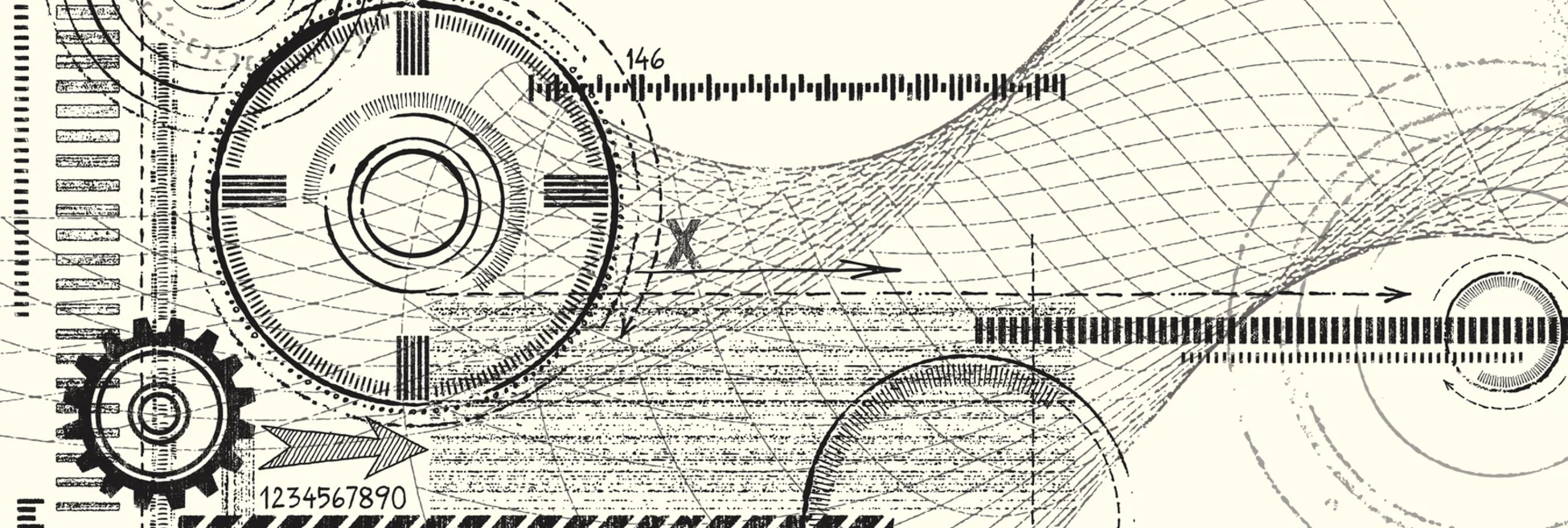
Bloomberg deploys math, not AI, to blend risk management and portfolio construction
The Mac3 GRM risk solution is live for equities users, uses no AI or machine learning, and will be rolled out to more asset classes next.
Investment management, the domain of portfolio managers and chief investment officers; and risk management, handled by risk analysts and chief risk officers, are closer to becoming a single, blended function rather than discrete operations, says Antonios Lazanas, head of portfolio and index research at data giant Bloomberg.
There are several reasons for this. One is the Covid-19 pandemic and the hidden correlations it revealed between seemingly separate economic sectors; another is the rising popularity of environmental, social, and governance (ESG) investing correlated with rising atmospheric temperatures. And so Bloomberg is betting that the industry’s risk modeling capabilities will have to become more sophisticated.
In response, Bloomberg rolled out its multi-asset class global risk model (Mac3 GRM) solution suite at the end of last year for equities managers. The company now plans to begin serving fixed-income users with the same product over the next six months. By the end of 2022, the aim is to complete the entire Mac3 GRM release with applications in commodities, private equity, and alternatives. Currently, Bloomberg is in the process of moving all of its equities clients onto Mac3.
“Risk managers continue to be worried about the same thing [as portfolio managers]: ‘What can happen to my portfolio?’. What’s happening is that on the portfolio construction side, things are getting more complicated, which means managers have more sources of returns and risk at their disposal,” Lazanas says.
The new suite is the culmination of combining Bloomberg Port with the Barclays Risk Analytics and Index Solutions (Brais) business that Bloomberg acquired in 2016 and is available to Terminal users and Enterprise users—companies that pay additional fees to run their businesses with Bloomberg’s models with ad hoc use of the Terminal—though some features are reserved for Enterprise users only.
One such feature is full-term structure of risk, wherein Bloomberg provides different risk customers with different portfolio, or rebalancing, horizons. Enterprise users can utilize timespans including daily, weekly, monthly quarterly, and yearly, but Terminal-only users can opt for weekly and quarterly measurements.
The second option Bloomberg has restricted is an advanced feature in Mac3’s tail risk model for handling option-like instruments. The Port tail risk model uses a simulation engine to generate a large number of market scenarios and calculates the portfolio return under each scenario. The worst scenarios are used to estimate a portfolio’s tail risk.
This is traditionally an expensive task, Lazanas says, because to get an adequate view of risk, a user needs to account for roughly 30,000 scenarios. This requires efficient calculation methods that rely on some sort of approximation. For very complex derivatives-heavy portfolios, users may opt for portfolio returns valuation using a smaller number of scenarios. Bloomberg makes this “full valuation” tail risk model within their Multi Asset Risk System (Mars).
Mac3 uses no forms of artificial intelligence, including machine learning, in favor of optimization, or mathematical formulas based on statistical analyses, for the best chance at explainability.
“You could use machine learning to create an optimized portfolio approach. We haven’t tried that yet because it’s more difficult to control machine-learning algorithms and what they do—it gives us a little bit of pause. I will not say that we will not go there; we will go there,” Lazanas says. “You could use machine learning for risk modeling. That’s, again, something we haven’t tried yet, but we will eventually.”
As an example of what optimization looks like in practice, Lazanas describes a pension fund that recently requested help in maximizing its ESG exposure—a hot topic, but much easier said than done. “Here’s the situation: If you try to create a portfolio that loads on good ESG companies, by the nature of the ESG companies, you’re also loading on other risk factors,” Lazanas says.
In equities, good ESG companies are usually larger in size, lower in volatility, and better in quality—these attributes are historically seen in ESG data, Lazanas says. But a manager, perhaps at a hedge fund, may like the prospect of high quality and low volatility, but large size is historically associated with lower returns. At the same time, the manager may be concerned with taking an increase in tracking error, the deviation between price behavior of a portfolio and a given benchmark, or controlling turnover.
“Controlling turnover heuristically is very difficult at the same time as trying to maximize your ESG and minimize tracking error. Once the portfolio construction method becomes very complicated, then it’s almost impossible to solve without a complicated mathematical model,” Lazanas says.
Optimization itself can be opaque too, but it lends itself more easily to controls than forms of AI by applying structure of risk constraints related to sectors, indexes, and other parameters.
Objects in model are closer than they appear
Lazanas says his team learned a few things from the Covid-19 pandemic that went into the making of the Mac3 model. One was the new relationships that were uncovered, such as the correlation between pharmaceuticals and technology, and the realization that some of those correlations were extremely high.
“I would say that during Covid, there was a Covid factor that emerged. No model had that factor. The same thing happened in 1999 during the dot-com emergence: nobody had the software factor in their models, but that emerged. So what happens? You can try to look at the structure of the economy and say, ‘This is a new economy that requires a new set of factors.’ And people do it, and we are going to do it,” Lazanas says. “But I think you need a more systematic approach because the economy will keep evolving, and you will always be following the economy.”
This systematic approach Bloomberg sought resulted in a heavy focus on “responsiveness,” or the ability to read the market “super-fast.” It was also the hardest to achieve. The more responsive you hope to be, the less data—only the most recent—you want to include. Though it seems counterintuitive at first, it makes sense: more historical data will make a model correct on average, but it won’t make it correct today. However, with less data, much more noise is introduced into the model’s forecasts. Reducing noise while using as little data as possible required the most advanced statistical methods Lazanas and his research team had in their arsenal—in other words, a lot of math.
As the research team continues to build out the model, it will continue to look for factors previously unrealized, and judge them transient (like Covid-19) or has having staying power (like software). A governance process will review emerging trends for consideration on whether to add them to the model on forward basis.
“Most traditional models are usually a blend of the very responsive and the long-term. And you are correct neither short-term nor long-term.” Lazanas says. “If you have a risk model with constant risk like 18%, on average over 20 years, it’s correct. The average volatility is 18%, but you’re never correct. That’s the danger of being correct on average.”
Only users who have a paid subscription or are part of a corporate subscription are able to print or copy content.
To access these options, along with all other subscription benefits, please contact info@waterstechnology.com or view our subscription options here: http://subscriptions.waterstechnology.com/subscribe
You are currently unable to print this content. Please contact info@waterstechnology.com to find out more.
You are currently unable to copy this content. Please contact info@waterstechnology.com to find out more.
Copyright Infopro Digital Limited. All rights reserved.
As outlined in our terms and conditions, https://www.infopro-digital.com/terms-and-conditions/subscriptions/ (point 2.4), printing is limited to a single copy.
If you would like to purchase additional rights please email info@waterstechnology.com
Copyright Infopro Digital Limited. All rights reserved.
You may share this content using our article tools. As outlined in our terms and conditions, https://www.infopro-digital.com/terms-and-conditions/subscriptions/ (clause 2.4), an Authorised User may only make one copy of the materials for their own personal use. You must also comply with the restrictions in clause 2.5.
If you would like to purchase additional rights please email info@waterstechnology.com
More on Emerging Technologies
This Week: Startup Skyfire launches payment network for AI agents; State Street; SteelEye and more
A summary of the latest financial technology news.
Waters Wavelength Podcast: Standard Chartered’s Brian O’Neill
Brian O’Neill from Standard Chartered joins the podcast to discuss cloud strategy, costs, and resiliency.
SS&C builds data mesh to unite acquired platforms
The vendor is using GenAI and APIs as part of the ongoing project.
Chevron’s absence leaves questions for elusive AI regulation in US
The US Supreme Court’s decision to overturn the Chevron deference presents unique considerations for potential AI rules.
Reading the bones: Citi, BNY, Morgan Stanley invest in AI, alt data, & private markets
Investment arms at large US banks are taken with emerging technologies such as generative AI, alternative and unstructured data, and private markets as they look to partner with, acquire, and invest in leading startups.
Startup helps buy-side firms retain ‘control’ over analytics
ExeQution Analytics provides a structured and flexible analytics framework based on the q programming language that can be integrated with kdb+ platforms.
The IMD Wrap: With Bloomberg’s headset app, you’ll never look at data the same way again
Max recently wrote about new developments being added to Bloomberg Pro for Vision. Today he gives a more personal perspective on the new technology.
LSEG unveils Workspace Teams, other products of Microsoft deal
The exchange revealed new developments in the ongoing Workspace/Teams collaboration as it works with Big Tech to improve trader workflows.