Bloomberg Enlists NLG to Write News Summaries
The data provider is using natural language generation to summarize news articles and write automated stories.
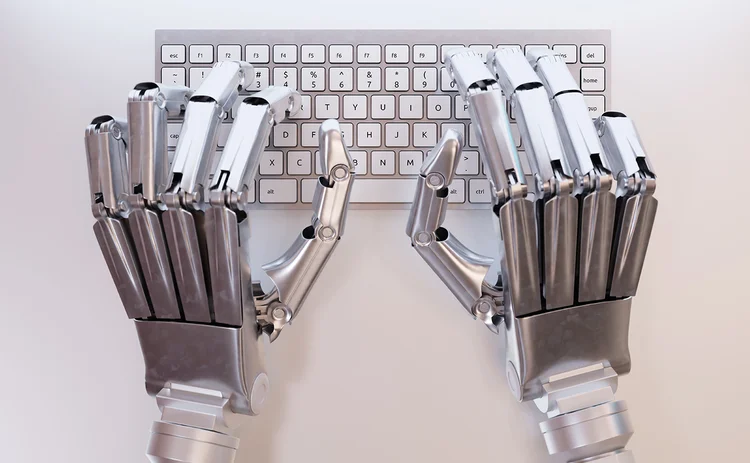
This line of text you are reading was written by a human. That might seem like an odd statement, but not at a time when natural language generation (NLG) has been making inroads in financial services and shaping how some firms are disseminating information.
Bloomberg has been using NLG for several years to generate automated articles, but in the last two years, it has turned its attention to building out a summarization tool. The offering uses machine learning to cluster thousands of articles based on a topic, and using NLG it produces a label that summarizes them. The firm’s NLG is built on a proprietary ontology, often known as a knowledge graph, which organizes all of the domain-specific information needed to construct human-readable text.
Ted Merz, global head of news products at Bloomberg, says the objective of the technology is to help data consumers—such as portfolio managers and traders—assimilate large volumes of news.
“We believe that this kind of technology allows people to very quickly absorb the major themes of the day, about a country, a company, or really any topic; whereas, if they just did a traditional search and scanned the headlines, it’s very hard to absorb that information in an expeditious manner.”
To put it into context, Merz says the Bloomberg system carries 1.5 million finance stories a day from various media outlets, but within that, there could be thousands of articles written about any one topic. For instance, there are on average 2,000 stories written about Apple on a given day and roughly 10,000 stories are written about UK interests daily, though those numbers can fluctuate during, say, earnings season or an election. During the time of this conversation, Merz ran a search to find that 428 articles had been published about Facebook by midday in the US.
Portfolio managers are responsible for monitoring hundreds of entities and staying informed about the multiple news events that could impact the performance of their portfolios. While analysts and sales traders are there to brief the front office on such events, Merz says the summarization tool automates much of the same work, as it surfaces the main information about the day’s events and omits any sensational language found in some news articles.
However, there are some challenges to consider when working with NLG and the most pressing one is ensuring data quality—in other words, making sure that the automated summaries are an accurate reflection of the news clusters. For instance, Merz says one of the biggest decisions that the development team had to make was to agree on a maximum length for the summary. The team found that if the length was too short, it did not make sense; if it was too long, it was no longer that useful as a summary.
“If you said the length was 10 characters, 30 characters, or 60 characters, you would get a different summarization every time. It is not like you just add characters; the computer recalculates (or recalibrates) the summary completely differently,” he says.
To explain this further, he uses Google Maps as an example. If someone is using Google Maps while driving, and they take a wrong turn, the map application will reroute the journey. The same concept applies to the summarization tool, where the NLG would have to adjust to different length specifications to summarize the text. In the end, the firm decided on a maximum of 50 characters per summary.
Like any machine-learning model, it works best on data it has already seen. For this reason, Merz says there is always a need for continuous training as new and unprecedented events emerge, such as the pandemic. So while the new summaries tied to Covid-19 may not have been perfect during the outbreak in March, Merz says, they improve over time as the model ingests more and more annotations and Covid-19 news examples.
Bloomberg also staffs a quality control (QC) team tasked with reviewing the news summaries and providing feedback to the training model. Using an internal interface, the QCs can train the model by giving summaries a thumbs up, a thumbs down, or killing the summary altogether.
News Bites
Bloomberg is also using NLG to generate five types of news articles: stories compiled from alternative data sources, corporate filings, combined datasets (a mixture of traditional and alternative data), press releases, and market anomalies.
The articles in the first group are generated from “obscure alt data sets” as Merz calls them, such as the number of people riding the subway, the number of people working in an office, or the number of restaurant bookings via applications such as OpenTable. Merz says that because traders or portfolio managers are less familiar with these types of datasets, turning them into articles makes them easier to understand.
“If you were only looking at the dataset from OpenTable about restaurant bookings, it would be hard to understand what was happening. So what we do is write scripts [or articles] that say, ‘The number of restaurant bookings is up or down versus comparable periods; these are the areas and places where they’re increasing or decreasing the most,’ and it really makes that data much faster and easier to absorb.”
The news articles from the second group summarize large datasets that include corporate filings, such as earnings calls or filings with the US Securities and Exchange Commission (SEC). Merz says the automated news tool combs through the data, compares it with previous filings, extrapolates trends in the data, and then, using the NLG turns that information into short news articles in seconds.
The third group of articles is generated from multiple datasets. For instance, when a hurricane is forming, the news tool pulls in data including weather and geolocation information, to predict what company assets are in danger of being impacted. The solution is based on a set of language rules and thresholds, which dictate when a story should be published. To illustrate this further, Merz says that in the third group of news articles, if a hurricane were to hit 25% of Exxon’s oil facilities, that risk percentage would be enough to trigger the system to publish a story. The logic behind this is to avoid spamming traders or portfolio managers with meaningless information.
Bloomberg is also generating news stories from press releases and market anomalies, such as a significant drop in stock prices or if the options market fluctuates. The firm recently released a whitepaper where it found that monitoring market anomalies and the number of automated articles written about a specific company could help predict a corporate action, such as a merger or acquisition.
“These small market anomalies could be occurring before a major corporate event and it’s sometimes hard for a person to put those together and understand that it’s a big deal. But if you see multiple [market anomalies] occurring, then you can understand that is a situation to watch,” Merz says.
Further reading
Only users who have a paid subscription or are part of a corporate subscription are able to print or copy content.
To access these options, along with all other subscription benefits, please contact info@waterstechnology.com or view our subscription options here: http://subscriptions.waterstechnology.com/subscribe
You are currently unable to print this content. Please contact info@waterstechnology.com to find out more.
You are currently unable to copy this content. Please contact info@waterstechnology.com to find out more.
Copyright Infopro Digital Limited. All rights reserved.
As outlined in our terms and conditions, https://www.infopro-digital.com/terms-and-conditions/subscriptions/ (point 2.4), printing is limited to a single copy.
If you would like to purchase additional rights please email info@waterstechnology.com
Copyright Infopro Digital Limited. All rights reserved.
You may share this content using our article tools. As outlined in our terms and conditions, https://www.infopro-digital.com/terms-and-conditions/subscriptions/ (clause 2.4), an Authorised User may only make one copy of the materials for their own personal use. You must also comply with the restrictions in clause 2.5.
If you would like to purchase additional rights please email info@waterstechnology.com
More on Data Management
New working group to create open framework for managing rising market data costs
Substantive Research is putting together a working group of market data-consuming firms with the aim of crafting quantitative metrics for market data cost avoidance.
Off-channel messaging (and regulators) still a massive headache for banks
Waters Wrap: Anthony wonders why US regulators are waging a war using fines, while European regulators have chosen a less draconian path.
Back to basics: Data management woes continue for the buy side
Data management platform Fencore helps investment managers resolve symptoms of not having a central data layer.
‘Feature, not a bug’: Bloomberg makes the case for Figi
Bloomberg created the Figi identifier, but ceded all its rights to the Object Management Group 10 years ago. Here, Bloomberg’s Richard Robinson and Steve Meizanis write to dispel what they believe to be misconceptions about Figi and the FDTA.
SS&C builds data mesh to unite acquired platforms
The vendor is using GenAI and APIs as part of the ongoing project.
Aussie asset managers struggle to meet ‘bank-like’ collateral, margin obligations
New margin and collateral requirements imposed by UMR and its regulator, Apra, are forcing buy-side firms to find tools to help.
Where have all the exchange platform providers gone?
The IMD Wrap: Running an exchange is a profitable business. The margins on market data sales alone can be staggering. And since every exchange needs a reliable and efficient exchange technology stack, Max asks why more vendors aren’t diving into this space.
Reading the bones: Citi, BNY, Morgan Stanley invest in AI, alt data, & private markets
Investment arms at large US banks are taken with emerging technologies such as generative AI, alternative and unstructured data, and private markets as they look to partner with, acquire, and invest in leading startups.