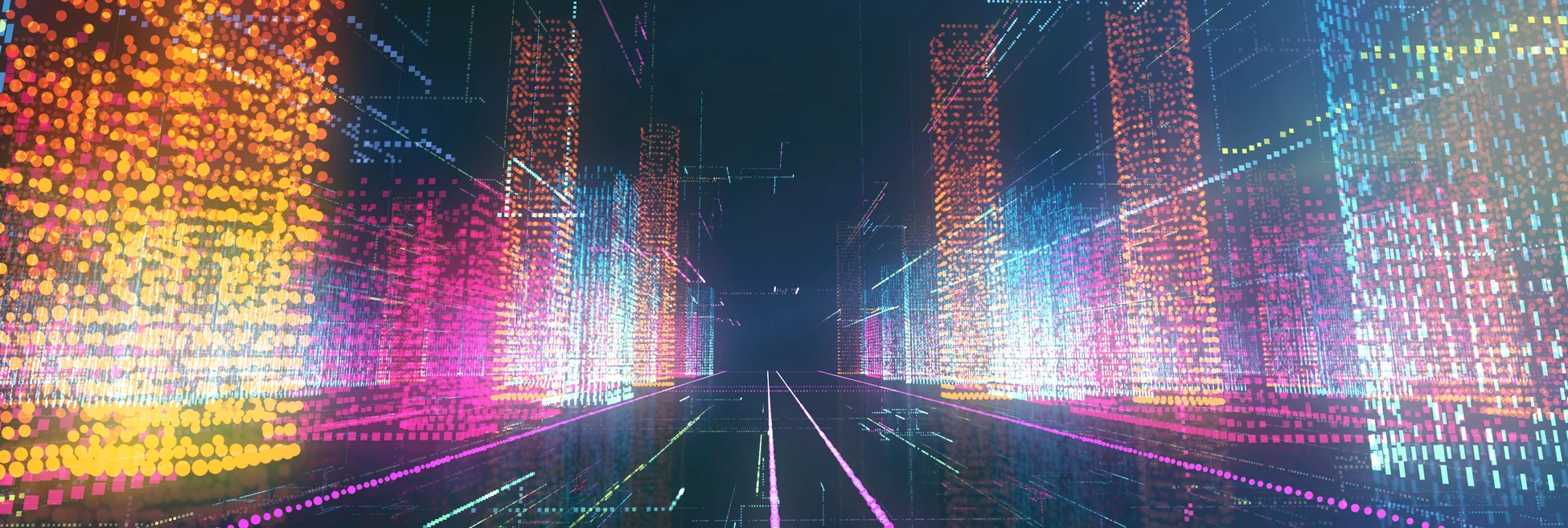
BNP Paribas AM Combines NLP, ML for Sentiment Indicators in News Data
The asset manager is teaming with a vendor on the project, which will first be used for equities trading before moving to corporate bonds.
BNP Paribas Asset Management plans to go live this year with an NLP-based model that finds sentiment indicators in news reports to forecast company returns.
“We are not yet big users of text and NLP, but this is an area we have been developing recently, and where we expect to deploy some models,” says Raul Leote de Carvalho, deputy head of BNP Paribas Asset Management’s Quantitative Research Group.
The NLP-based sentiment indicators “should go into production very soon,” he adds.
The model represents the confluence of two powerful trends: widespread availability and easy access to unstructured text data, such as news reports; and major advancements in natural language processing (NLP), which are revolutionizing the field of text research.
But while the cloud, machine learning, and other automation tools have made it easier and less costly to collect, store, and analyze text data, the exercise is still extremely time-consuming and riddled with complexities, such as licensing requirements. So BNP Paribas is relying on an external data provider with the necessary licenses to scrape reputable online news outlets. The vendor then structures the data, removing unnecessary words and producing a numerical signal from the material information.
BNP Paribas uses the structured data to construct its models, selecting only the information that is necessary to build long-term investment signals. Within the model, the news is classified by different topics that cover various aspects of a company’s operations and activities.
“We choose the topics we want to rely on to decide if a company is worth investing in or not, based on whether the sentiment about the company is positive or negative,” Leote de Carvalho says. “The key question is, ‘What are the topics that we should use in order to build this model?’ For this model, that is what we are doing. [Additionally], we plan to build other models for other approaches.”
He says the asset manager has been careful not to overfitt the data, which is a general problem in machine learning and quant modeling, and becomes a bigger issue with more complex models.
“If you [are] just overfitting the data by having too many degrees of freedom in your model, that serves no purpose. It is guaranteed that the backtest is beautiful, but in the future [it won’t provide] added value,” he says. “That is one of the big difficulties of just using machine learning lightly when you are applying it to asset management. You have to be extremely careful with it.”
The current focus of the project, which BNP began working on in early 2018, is on finding sentiment signals for equities, but the firm plans to also look at corporate bonds in the future.
BNP Paribas already uses various machine learning techniques for a variety of applications, including to build risk models.
Leote de Carvalho says machine learning has been particularly useful for conducting and improving upon principal component analysis (PCA), a technique that reduces the variables in a dataset to bring out more vivid patterns. This can be useful constructing covariance matrixes, which measures the relationship between assets in a portfolio.
To calculate the expected volatility of a portfolio, a risk manager must first determine expected volatility of each stock and then account for the diversification effect of buying many stocks, which requires a correlation matrix.
“If you put the volatility and correlation matrix together, you get the variance/covariance matrix, and that is a key question of risk modeling in equities: what is the best variance/covariance matrix you can come up with,” Leote de Carvalho says.
Then BNP separates the two parts. It uses econometric models for forecasting stock volatility, and for the correlation matrix it uses approaches such as PCA.
Leote de Carvalho says that using PCA can reduce the matrix into “a much smaller matrix with just a few risk factors, and then it just tells you how each stock is exposed to the factors, and the number of factors—and how that reduction in dimensionality is done is unsupervised.”
This particular model has allowed BNP Paribas to improve its variance/covariance matrixes, he says:
“If you do it well, the modeling of the correlations using principal component analysis is quite powerful for diversified portfolios.”
- Quants Use Nowcasting As Covid Crystal Ball: Experts from UBS, Unigestion, MIT and QuantConnect discuss the need for nowcasting, and what the alt data boom has made possible in trying to navigate today’s crisis. Click here to read more.
The model takes stock return time-series as an input and outputs a correlation matrix with a smaller number of key factors.
Leote de Carvalho says that usually when there are a large number of stocks being looked at for portfolio construction, there is a problem of dimensionality. “The question is, how do you tackle this problem, and how can you estimate a correlation matrix in a way that can be used to—as accurately as possible—then calculate the expected tracking volatility of your portfolio? We found that these types of unsupervized models—which fall into the factor analysis-type model, like PCA and similar algos or models—tend to do a good job.”
The Quant Research Group was created in 2017 with the aim of bringing together all the quants that previously were scattered around the organization. Leote de Carvalho says the aim was to get the quants on the same page to tackle similar problems across the entire organization.
“Increasingly, quant goes into all investment processes, and there is no point in having different people thinking and developing exactly the same models,” he says.
Further reading
Only users who have a paid subscription or are part of a corporate subscription are able to print or copy content.
To access these options, along with all other subscription benefits, please contact info@waterstechnology.com or view our subscription options here: http://subscriptions.waterstechnology.com/subscribe
You are currently unable to print this content. Please contact info@waterstechnology.com to find out more.
You are currently unable to copy this content. Please contact info@waterstechnology.com to find out more.
Copyright Infopro Digital Limited. All rights reserved.
As outlined in our terms and conditions, https://www.infopro-digital.com/terms-and-conditions/subscriptions/ (point 2.4), printing is limited to a single copy.
If you would like to purchase additional rights please email info@waterstechnology.com
Copyright Infopro Digital Limited. All rights reserved.
You may share this content using our article tools. As outlined in our terms and conditions, https://www.infopro-digital.com/terms-and-conditions/subscriptions/ (clause 2.4), an Authorised User may only make one copy of the materials for their own personal use. You must also comply with the restrictions in clause 2.5.
If you would like to purchase additional rights please email info@waterstechnology.com
More on Data Management
New working group to create open framework for managing rising market data costs
Substantive Research is putting together a working group of market data-consuming firms with the aim of crafting quantitative metrics for market data cost avoidance.
Off-channel messaging (and regulators) still a massive headache for banks
Waters Wrap: Anthony wonders why US regulators are waging a war using fines, while European regulators have chosen a less draconian path.
Back to basics: Data management woes continue for the buy side
Data management platform Fencore helps investment managers resolve symptoms of not having a central data layer.
‘Feature, not a bug’: Bloomberg makes the case for Figi
Bloomberg created the Figi identifier, but ceded all its rights to the Object Management Group 10 years ago. Here, Bloomberg’s Richard Robinson and Steve Meizanis write to dispel what they believe to be misconceptions about Figi and the FDTA.
SS&C builds data mesh to unite acquired platforms
The vendor is using GenAI and APIs as part of the ongoing project.
Aussie asset managers struggle to meet ‘bank-like’ collateral, margin obligations
New margin and collateral requirements imposed by UMR and its regulator, Apra, are forcing buy-side firms to find tools to help.
Where have all the exchange platform providers gone?
The IMD Wrap: Running an exchange is a profitable business. The margins on market data sales alone can be staggering. And since every exchange needs a reliable and efficient exchange technology stack, Max asks why more vendors aren’t diving into this space.
Reading the bones: Citi, BNY, Morgan Stanley invest in AI, alt data, & private markets
Investment arms at large US banks are taken with emerging technologies such as generative AI, alternative and unstructured data, and private markets as they look to partner with, acquire, and invest in leading startups.