Brown Brothers Harriman Experiments with Machine Learning
The firm is developing machine learning models internally to optimize the reconciliation process and detect price anomalies.
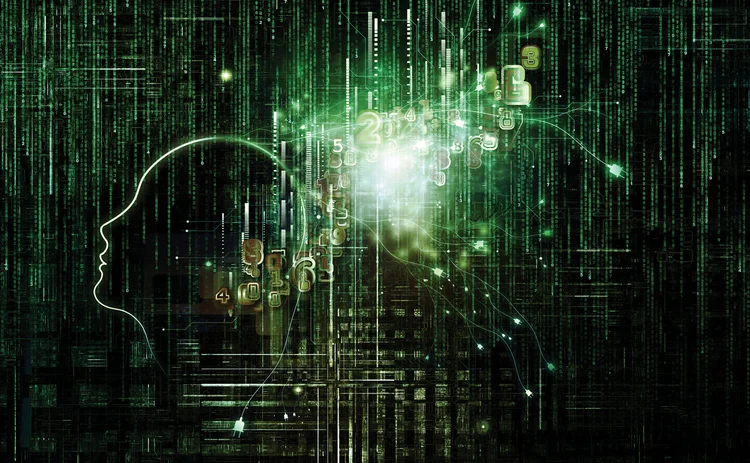
Brown Brothers Harriman (BBH), which services more than $5 trillion of assets, is developing its own machine learning models to detect price anomalies and eliminate manual processes involved in reconciliation, Michael McGovern, managing director and head of the firm’s investor services fintech offering, said while speaking on a panel at the North American Financial Information Summit (NAFIS).
Like many financial services firms, BBH is testing the machine learning waters and has found that a natural area for adoption is in the reconciliations realm. BBH’s trained and supervised model pulls in client data and combines old-school statistics with new forms of AI to “provide a signal and separate it from noise, and then deploy that as an input into a reconciliation process,” he said.
- To read about how BNY Mellon is using machine learning to make access to—and understanding of—its documents easier for its employees, click here.
As an example, machine learning helps bring efficiency to one of the products under BBH’s Infomediary franchise, called InfoRecon, which makes use of a back-end reconciliation engine. In working with one client, the data coming in was ideal, according to McGovern—clean, adequately governed, high quality. Yet, the match rate hovered around 91%. By using machine learning algorithms to better find and highlight breaks, that figure reached 98.4% by identifying additional unmatched items.
Perhaps even more surprising, the model was trained entirely over a single weekend.
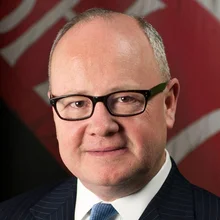
“If you think about it, the difference between 91 and 100 is all the manual processing left in that particular process. With this one model, we’ve eliminated 90% of that,” he said.
Discounting the labor involved in just collecting a massive amount of data, McGovern said the line between opportunity and challenge is a little bit blurred in regards to democratizing machine learning and integrating seamlessly across the industry. The key is to get the people equation right, first.
“Get the tools in front of the people that have the domain expertise and have analytic mindsets and expertise in a specific area—could be an asset class, could be an investment strategy, could be geography, could be some arcane form of risk,” he said. “This is not my term, but I love it, so I plagiarized it: ‘I think we need to create a generation of citizen data scientists, and enable the analysts we have in different parts of our business to utilize this toolset to generate the next set of insights.’”
- To read about how Zurich Insurance is trying out a Data-as-a-Service (DaaS) model, in conjunction with AIM Software, to be able to direct its energies toward high-level analytics and artificial intelligence, click here.
McGovern also noted that the firm has started using machine learning to detect price anomalies, but did not provide further detail.
Further reading
Only users who have a paid subscription or are part of a corporate subscription are able to print or copy content.
To access these options, along with all other subscription benefits, please contact info@waterstechnology.com or view our subscription options here: http://subscriptions.waterstechnology.com/subscribe
You are currently unable to print this content. Please contact info@waterstechnology.com to find out more.
You are currently unable to copy this content. Please contact info@waterstechnology.com to find out more.
Copyright Infopro Digital Limited. All rights reserved.
As outlined in our terms and conditions, https://www.infopro-digital.com/terms-and-conditions/subscriptions/ (point 2.4), printing is limited to a single copy.
If you would like to purchase additional rights please email info@waterstechnology.com
Copyright Infopro Digital Limited. All rights reserved.
You may share this content using our article tools. As outlined in our terms and conditions, https://www.infopro-digital.com/terms-and-conditions/subscriptions/ (clause 2.4), an Authorised User may only make one copy of the materials for their own personal use. You must also comply with the restrictions in clause 2.5.
If you would like to purchase additional rights please email info@waterstechnology.com
More on Data Management
New working group to create open framework for managing rising market data costs
Substantive Research is putting together a working group of market data-consuming firms with the aim of crafting quantitative metrics for market data cost avoidance.
Off-channel messaging (and regulators) still a massive headache for banks
Waters Wrap: Anthony wonders why US regulators are waging a war using fines, while European regulators have chosen a less draconian path.
Back to basics: Data management woes continue for the buy side
Data management platform Fencore helps investment managers resolve symptoms of not having a central data layer.
‘Feature, not a bug’: Bloomberg makes the case for Figi
Bloomberg created the Figi identifier, but ceded all its rights to the Object Management Group 10 years ago. Here, Bloomberg’s Richard Robinson and Steve Meizanis write to dispel what they believe to be misconceptions about Figi and the FDTA.
SS&C builds data mesh to unite acquired platforms
The vendor is using GenAI and APIs as part of the ongoing project.
Aussie asset managers struggle to meet ‘bank-like’ collateral, margin obligations
New margin and collateral requirements imposed by UMR and its regulator, Apra, are forcing buy-side firms to find tools to help.
Where have all the exchange platform providers gone?
The IMD Wrap: Running an exchange is a profitable business. The margins on market data sales alone can be staggering. And since every exchange needs a reliable and efficient exchange technology stack, Max asks why more vendors aren’t diving into this space.
Reading the bones: Citi, BNY, Morgan Stanley invest in AI, alt data, & private markets
Investment arms at large US banks are taken with emerging technologies such as generative AI, alternative and unstructured data, and private markets as they look to partner with, acquire, and invest in leading startups.