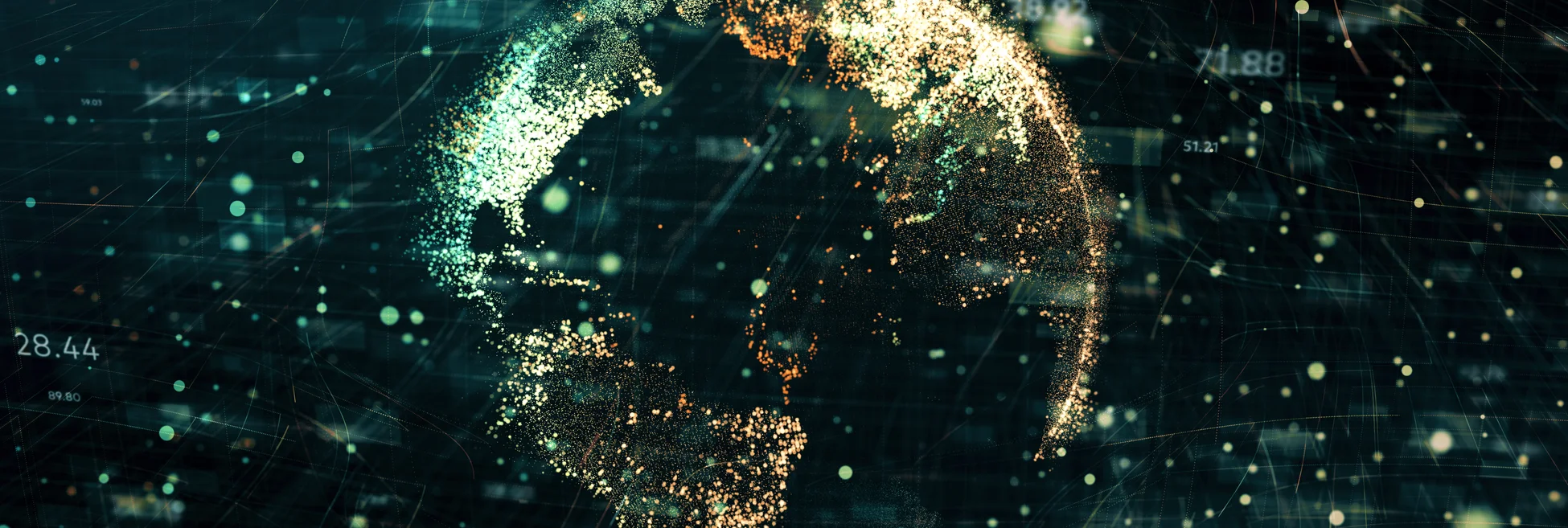
Brown Brothers Harriman Re-Imagines AI Ecosystem After March Market Madness
Unprecedented volatility in March is leading the bank to double down on its AI systems in a big way.
On March 9, as the S&P 500 slid nearly 8% amid coronavirus panic, market participants endured whiplash, including Kevin Welch, managing director of investor services at New York-based Brown Brothers Harriman.
Welch and his team had a very small window at the end of the day to strike a net asset value (NAV) for their clients, even as the number of price anomalies detected soared. With the help of supervised machine learning models that were put into place a few years ago, they were able to do it, but not without readjustments. And for all the trauma March brought, Welch is hopeful it can inspire new ways of thinking about artificial intelligence at the bank.
Before the implementation of the Anomaly NAV Tracking System (ANTS), built upon machine-learning algorithms, the analysts on BBH’s fund accounting team had to review 10,000 or more securities each day that broke a traditional pricing threshold. With the system, they reduced the number of true anomalies to fewer than 500, Welch said in December, speaking at Waters USA.
The process has opened up a new avenue that Welch is eager to explore: multi-model environments that range between two and five models…where, on a day of heightened volatility like March 9, the business can watch and evaluate the models’ performances side-by-side in real time and adjust where appropriate.
If a day like March 9 had occurred before the ANTS project, Welch estimates that 30% of the securities it tracks would have required manual validation.
“Think about if you had a million securities you were pricing on a daily basis; you’re now talking about 300,000 that someone would have to review,” Welch said. “That would have been challenging if everybody was in the office [and] incredibly challenging in a remote environment.”
In reality, the analysts had to manually review about 3% of price movements, but they didn’t stop there. The team watched securities follow a new pattern in real-time—prices were moving by 5% on average, up from a previous month when 3% was the record—and reduced the amount of manual reviews to 1% in the span of a week.
“When you think about AI, it takes a lot of models even to get to the one that’s most effective for us. So in the scenario we talked about where the movement went from 3% to 1% exception, there were actually nine models that our data scientists came up with, and then we narrowed it down to the one the business thought was most appropriate to employ,” Welch says.
The model was fed back to the business users, who reviewed adjustments to the algorithms to ensure they would reduce exceptions safely and sustainably, then implemented them.
The process has opened up a new avenue that Welch is eager to explore: multi-model environments that range between two and five models—but probably no more than three at first—where, on a day of heightened volatility like March 9, the business can watch and evaluate the models’ performances side-by-side in real time and adjust where appropriate.
“In this potential new future, it would actually be within the application that business users would have two or three pre-approved models that they validated and tested, and then they would be able to toggle and shift through those models based on what they were seeing on a day-to-day basis,” Welch says.
No model is forever, and will always require updates and adjustments as outside events unfold. Though they automatically improved based on the addition of more historical data, Welch says it’s interesting to think about how humans can get ahead of them and future-proof against what hasn’t yet been experienced. It’s relatively easy to program a model with hundreds or thousands of possible scenarios—including ones that haven’t happened yet—but it would lack data that prescribes what actions systems would have or should have taken. That’s not to say it can’t be done.
For a reconciliations application called Linc, the bank retrained the algorithm by feeding through thousands of scenarios, and pre-programming how the system should react in each of them—though that’s likely easier to do for accounting than for world events such as pandemics and natural disasters.
“You have to think in advance: What’s the condition that I’m trying to solve for, and what is the action that I want the machine to take?” Welch says. “And then if you can answer both of those questions, it’s very easy to think ahead and train the machine to do that. I think the challenge is in our own imaginations—all the different scenarios that can happen.”
Further reading
Only users who have a paid subscription or are part of a corporate subscription are able to print or copy content.
To access these options, along with all other subscription benefits, please contact info@waterstechnology.com or view our subscription options here: http://subscriptions.waterstechnology.com/subscribe
You are currently unable to print this content. Please contact info@waterstechnology.com to find out more.
You are currently unable to copy this content. Please contact info@waterstechnology.com to find out more.
Copyright Infopro Digital Limited. All rights reserved.
As outlined in our terms and conditions, https://www.infopro-digital.com/terms-and-conditions/subscriptions/ (point 2.4), printing is limited to a single copy.
If you would like to purchase additional rights please email info@waterstechnology.com
Copyright Infopro Digital Limited. All rights reserved.
You may share this content using our article tools. As outlined in our terms and conditions, https://www.infopro-digital.com/terms-and-conditions/subscriptions/ (clause 2.4), an Authorised User may only make one copy of the materials for their own personal use. You must also comply with the restrictions in clause 2.5.
If you would like to purchase additional rights please email info@waterstechnology.com
More on Trading Tech
Recent volatility highlights tech’s vital role in fixed income pricing
MarketAxess’ Julien Alexandre discusses how cutting-edge technology is transforming pricing and execution in the fixed income market amid periodic bouts of volatility
Banks fret over vendor contracts as Dora deadline looms
Thousands of vendor contracts will need repapering to comply with EU’s new digital resilience rules
Where have all the exchange platform providers gone?
The IMD Wrap: Running an exchange is a profitable business. The margins on market data sales alone can be staggering. And since every exchange needs a reliable and efficient exchange technology stack, Max asks why more vendors aren’t diving into this space.
This Week: Trading Technologies completes ANS deal; State Street; Equinix; and more
A summary of the latest financial technology news.
Interactive Brokers looks beyond US borders for growth opportunities
As retail trading has grown in volume and importance, Interactive Brokers and others are expanding international offerings and marketing abroad.
JP Morgan’s goal of STP in loans materializes on Versana’s platform
The accomplishment highlights the budding digitization of private credit, though it’s still a long road ahead.
As data volumes explode, expect more outages
Waters Wrap: At least for those unprepared—though preparation is no easy task—says Anthony.
This Week: ICE Bonds and MarketAxess plan to connect liquidity networks, TS Imagine, Bloomberg, and more
A summary of the latest financial technology news.