Brown Brothers Harriman To 'Transform' Its Fund Accounting Service by 2021
The bank is in the throes of a hefty transformation project within its Investor Services division, which began with machine-learning efforts last year.
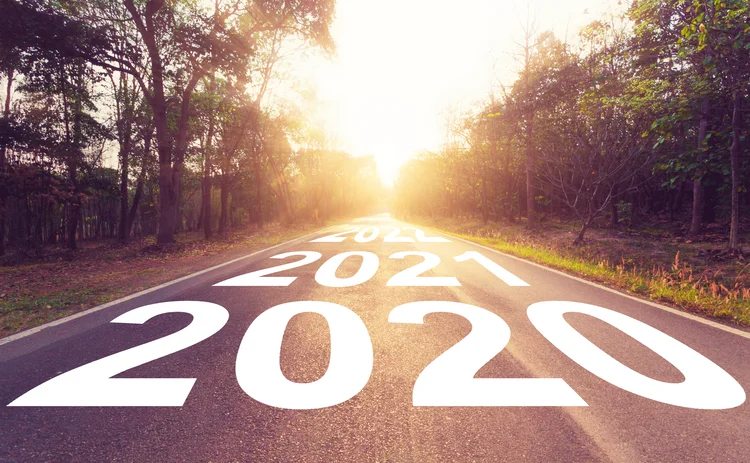
By 2021, Brown Brothers Harriman’s (BBH) hopes to have radically transformed its fund accounting process end-to-end. At the core of this movement is AI, including machine learning and natural language generation (NLG).
Starting last year, BBH began incorporating supervised machine learning in order to strike net asset values (NAVs) for clients. The algorithms replaced hundreds of manual-work hours by analysts, who would sift through thousands of pricing anomalies, or exceptions, finding that as low as 1% were true exceptions. Through its machine learning-powered Anomaly NAV Tracking System (ANTS), the bank has eliminated 90% of false positives.
The next big venture began in Q4 of 2019 and will be implemented by the end of Q2 this year. The system, dubbed Guardrail, expands upon ANTS and entails looking at the overall portfolio of a fund against a benchmark, such as the S&P 500 or another index, and making sure there is not dramatic price movements from a client’s fund versus the desired benchmark. Clients will be able to receive custom reports based on these comparisons.
One of the benefits of Guardrail, says Michael Cook, vice president of investor services, is the time saved by BBH’s analysts who, if there is such a deviation, currently have to dig through all the components of a NAV to determine why it deviated, and write up an analysis.
“2021 is where all these AI projects we’re doing in fund accounting come together,” Cook says. “ANTS right now is [about] security pricing, but as we build out for the AI to review interest, and to do standardization, and to go through all these journal entries to be able to summarize and provide an analysis at the end of the day, we think that’s going to be transformational.”
The firm is also leaning on NLG technology in order to lessen analysts’ reliance on Excel spreadsheets to make decisions. After taking in all of the information within their spreadsheets—the bank has already developed its natural-language processing capabilities—the bank is building a platform that will be able to automatically generate language that says definitively, for example, “Yes, your Class A share diverged [due to an expense posting, for example],” says Kevin Welch, managing director of investor services for BBH.
Finally, the institution is also looking to develop an algo-validation tool that can help clients to more easily verify their own proofs-of-concept.
“Something like 70% of AI algorithms that are being built never go into production,” Welch says. “If BBH has built something and you’ve got a hypothesis, we have the opportunity to let [the client] leverage that to make sure it’s a good investment for them before they move forward, or they can make the decision that maybe it’s even something they want to use daily in our algorithm as well.”
The tool has been in development since July. While the service has not had client uptake yet, the bank has had preliminary talks with some interested parties.
BOX: In The Matrix
Despite the industry-wide push for innovation, regulatory concerns can hinder development. When machines are making decisions for humans, explainability is crucial. That’s where a confusion matrix comes in. Though not new nor an exclusive method—it’s used in most, if not all, machine-learning endeavors—Welch says that they are crucial in providing a visual into how these algorithms perform. Being that many new and sophisticated machine-learning algos are essentially black boxes, a confusion matrix can provide explainability, management and oversight, and the ability to measure the machine’s effectiveness.
“I think for BBH’s risk profile, and I think for the industry’s risk profile, we need to focus on making sure our solutions are glass boxes—where we can sit down with a regulator, an auditor, or one of our internal control groups, and we can show them how the machine is making the decision,” Welch says. “In order for me to do that, I need to understand things like the confusion matrix because that’s going to be a proof point when I’m showing that.”
On the matrix’s X-axis are predicted results. One column shows a “yes” prediction, while the other shows a “no” prediction. The Y-axis shows actual results—an actual “no” and an actual “yes.” Ideally, the values in the quadrants should match up. Welch notes that whether you begin with test data, or in the production phase, it’s vital to have a dataset of actual value to go against the prediction.
For ANTS, Welch and his team created a user interface that could review all the exceptions flagged by the algorithm and validate them. That dataset—the users performed about 40,000 of those reviews—was the dataset used to train the model.
What Welch and BBH’s data scientists have done within their production environment is added a dashboard with a confusion matrix right on it. The team can use the dashboard and decide where to make an adjustment if there’s an unacceptable number of false positives. (It should be in the vicinity of 500, Welch says.) To that end, they’ve created a threshold where, if the analysts start to see 750 to 1,000 false positives, for example, the tech teams might need to go back into the algorithm and make an adjustment.
“I think what’s changing in the industry is the fact that you have two people from the business with you today, talking about the confusion matrix,” Welch says. “As we shift to this new model for data-driven work and data-driven transformation, you now have a group of business users, support teams, [and] control functions, that really understand how all this works. Because there’s a lot of oversight and work that we need to do, we have to understand how the machines learn.”
Further reading
Only users who have a paid subscription or are part of a corporate subscription are able to print or copy content.
To access these options, along with all other subscription benefits, please contact info@waterstechnology.com or view our subscription options here: http://subscriptions.waterstechnology.com/subscribe
You are currently unable to print this content. Please contact info@waterstechnology.com to find out more.
You are currently unable to copy this content. Please contact info@waterstechnology.com to find out more.
Copyright Infopro Digital Limited. All rights reserved.
As outlined in our terms and conditions, https://www.infopro-digital.com/terms-and-conditions/subscriptions/ (point 2.4), printing is limited to a single copy.
If you would like to purchase additional rights please email info@waterstechnology.com
Copyright Infopro Digital Limited. All rights reserved.
You may share this content using our article tools. As outlined in our terms and conditions, https://www.infopro-digital.com/terms-and-conditions/subscriptions/ (clause 2.4), an Authorised User may only make one copy of the materials for their own personal use. You must also comply with the restrictions in clause 2.5.
If you would like to purchase additional rights please email info@waterstechnology.com
More on Data Management
New working group to create open framework for managing rising market data costs
Substantive Research is putting together a working group of market data-consuming firms with the aim of crafting quantitative metrics for market data cost avoidance.
Off-channel messaging (and regulators) still a massive headache for banks
Waters Wrap: Anthony wonders why US regulators are waging a war using fines, while European regulators have chosen a less draconian path.
Back to basics: Data management woes continue for the buy side
Data management platform Fencore helps investment managers resolve symptoms of not having a central data layer.
‘Feature, not a bug’: Bloomberg makes the case for Figi
Bloomberg created the Figi identifier, but ceded all its rights to the Object Management Group 10 years ago. Here, Bloomberg’s Richard Robinson and Steve Meizanis write to dispel what they believe to be misconceptions about Figi and the FDTA.
SS&C builds data mesh to unite acquired platforms
The vendor is using GenAI and APIs as part of the ongoing project.
Aussie asset managers struggle to meet ‘bank-like’ collateral, margin obligations
New margin and collateral requirements imposed by UMR and its regulator, Apra, are forcing buy-side firms to find tools to help.
Where have all the exchange platform providers gone?
The IMD Wrap: Running an exchange is a profitable business. The margins on market data sales alone can be staggering. And since every exchange needs a reliable and efficient exchange technology stack, Max asks why more vendors aren’t diving into this space.
Reading the bones: Citi, BNY, Morgan Stanley invest in AI, alt data, & private markets
Investment arms at large US banks are taken with emerging technologies such as generative AI, alternative and unstructured data, and private markets as they look to partner with, acquire, and invest in leading startups.