Brown Brothers Harriman's Welch Explains Bank's Use of Artificial Intelligence
The bank has a number of projects using emerging technologies, one of which optimizes the process of detecting price anomalies.
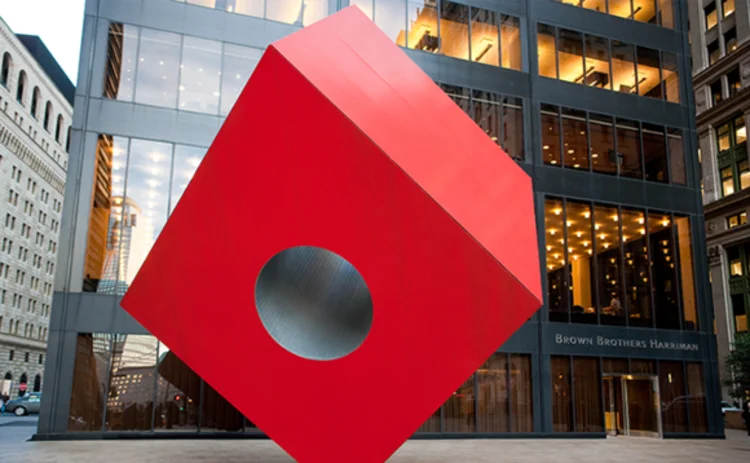
At Brown Brothers Harriman (BBH), which services more than $5 trillion of assets, several projects that use emerging technologies—namely, artificial intelligence, machine learning and robotic process automation—are underway. Kevin Welch, managing director of investor services at BBH, spoke with WatersTechnology about how the investment firm has used these technologies to tackle both internal problems and those of clients, projects in its pipeline, and a changing landscape at one of the oldest private banks in the US.
Our analysts had to review tens of thousands of exceptions that broke traditional pricing thresholds to make sure there weren’t any true net value asset (NAV) impacts. In today’s environment, using AI, we would have gotten that to under 1,000.
Kevin Welch, BBH
One area in which machine learning has found a home is detecting price anomalies, an ordinarily onerous process that requires hundreds of hours from a raft of human analysts.
Historically, the bank would track the movements of securities by a certain percentage, and if those thresholds were crossed, that would generate an exception—a rather crude way of running these checks. Analysts would sift through thousands of exceptions each night, finding “maybe 1%” of them were true exceptions, Welch says. Now, the bank uses machine learning algorithms to perform predictive analytics, saving man-hours and improving accuracy.
Welch points to Brexit as an example. “When that happened a few years ago, all securities in the market moved by probably over 7%,” Welch says. “We have hundreds of thousands of securities in our fund accounting system. Our analysts had to review tens of thousands of exceptions that broke traditional pricing thresholds to make sure there weren’t any true net value asset (NAV) impacts. In today’s environment, using AI, we would have gotten that to under 1,000.”
The algorithm is fed with historical data over several years that shows how the movement of a certain stock should correlate to all of the other securities in the bank’s fund accounting system. It then reviews all of the intra-day movements of all those securities versus one they expect to go up or down in price. If an anomaly occurs, say a stock price drops by 5% when it was expected to do the opposite, only then is it flagged for analyst review. Welch adds that having that correlation removes the need to spend a lot of time investigating normal market events, such as stock splits and responses to earnings reports.
With our use of AI, we’ve been able to reduce the number of false positives, which has meant a total reduction in the number of exceptions by 99%.
Kevin Welch
As a result, the bank’s clients are able to carry out their own duties much quicker within a very compressed timeframe.
“We’re striking a NAV for our clients every single day,” Welch says. “With our use of AI, we’ve been able to reduce the number of false positives, which has meant a total reduction in the number of exceptions by 99%. We’re able to get through the remaining 1% faster, and pass that to our clients, who have to do checks on their side earlier in the day.”
Improved Decisions
BBH currently has four projects in its pipeline in different areas of the bank, which use different forms of AI and predictive analytics. One project in development is a proof-of-concept tool, which will allow clients to anonymize their data, send it to the bank, which will feed it through their algorithm, and replicate what their results would be before the client makes an investment.
“A lot of our work is [around the] decision-making [process],” Welch says. “There are hundreds of activities like that across our organization. Where we see the opportunity to use AI is where we can assist our analysts in augmenting their decision-making thought process.”
Back in May, BBH’s Michael McGovern, managing director and head of the firm’s investor services fintech offering, spoke at the North American Financial Information Summit (NAFIS) about machine learning’s effects on reconciliations for the bank, another highly-manual process. The bank has used it to solve issues internally, but has also deployed its solution through its Infomediary franchise for clients to use in their own organizations.
That’s been a key focus in deciding how and where to implement emerging technologies, Welch says, naming three key lessons learned.
“The first one is, we’re investing in the business problem, not AI,” Welch says. “There’s a lot of hype around these techs, whether it’s DLT, AI, or RPA. … I think what we’ve done is we’ve looked at what the business problems are that we have as an organization, and where we can apply it across the board. Reconciliations are a perfect example of a common industry problem across asset managers and asset services, so by developing a machine-learning solution for that, you can scale that efficiency, and we can help our clients by embedding it in some of our products.”
When you’re talking about AI and machine learning, you’re really talking about re-thinking, re-imagining and re-designing the process.
Kevin Welch
Second, they avoid one-off, catch-all projects. When Welch and McGovern set out to optimize reconciliations, they had to approach it in phases, and the same went for pricing anomalies. The first step was to use the technology to identify anomalies and code them. Only when that was figured out could they go and confidently build and train a supervised machine-learning model to resolve anomalies on its own.
Last, they’ve had to keep employees in mind. Their specialists have spent entire careers clearing exceptions, but technology has added a whole new dimension to their jobs. Not only are they responsible for clearing a transaction, but also training an algorithm to the degree that the next time a similar transaction comes through, the machine can reliably resolve it versus the human. At the same time, the bank has brought in new and diverse talent, from recent university grads to veterans from other industries who have deployed the same technologies in different ways.
“When you’re talking about AI and machine learning, you’re really talking about re-thinking, re-imagining and re-designing the process,” Welch says. “And to do that, you’ve got to bring in some fresh ideas and mindsets into your organization and team.”
Of course, implementing and deploying a project is far from the end of the road. One tool that helps ensure the bank’s solutions are being utilized effectively and working as they should is the confusion matrix, a four-box quadrant that tells what the machine predicted and what actually resulted.
So with the security pricing exception, for example, the AI will judge pass or fail on those exceptions. Anything that says it’s failed is passed onto a user to determine first, whether the machine was right, second, why it judged the way it did, and then feed the data into the machine to generate a better result the next time.
Only users who have a paid subscription or are part of a corporate subscription are able to print or copy content.
To access these options, along with all other subscription benefits, please contact info@waterstechnology.com or view our subscription options here: http://subscriptions.waterstechnology.com/subscribe
You are currently unable to print this content. Please contact info@waterstechnology.com to find out more.
You are currently unable to copy this content. Please contact info@waterstechnology.com to find out more.
Copyright Infopro Digital Limited. All rights reserved.
As outlined in our terms and conditions, https://www.infopro-digital.com/terms-and-conditions/subscriptions/ (point 2.4), printing is limited to a single copy.
If you would like to purchase additional rights please email info@waterstechnology.com
Copyright Infopro Digital Limited. All rights reserved.
You may share this content using our article tools. As outlined in our terms and conditions, https://www.infopro-digital.com/terms-and-conditions/subscriptions/ (clause 2.4), an Authorised User may only make one copy of the materials for their own personal use. You must also comply with the restrictions in clause 2.5.
If you would like to purchase additional rights please email info@waterstechnology.com
More on Emerging Technologies
This Week: Startup Skyfire launches payment network for AI agents; State Street; SteelEye and more
A summary of the latest financial technology news.
Waters Wavelength Podcast: Standard Chartered’s Brian O’Neill
Brian O’Neill from Standard Chartered joins the podcast to discuss cloud strategy, costs, and resiliency.
SS&C builds data mesh to unite acquired platforms
The vendor is using GenAI and APIs as part of the ongoing project.
Chevron’s absence leaves questions for elusive AI regulation in US
The US Supreme Court’s decision to overturn the Chevron deference presents unique considerations for potential AI rules.
Reading the bones: Citi, BNY, Morgan Stanley invest in AI, alt data, & private markets
Investment arms at large US banks are taken with emerging technologies such as generative AI, alternative and unstructured data, and private markets as they look to partner with, acquire, and invest in leading startups.
Startup helps buy-side firms retain ‘control’ over analytics
ExeQution Analytics provides a structured and flexible analytics framework based on the q programming language that can be integrated with kdb+ platforms.
The IMD Wrap: With Bloomberg’s headset app, you’ll never look at data the same way again
Max recently wrote about new developments being added to Bloomberg Pro for Vision. Today he gives a more personal perspective on the new technology.
LSEG unveils Workspace Teams, other products of Microsoft deal
The exchange revealed new developments in the ongoing Workspace/Teams collaboration as it works with Big Tech to improve trader workflows.