Data Issues Still Hamper AI—But AI Can Fix Them
Instead of waiting for data quality to be sufficient to power AI models, those at the cutting edge are building models to bridge the gaps in the data, and apply it to more sophisticated use cases.
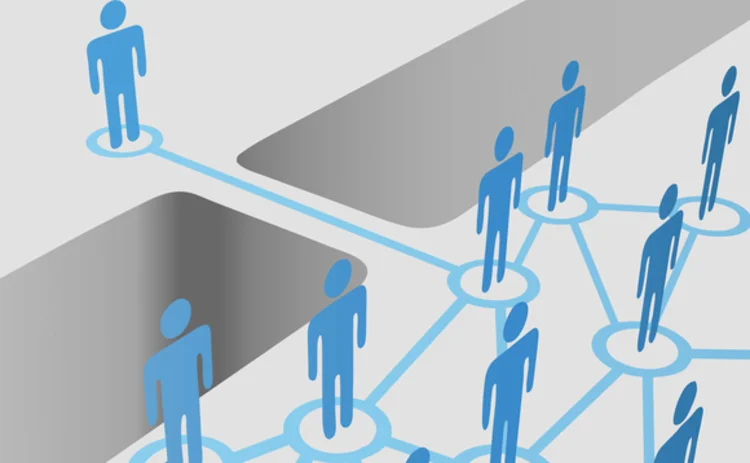
Dirty, incorrect, and incomplete data continues to pose barriers to adoption of artificial intelligence in key areas of financial firms’ workflows, such as development of trading algorithms. But there’s good news: the same AI techniques firms are using to create new trading models can also be used to fix the data issues that have traditionally hampered the effectiveness of data-hungry AI models for trading and analytics.
“All of these models are dependent on data, whether you’re using AI, machine learning, deep learning—all of these require a good set of data. Whether you’re writing in R, Python, or Matlab, the data is going to be an impediment to any kind of model,” said Laura Hamilton, MD and global head of treasury technology at Bank of America Merrill Lynch, speaking on a virtual panel at WatersTechnology’s Innovation Exchange event.
However, datasets that provide deep levels of historical data often have gaps or errors—a problem that has plagued these datasets, and any attempts to build models, from the start. These can be minor gaps in time-series data fields that can nevertheless disrupt a trading model or skew results, or can be major errors that seriously limit the amount of data that can be reliably used to test a model. Or a dataset that is relatively new may simply not have enough history to be effective for building or testing models.
“The truth is, the data is always outdated, inconsistent, contradictory, dirty, and it’ll never be better,” said Michael Natusch, global head of AI at Prudential. “But the reality is, humans are also making decisions based on that data. It’s 2020 now, and we have a whole bunch of tools that allow us to make sense of that data.”
For example, FactSet developed a model to detect errors and fill in missing values in its dataset of shipping information, which provides data on the supply of goods based on shipping containers arriving at their destination port. The data for each container comprises a code and a textual description of its contents. However, sometimes one of these identifiers would be missing or incorrect.
“For example, a container may have the code for coffee beans, but its description says ‘beach chairs’. So now the question is, Which one is right, and if one is missing, what do you do?” said Ruggero Scorcioni, VP and principal machine learning engineer at FactSet. “Now we are providing better shipping information by fixing the errors and filling in the missing information. We do this for [the data we make available to] our clients, but you can also do this internally if you have a large enough dataset,” he added, referring to the ability to use AI to fix the datasets required to power different AI models.
Hamilton also noted that there is “a big push around data management” underway to promote tools and techniques that can combine and analyze different data types—even unstructured data.
“Data will always be incomplete. The question is, will it be good enough for a specific model?” Scorcioni said, adding that firms should apply the “good-enough” philosophy to how it measures success of the models for which it uses the data. Just being able to apply machine learning to a problem and hoping for the best is not a plan for success, he said, adding that firms should understand what is the minimum threshold at which they can achieve value, rather than expecting technology to deliver big wins from day one. “The biggest lesson is to try to have a clear idea of what success looks like. Having a model that has 100% success is unrealistic. Having 95% is really, really hard. Is 70% success or not?”
To set realistic levels for success and manage expectations from the business, it’s key to work with a business partner who understands the realistic use cases, is willing to push the boundaries of technology together, and understands that there will be a lot of trial and error during the early stages of any AI project, Hamilton said.
“There’s a lot of foundational work to get the data right, to go through that learning curve, but once you get above that learning curve, there are so many things that you can run with. Then they start coming out of the woodwork, because it’s ‘the art of the possible.’ And when you start to demonstrate something after you’ve come over that curve, it’s amazing how people start lining up at your door to ask if they can try different use cases. And because there is so much potential for this, you get a lot of that engagement, a lot of that interest,” she said.
Only users who have a paid subscription or are part of a corporate subscription are able to print or copy content.
To access these options, along with all other subscription benefits, please contact info@waterstechnology.com or view our subscription options here: http://subscriptions.waterstechnology.com/subscribe
You are currently unable to print this content. Please contact info@waterstechnology.com to find out more.
You are currently unable to copy this content. Please contact info@waterstechnology.com to find out more.
Copyright Infopro Digital Limited. All rights reserved.
As outlined in our terms and conditions, https://www.infopro-digital.com/terms-and-conditions/subscriptions/ (point 2.4), printing is limited to a single copy.
If you would like to purchase additional rights please email info@waterstechnology.com
Copyright Infopro Digital Limited. All rights reserved.
You may share this content using our article tools. As outlined in our terms and conditions, https://www.infopro-digital.com/terms-and-conditions/subscriptions/ (clause 2.4), an Authorised User may only make one copy of the materials for their own personal use. You must also comply with the restrictions in clause 2.5.
If you would like to purchase additional rights please email info@waterstechnology.com
More on Data Management
New working group to create open framework for managing rising market data costs
Substantive Research is putting together a working group of market data-consuming firms with the aim of crafting quantitative metrics for market data cost avoidance.
Off-channel messaging (and regulators) still a massive headache for banks
Waters Wrap: Anthony wonders why US regulators are waging a war using fines, while European regulators have chosen a less draconian path.
Back to basics: Data management woes continue for the buy side
Data management platform Fencore helps investment managers resolve symptoms of not having a central data layer.
‘Feature, not a bug’: Bloomberg makes the case for Figi
Bloomberg created the Figi identifier, but ceded all its rights to the Object Management Group 10 years ago. Here, Bloomberg’s Richard Robinson and Steve Meizanis write to dispel what they believe to be misconceptions about Figi and the FDTA.
SS&C builds data mesh to unite acquired platforms
The vendor is using GenAI and APIs as part of the ongoing project.
Aussie asset managers struggle to meet ‘bank-like’ collateral, margin obligations
New margin and collateral requirements imposed by UMR and its regulator, Apra, are forcing buy-side firms to find tools to help.
Where have all the exchange platform providers gone?
The IMD Wrap: Running an exchange is a profitable business. The margins on market data sales alone can be staggering. And since every exchange needs a reliable and efficient exchange technology stack, Max asks why more vendors aren’t diving into this space.
Reading the bones: Citi, BNY, Morgan Stanley invest in AI, alt data, & private markets
Investment arms at large US banks are taken with emerging technologies such as generative AI, alternative and unstructured data, and private markets as they look to partner with, acquire, and invest in leading startups.