Data Issues Still Seen as Higher Investment Priority than AI
Investment spend is largely seen to be going to data management programs before emerging tech initiatives.
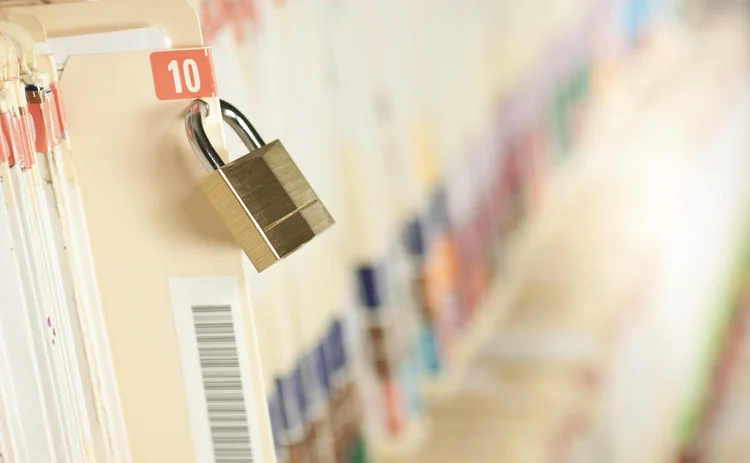
Despite widespread industry chatter around the perks and benefits of artificial intelligence (AI), banks are still largely concerned with more prosaic issues relating to data governance and management than they are emerging technologies.
The double whammy of data-intensive regulations going live in quick succession, specifically BCBS 239 and the General Data Protection Regulation (GDPR), in particular, has stretched the capabilities of bank technology and data departments.
In many instances, chief data officers (CDOs) say, this has been due to a lack of foresight in some areas of the industry around how to build effective platforms to tackle regulatory challenges associated with the two rules.
“Across the financial sector a lot of work has been done around the data infrastructure that supports the management of trading and product offerings, such as BCBS 239, and many banks started with a large legacy infrastructure that needed to be remediated for BCBS 239,” says Gary Goldberg, CDO at Mizuho International. “GDPR has required the same banks to implement similar controls and standards around personal data that BCBS 239 required for the financial data, but if an organization didn’t address BCBS 239 strategically and apply those same lessons to non-financial data, then it’s very possible that they had to start again for GDPR.”
This problem, Goldberg says, has provided a “salutary” lesson for banks in how to deal with complex data compliance projects, which can abruptly and quickly consume resources that might otherwise be deployed in more forward-looking initiatives, such as machine-learning projects and robotics research.
“[GDPR has kicked] some other projects we wanted to work on down the road a ways—that’s why it stings a bit to come to conferences like this and hear everyone talking about their latest machine-learning algo or AI integration, and I’m here like ‘yeah, I just spent a few million dollars on getting everyone to agree who’s in charge of data’. That’s me, by the way, so it doesn’t look like it’s going to get better any time soon.”
A Buy-Side Data Manager
A recent survey by data specialists Gresham Technologies, the Financial Information Management Association and WBR Insights also found that projects involving GDPR represented the highest level of complexity for financial institutions, which often jarred with ongoing projects—for instance, banks have struggled with the right to be forgotten and the feasibility of erasing personal information from distributed ledgers, which are designed to specifically prohibit such activity. As such, data projects still take the lead on investment dollars when compared to other technology projects.
“Without a solid base of data that can be trusted, the cost and risk barriers of innovating can be prohibitive,” says Bill Blythe, global business development director at Gresham.
Out of the 100 respondents surveyed, most said that they would still be pursuing emerging technology projects, with around 72 percent investing in artificial intelligence, and around 65 percent looking at initiatives related to distributed-ledger technology. However, the lion’s share of respondents—around 89 percent—said their highest investment would be in data-management programs.
“All of these underlying [disruptive] technologies help data management, and data management helps many of the other technologies,” said Chris Bannocks, CDO at ING, in the report. “Machine learning and AI require data in a certified state, unless you burden those technologies with data quality work.”
For digitalization, meanwhile, the growing focus is on managing the coexistence and overlap of data governance and data science considerations, according to Mizuho’s Goldberg.
“Data needs for data science can often differ to what is required for operational uses of data,” he says. “For example, data scientists need access to data across systems and business processes that might not otherwise be required for operational purposes. In order to deliver data science projects, it is important to understand the data, its source and ensuring it’s clean, and to this extent, data governance and data science are symbiotic partners. The better the data governance—and therefore the quality of the data—the better and more efficient the data science can be.”
This problem isn’t just confined to the sell side, either. Speaking on the sidelines of the SS&C Deliver conference in Las Vegas, one data management head at a prominent New York-based asset manager said that GDPR resembled a “waking nightmare” for them and their team.
“We spent months getting to grips with this foreign regulation, and the amount of work it’s consumed is simply incredible,” they said. “It’s even had to kick some other projects we wanted to work on down the road a ways—that’s why it stings a bit to come to conferences like this and hear everyone talking about their latest machine-learning algo or AI integration, and I’m here like ‘yeah, I just spent a few million dollars on getting everyone to agree who’s in charge of data’. That’s me, by the way, so it doesn’t look like it’s going to get better any time soon.”
Further reading
Only users who have a paid subscription or are part of a corporate subscription are able to print or copy content.
To access these options, along with all other subscription benefits, please contact info@waterstechnology.com or view our subscription options here: http://subscriptions.waterstechnology.com/subscribe
You are currently unable to print this content. Please contact info@waterstechnology.com to find out more.
You are currently unable to copy this content. Please contact info@waterstechnology.com to find out more.
Copyright Infopro Digital Limited. All rights reserved.
As outlined in our terms and conditions, https://www.infopro-digital.com/terms-and-conditions/subscriptions/ (point 2.4), printing is limited to a single copy.
If you would like to purchase additional rights please email info@waterstechnology.com
Copyright Infopro Digital Limited. All rights reserved.
You may share this content using our article tools. As outlined in our terms and conditions, https://www.infopro-digital.com/terms-and-conditions/subscriptions/ (clause 2.4), an Authorised User may only make one copy of the materials for their own personal use. You must also comply with the restrictions in clause 2.5.
If you would like to purchase additional rights please email info@waterstechnology.com
More on Emerging Technologies
This Week: Startup Skyfire launches payment network for AI agents; State Street; SteelEye and more
A summary of the latest financial technology news.
Waters Wavelength Podcast: Standard Chartered’s Brian O’Neill
Brian O’Neill from Standard Chartered joins the podcast to discuss cloud strategy, costs, and resiliency.
SS&C builds data mesh to unite acquired platforms
The vendor is using GenAI and APIs as part of the ongoing project.
Chevron’s absence leaves questions for elusive AI regulation in US
The US Supreme Court’s decision to overturn the Chevron deference presents unique considerations for potential AI rules.
Reading the bones: Citi, BNY, Morgan Stanley invest in AI, alt data, & private markets
Investment arms at large US banks are taken with emerging technologies such as generative AI, alternative and unstructured data, and private markets as they look to partner with, acquire, and invest in leading startups.
Startup helps buy-side firms retain ‘control’ over analytics
ExeQution Analytics provides a structured and flexible analytics framework based on the q programming language that can be integrated with kdb+ platforms.
The IMD Wrap: With Bloomberg’s headset app, you’ll never look at data the same way again
Max recently wrote about new developments being added to Bloomberg Pro for Vision. Today he gives a more personal perspective on the new technology.
LSEG unveils Workspace Teams, other products of Microsoft deal
The exchange revealed new developments in the ongoing Workspace/Teams collaboration as it works with Big Tech to improve trader workflows.