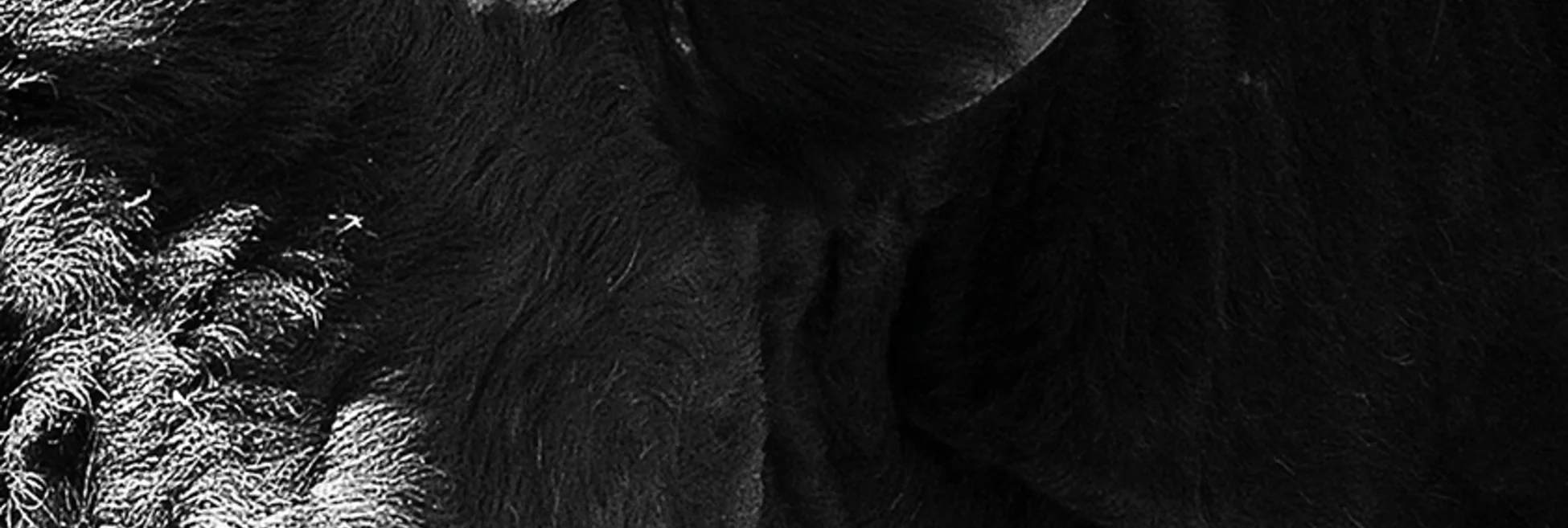
'Data Mining is Bullsh*t' — An Examination
With the growth of alternative data in the capital markets, firms are struggling to find value, and are disillusioned by the loss of time, human capital, and money. Goldman Sachs’ Matthew Rothman believes this has created a situation where vendors and buy-side firms are promising vast riches, but much of that talk, he says, is BS. As you might expect, not everyone agrees.
“Are you willing to take the risk of investing in bullshit? Or do you think that by not investing in what might be bullshit, you’re leaving alpha on the table, and you’re going to lose to the people who are more comfortable, potentially, investing in bullshit—or found something that’s just really cool—and [that] your minds are really shallow?”
This is the controversial question posed by Matthew Rothman, a managing director at Goldman Sachs, during his opening presentation—Data Mining: The (un)Original Sin—at the Quandl Data Conference on January 23 in New York.
Like alternative data, his talk even had its own backstory: It was originally titled “Data Mining: The Second Oldest Profession”—a coy reference to prostitution being the first. Unsurprisingly, the idea didn’t fly with the bank’s compliance department.
Next, he tried his luck at naming it “Data Mining By Dummies”—a play on the real book, “Data Mining For Dummies”—but compliance didn’t seem to like that one any better. In a third attempt, he vied for “The Astrology of Finance: Unlocking Patterns in Nonstationary Data with Machine Learning,” before realizing that was the actual title of an upcoming talk someone was planning to give during an IBM plenary session.
“And then I was going to name it ‘On Hogwash’—there’s another word [for that]. We’ll just say it once: bullshit—‘In Empirical Analysis and Finance.’ … That really is the true topic, the true title of this talk,” Rothman said.
When he first started out in the industry as a research associate at Goldman Sachs Asset Management in 2001, the worst thing you could call someone was a “data miner,” Rothman said. Now, firms have carved out specific roles for the practice, such as the hedge funds that now hire “data hunters,” who are tasked with finding new and unique datasets—a byproduct of the alternative data boom, which has created a world where anyone can access practically any kind of data they want, Rothman said. If you don’t already have the data you want, he added, it’s only because you haven’t looked hard enough.
But while having all the data in the world at your fingertips is an enticing thought, it’s not necessarily useful—or even a good thing.
Investors, asset managers, and hedge funds have flocked to alt data—a trend driven by FOMO, otherwise known as the fear of missing out, Rothman said—in pursuit of increasingly elusive alpha. But in their quests, many realize there is no “there” there.
Regardless, the toil continues. Outlandish datasets are a burgeoning area of research for financial markets participants. For example, face width-to-height ratios can indicate a portfolio manger’s tendencies toward unnecessary risk-taking, according to this study by Yan Lu of the University of Central Florida, and Melvyn Teo of Singapore Management University, which has been downloaded more than 3,800 times. Rothman attributes this, along with the shift in the dirty-word status of “data miner,” to firms’ newfound willingness to accept type-one errors, or errors that generate positive results despite being clearly false.
“I don’t really think that we have a problem in finance of people producing things that they know are just wrong and putting them in their models,” Rothman said. “I think there’s a problem of people not knowing anything about data or science—and so they produce bullshit.”
It’s not just: ‘I’m going to make a big stew, and take every piece of crap that I can find in the kitchen cupboard, throw it in there and hope it tastes good at the end.’ It won’t taste good because you’re putting licorice in with fucking kidney beans.
Alexander Fleiss, Rebellion Research
To illustrate his point, Rothman offered up a half-dozen datasets, including one involving penis size correlated to a country’s GDP growth over the long-term, which is a real dataset, but here are three austere examples, with two that are real, and one that is fake: First, in 2003, Cesare Robotti, professor of finance at Warwick Business School, and Boston College’s Anna Krivelyova published a paper that found that unusually high geomagnetic activity is associated with negative stock index returns—5.5% lower—even after controlling for other calendar effects across international markets (real).
Second, a 2009 study led by Cambridge neuroscientist John Coates found that the traders at a major high-frequency trading firm in London whose index fingers were relatively shorter than the ring finger earned 5.4 times the profit than their inverse colleagues, controlling for age and experience (real).
Finally, a 2017 working paper by MIT concluded that nations in which country singer Shania Twain had at least two local Billboard-charting songs from 1995 to 2002 saw GDP growth rates 2.6 times higher on average over those years, compared to countries where she did not top the music charts (fake).
“The only one that actually has some real economic story is actual bullshit,” Rothman said. “So on that, I conclude—be diligent, be skeptical, and be wary of bullshit.”
Data Stew
The problem isn’t necessarily a question of selecting the best alt data vendor or analytics platform; rather, the heart of the issue is not having the faintest idea what you’re looking for or what you want to prove, says Alexander Fleiss, co-founder and CEO of quant hedge fund Rebellion Research. Fleiss, also an adjunct professor teaching artificial intelligence and machine learning at Cornell Financial Engineering Manhattan, “100%” agrees with the view that finance as a whole is undereducated in data and science. As a result, many so-called data-mining exercises prove to be futile efforts.
All too often, he says, he comes across students and engineers—“brilliant kids”—who don’t have clear objectives for their data and their algos, and because of that, their projects fail.
“They throw in every piece of junk they can think of, and in the end, they get a piece of junk,” Fleiss tells WatersTechnology. “It’s not just: ‘I’m going to make a big stew, and take every piece of crap that I can find in the kitchen cupboard, throw it in there and hope it tastes good at the end.’ It won’t taste good because you’re putting licorice in with fucking kidney beans.”
That said, alpha has to be found somehow. And sometimes, traders and portfolio managers do put some faith in riskier, unconventional datasets. To illustrate the point, he gives the example of a portfolio manager who’s stuck a lot of their position on arts-and-crafts company Michaels. A data vendor comes along and offers real-time data on the business’ returns. In looking at that data, the portfolio manager decides those returns are highly correlated with XYZ and takes a position based on that information.
“Is that outlandish? Kind of. Will that guy survive? Maybe not. Will he pay a lot for it? Probably. Would he have 40 years ago? Maybe not—but now it’s harder to find alpha,” Fleiss says.
A Rebuttal
Suvrat Bansal, chief data officer and head of innovation at UBS Asset Management, who has a long history working on big-data endeavors at buy-side firms, rejects the theory that FOMO runs amok on the buy side, along with the view that finance has a data science knowledge problem. Rather, he believes the issue is that firms are still learning how to incorporate these new sources of information—particularly smaller firms and newcomers, which have long journeys ahead to unlock full value. But when managed properly, these datasets do hold a lot of promise.
The first thing the science and data field teaches the industry, he says, is that correlations are not causations. If they were the same, everyone could start making money on correlations. Despite that, markets are ripe for disruption, resulting in not only information overload, but requiring new means of interpreting that information.
I want to go after the truth; I want to go after fully understanding what’s moving this company or this sector of the market. And wherever I do not fully trust official sources, I want to really try and leverage alternative data and data science techniques to extract an independent view.
Suvrat Bansal, UBS Asset Management
“At the end of the day, long-term returns speak to the value. The dynamics in the market right now are changing,” says Bansal. “Based on traditional value drivers, Walmart should have never been displaced by companies like Amazon. When you look at the macroeconomic drivers and the changing digital space, I think you have to say, ‘Look, while I don’t know exactly which factors are transpiring for me to understand what is the future of a high-value company, which has reigned in the market for 20 years, I have to first start with being open [to new data].’ That doesn’t mean you draw correlations and start shorting or longing Walmart.”
For asset managers with a long-term focus, the strongest use-case for alt data is as another form of research, which can add context to—and validate—broader fundamentals, Bansal says. One area of focus for him is climate awareness and sustainability. In a recent discussion with an academic about companies’ physical and transition risks—i.e., the change in assets’ value in a low-carbon economy scenario—the pair concluded that every company is exposed when it comes to weather and climate, making mining for that data crucial.
“If you do not understand that data, which has nothing to do with your fundamental data, how would we ever understand that?” says Bansal, referring to the exposure of each company to physical and transitional risk, which he believes is so crucial to understand that it basically makes the subset of alt data, fundamental.
Asked whether he thinks data mining is bullshit, he responds that it’s “kind of catchy to say these things,” but “it goes to the very discipline of what finance does as a field.”
Though he doesn’t see FOMO prevailing within the buy side, Bansal says alt data has allowed traders and investors to trust previously unreliable data. For example, in the energy market, in which certain countries are suspected to be fudging reports on how much oil they’re transporting or how full their containers are, data sources such as satellite imagery and shipment weights have alleviated some of those concerns.
“I want to go after the truth; I want to go after fully understanding what’s moving this company or this sector of the market,” Bansal says. “And wherever I do not fully trust official sources, I want to really try to leverage alternative data and data science techniques to extract an independent view.”
(Story continues after the box)
Make of It What You Will
WatersTechnology asked Tradeweb, which operates trading platforms in fixed income, money markets, and ETFs, and trades more than $800 billion per day, to look at clearly spurious data points that could correlate with yields, and—hypothetically, as Tradeweb is not in the business of offering investment advice—how those findings could be used.
Tradeweb’s head of research, Jonathan Rick, looked at average daily Treasury yields alongside reports from the US dairy industry. In summary, as Treasury yields have fallen, so has the consumption of full-fat ice cream (Chart 1, bottom of the page), while low-fat ice cream has seen rising demand (Chart 2); Americans are eating more butter (Chart 3); and Treasury yields seem to move in lockstep with availability of sherbet (Chart 4).
“Obviously, we hazard against drawing conclusions about Treasury yields from dairy data,” Rick says. “If you were, though, then other things to consider would be crop and dairy production reports, or issues with supply lines. If you’re really keen, you could look at satellite imaging and analyze supply-chain blockchains to determine which products might be produced. There are also qualitative signals to consider—like whether everyone you know has gone keto, or whether The New York Times’ recipe website has pivoted toward dairy alternatives.”
There probably won’t ever be a consensus on whether unearthing the most unique and outlandish of data is useful for finance or not—but from a theoretical perspective, the correlations are limitless.
Finance and Science: Strange Bedfellows
Most people who have taken a statistics class know that correlation and causation are not the same thing. But humans are wired to seek out patterns and dissect them. That same instinct is the one that compels people to see images of Jesus in potato chips—or ill-fated gorillas in Cheetos, in the case of one individual, who paid $99,900 for a single Cheeto because it resembled the now-infamous Harambe. And it can come into play amid the limitless influx of new datasets.
If you disagree with Rothman and Fleiss—on whether finance’s problem is a data and science knowledge gap—and also disagree with Bansal—that the majority of asset managers aren’t succumbing to “bullshit” data—then you might agree with Jay Finkelman, professor and chair of industrial-organizational business psychology at the Chicago School of Professional Psychology, who says the worlds of finance and science are diametrically opposed.
“[In science], if there’s more than a 5% chance that something may not be true, you don’t publish it because that could be embarrassing. In finance, you may have to shift those around because fame goes to those who call things correctly, and people tend to forget about the missteps,” Finkelman says. “They’re in a fast-moving market where you have to stay ahead. And the risk of letting something hot escape you is probably perceived as a greater risk than the risk of making a mistake.”
Because there’s so much variety and volume, you can pretty much have anything you want to have confirmed, confirmed. So therein lies the danger: A, do you know what you’re doing? And B, are you being honest with yourself? I think, sometimes, you’re so hoping your hypothesis to be right, you believe it, so you see it.
Mike Chen, PanAgora Asset Management
Of course, no one smiles on those who lose millions of dollars of other people’s money, and the reputational damage that comes with making mistakes is huge. Still, those blunders don’t typically disqualify market participants from from playing the game. Finkelman points to another example: History remembers who called the last downturn, especially if no one was listening, but it will forget all the wrong calls in between.
Anyone looking at information or data sources, in general, is unconsciously using principles of cognitive consonance and cognitive dissonance, Finkelman says, meaning they’re more receptive to things that are consistent with their own worldview and more hostile toward what isn’t. It’s a defense mechanism that protects oneself from information overload. In a sea of data, it’s easy for traders to select information that proves their hypothesis—full-fat ice cream consumption correlates to Treasury yields, but that can fail to take in a range of other, vital information that could potentially reject that theory, or better explain why the two track together.
It’s also why, in searching for a signal, a more intuitive idea doesn’t always work either, noted Goldman’s Rothman, who declined to expand on his talk. There’s an idea that if you obtained the history of Equal Employment Opportunity Commission (EEOC) complaints by organization—which are available from vendors or via a Freedom of Information Act request—the signal would be that companies with more complaints filed against them would have more hostile work environments, and therefore, are low-quality companies that will get negative publicity.
“Makes perfect sense on the face of it,” Rothman said during his talk. “But there’s a bit of a problem here. You have a big [censoring of data] problem, and you have a huge bias into what’s actually going into that dataset. Think about it: The complaints that have a high likelihood of having the most damaging and egregious information … as we all know, never see the light of day—they all get settled with NDAs. … So what you end up [with] is that companies that should have the lowest promise—the lowest score on your measure—aren’t anywhere in there and might actually appear good.”
A skilled data skeptic would see additional problems with that dataset. Mike Chen, director of portfolio management at PanAgora Asset Management, says there are even more issues to parse with that example—the need to correct for size, industry, and the nature of different job roles, are among just some of them. However, he adds, not accounting for those types of things is a common mistake.
“Because there’s so much variety and volume, you can pretty much have anything you want to have confirmed, confirmed. So therein lies the danger: A, do you know what you’re doing? And B, are you being honest with yourself? I think, sometimes, you’re so hoping your hypothesis to be right, you believe it, so you see it,” he says.
Though yes, portfolio managers, data scientists, and analysts do have to follow their intuition right up until they find out whether they’re right or wrong, they should be able to validate (or invalidate) that intuition along the way through a concept called ancillary testing—a traditionally medical term reserved to describe a wide range of diagnostic tests. If fewer complaints truly led to better workplaces, then one should also see, for example, higher average ratings on employment feedback websites, employees taking fewer sick days, and higher profit margins from high productivity.
And it can’t be overstated that different factors can make for odd bed fellows—and that’s the draw—but that doesn’t mean, “Buy, buy, buy!” There’s a famous correlation that says the amount of butter churn in Bangladesh is 99.9% correlated with the S&P 500—it’s an investment correlation that has been largely debunked. The fact is that data in capable hands is power, Chen says. If you can construct the right hypothesis and run the right statistical tests, you’re better off—but that’s a big “if.”
‘Really, Absolutely No Idea’
Others take the view that it doesn’t actually matter whether the data is good or bad, useful or not, because it’s always going to be subjective. The key to evaluating all of it in order to decide which of those categories data falls into for yourself is having the ability to onboard and process it at scale—a lofty task that data management and engineering firm Crux Informatics is looking to tackle. The startup counts Goldman Sachs, Citi, and Two Sigma as investors.
Philip Brittan, Crux’s CEO, says firms he talks to—primarily hedge funds—tell him that only about 10% of datasets they look at actually have some kind of value, leaving money, time, and effort wasted most of the time. (It’s worth noting that PanAgora’s Chen found that figure even a bit high, estimating that between 5% and 10% of alt datasets he comes across are useful.) So even though a firm incorporates a scientific process, two people can look at the same dataset and derive completely different levels of value. On top of that, in the hedge fund world, which promises high rewards for high risks, the pressure to succeed is heaviest.
“People don’t want to overlook anything. But they are coming to the realization that useful data are really needles in haystacks, and you go through a lot of hay before you find a needle,” Brittan says.
And to find a needle, two questions must be answered: Does it have predictive power and add alpha? And is it novel compared to other data a trader might have access to?
While the questions seem black and white, the answers rarely are—that’s why some alt data vendors, like geospatial data provider Thasos Group, are shrinking, consolidating, or going out of business, Brittan says. The market can’t hold too many entities of a uncertain value.
Still, according to statistics provided by alternativedata.org, the alt data market is expected to be worth $350 million in 2020, and 78% of firms use or expect to use alt data (up from 52% in 2016). Bullshit or not, there are plenty of buyers in this market.
There’s a metaphor that says data is the new oil, and you need to mine that data to find the valuable oil. But maybe data-as-manure is actually more apt: When combined effectively with scientific methods, it can be used to improve crop production and soil quality; it can be used as an energy source and even as a material to help build structures. Not all shit is bad … the key lies in knowing your shit and how to use it.
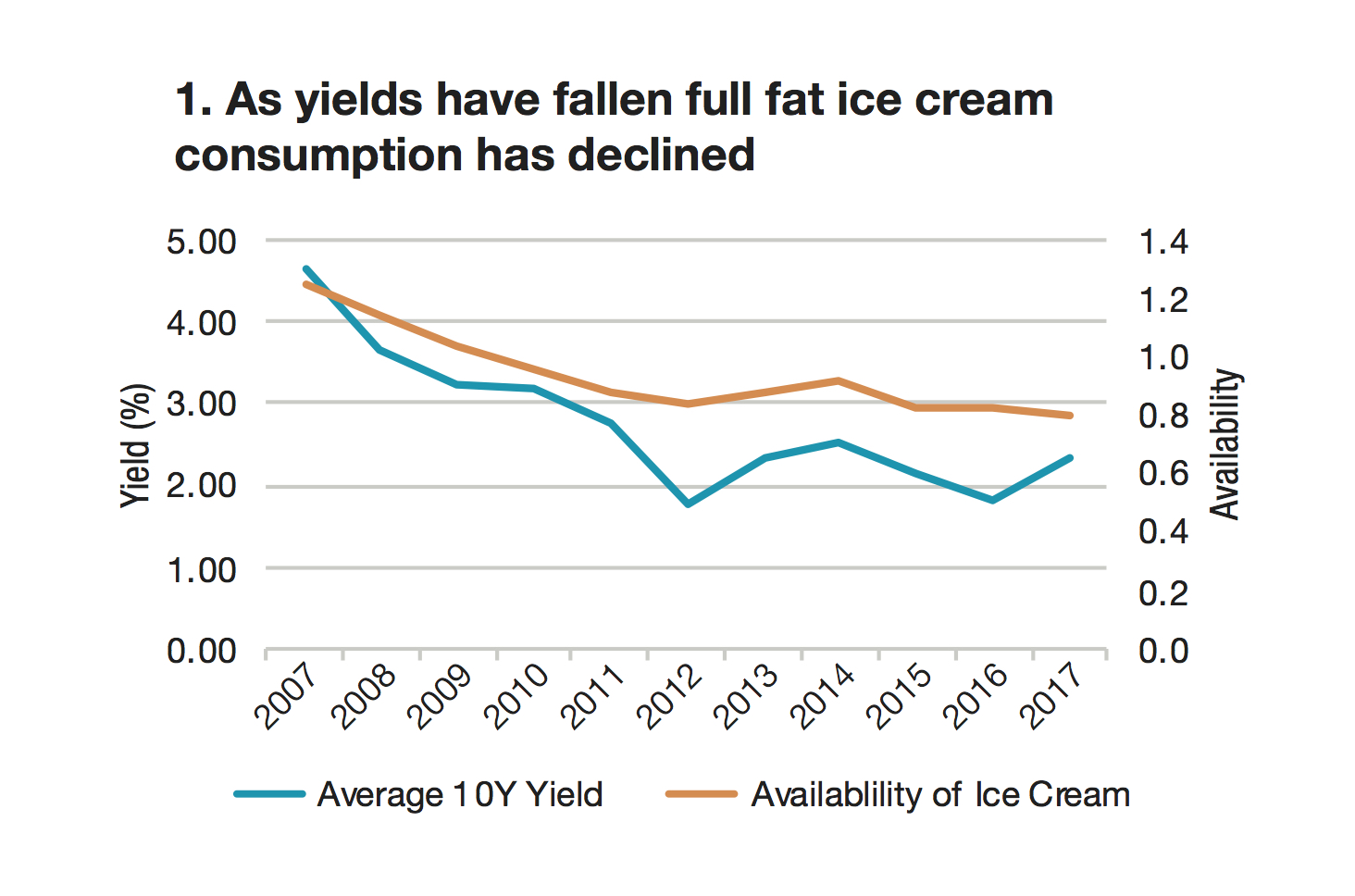
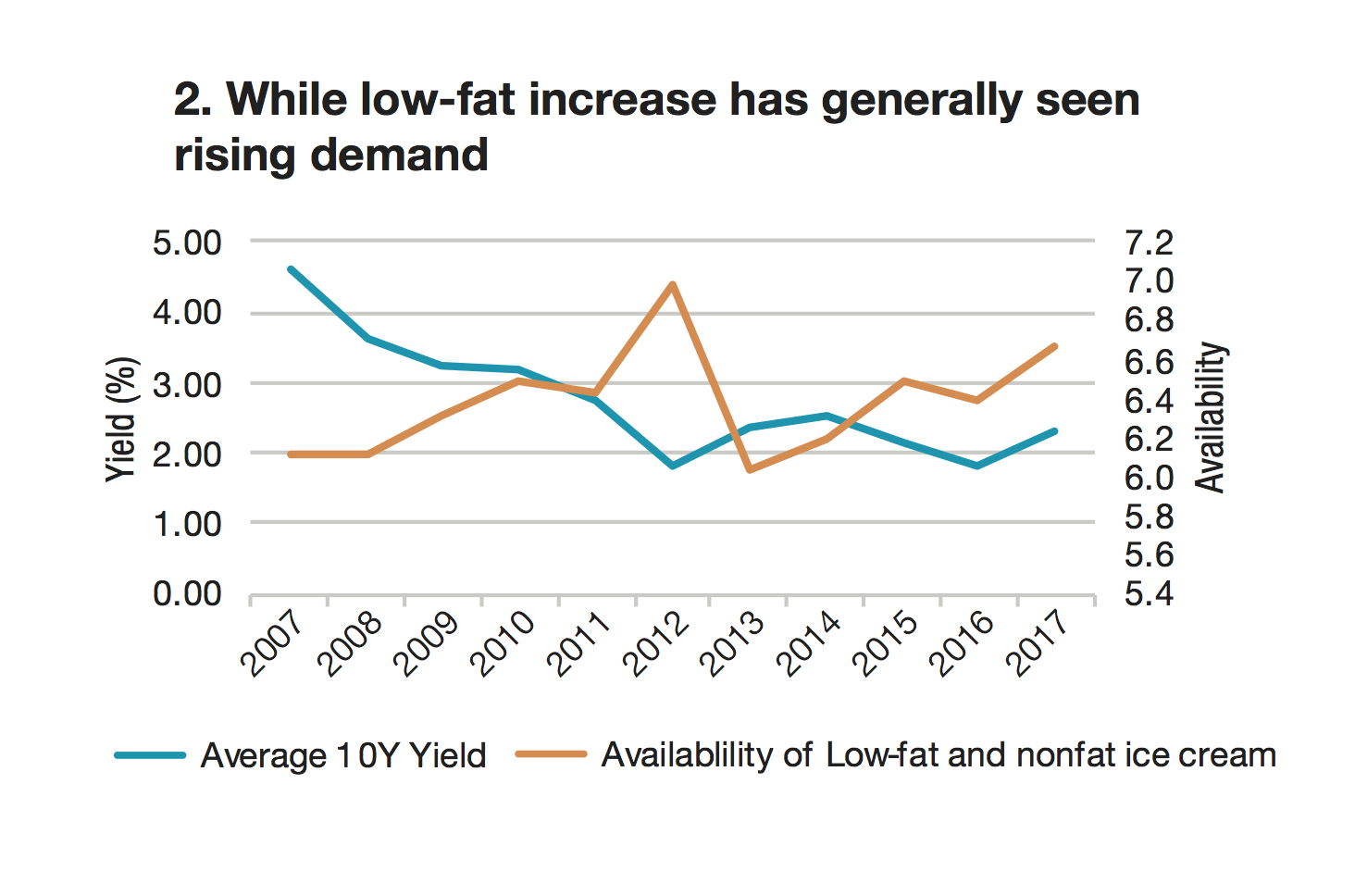
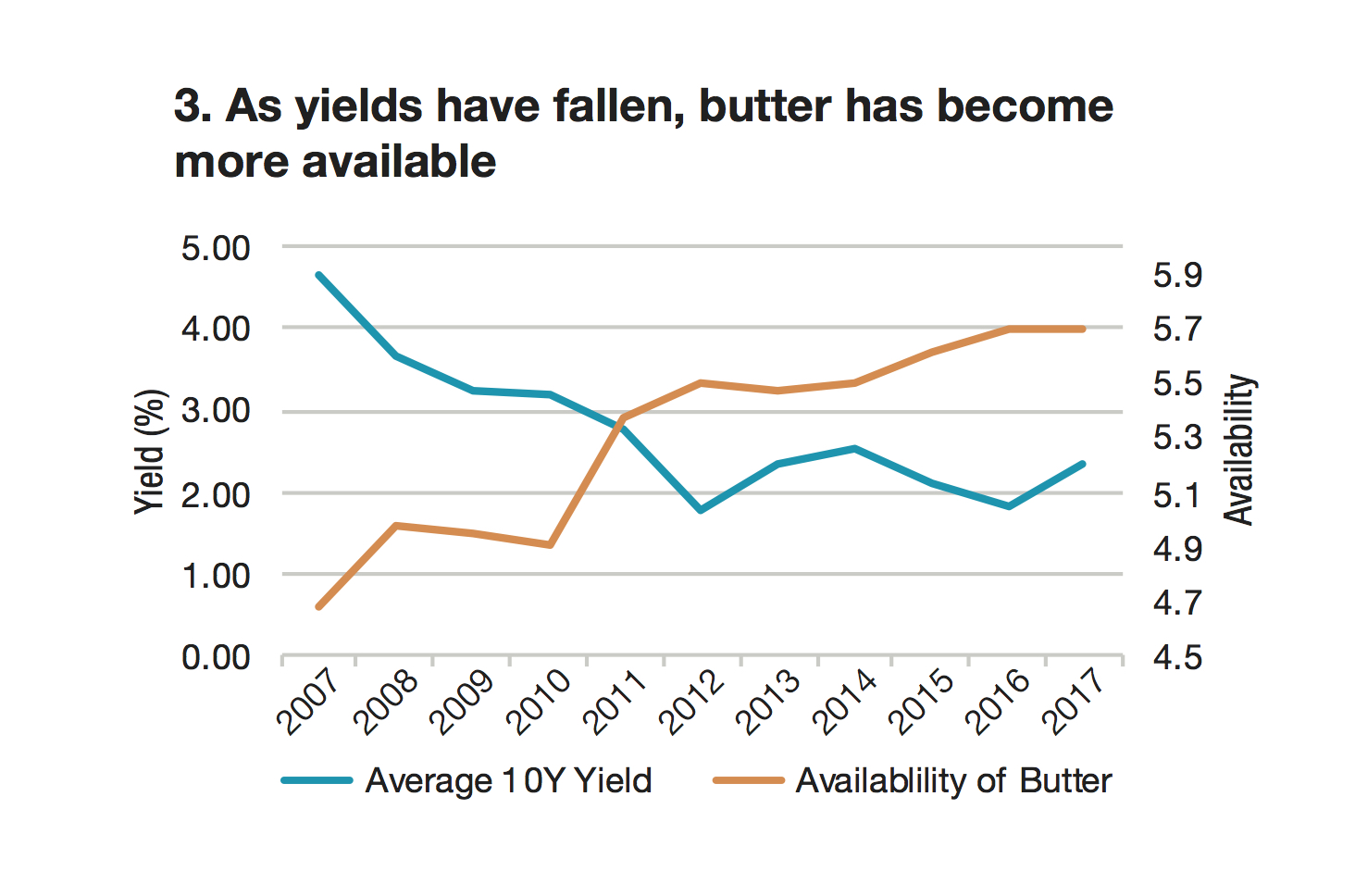
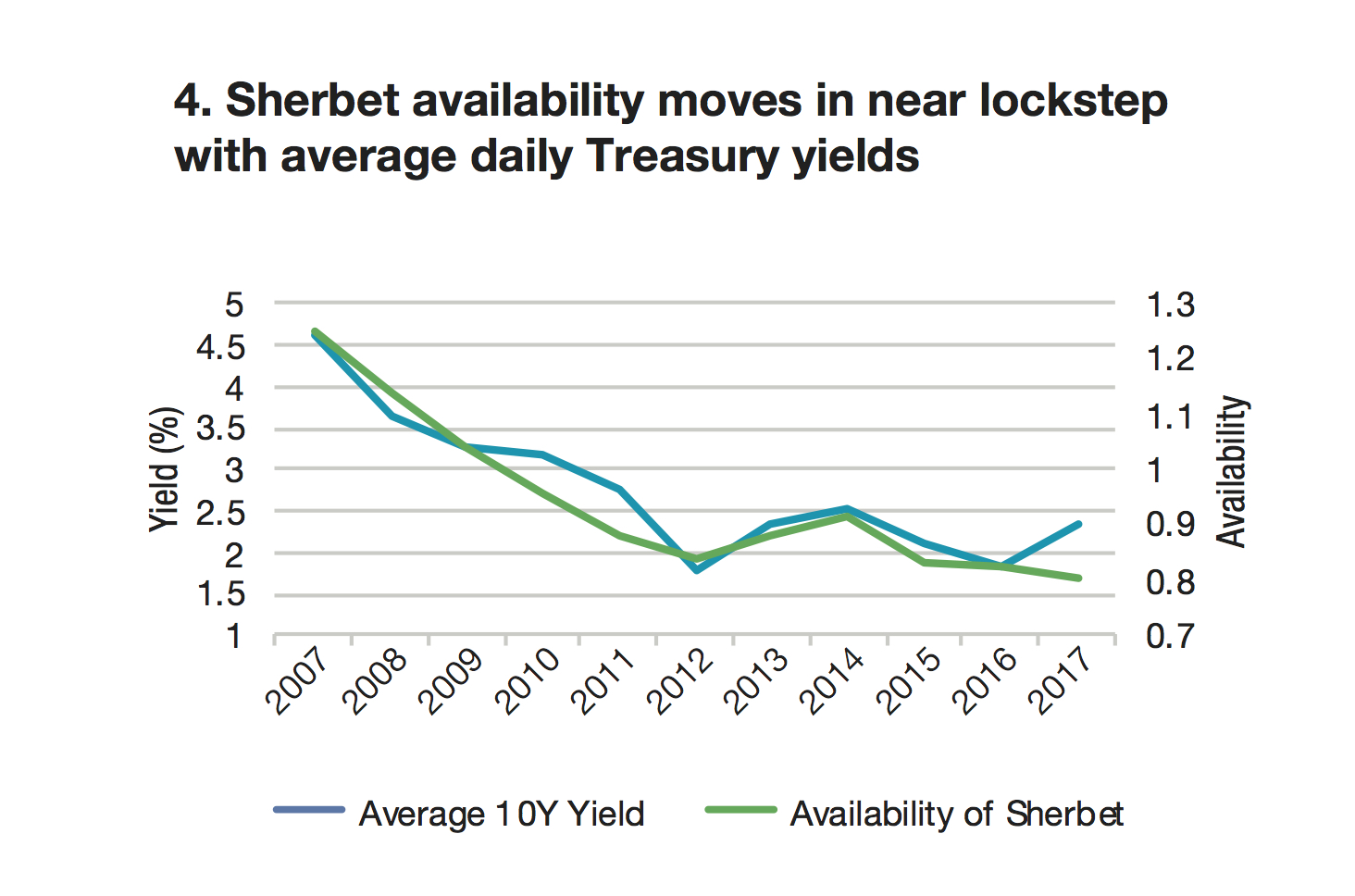
Further reading
Only users who have a paid subscription or are part of a corporate subscription are able to print or copy content.
To access these options, along with all other subscription benefits, please contact info@waterstechnology.com or view our subscription options here: http://subscriptions.waterstechnology.com/subscribe
You are currently unable to print this content. Please contact info@waterstechnology.com to find out more.
You are currently unable to copy this content. Please contact info@waterstechnology.com to find out more.
Copyright Infopro Digital Limited. All rights reserved.
As outlined in our terms and conditions, https://www.infopro-digital.com/terms-and-conditions/subscriptions/ (point 2.4), printing is limited to a single copy.
If you would like to purchase additional rights please email info@waterstechnology.com
Copyright Infopro Digital Limited. All rights reserved.
You may share this content using our article tools. As outlined in our terms and conditions, https://www.infopro-digital.com/terms-and-conditions/subscriptions/ (clause 2.4), an Authorised User may only make one copy of the materials for their own personal use. You must also comply with the restrictions in clause 2.5.
If you would like to purchase additional rights please email info@waterstechnology.com
More on Data Management
New working group to create open framework for managing rising market data costs
Substantive Research is putting together a working group of market data-consuming firms with the aim of crafting quantitative metrics for market data cost avoidance.
Off-channel messaging (and regulators) still a massive headache for banks
Waters Wrap: Anthony wonders why US regulators are waging a war using fines, while European regulators have chosen a less draconian path.
Back to basics: Data management woes continue for the buy side
Data management platform Fencore helps investment managers resolve symptoms of not having a central data layer.
‘Feature, not a bug’: Bloomberg makes the case for Figi
Bloomberg created the Figi identifier, but ceded all its rights to the Object Management Group 10 years ago. Here, Bloomberg’s Richard Robinson and Steve Meizanis write to dispel what they believe to be misconceptions about Figi and the FDTA.
SS&C builds data mesh to unite acquired platforms
The vendor is using GenAI and APIs as part of the ongoing project.
Aussie asset managers struggle to meet ‘bank-like’ collateral, margin obligations
New margin and collateral requirements imposed by UMR and its regulator, Apra, are forcing buy-side firms to find tools to help.
Where have all the exchange platform providers gone?
The IMD Wrap: Running an exchange is a profitable business. The margins on market data sales alone can be staggering. And since every exchange needs a reliable and efficient exchange technology stack, Max asks why more vendors aren’t diving into this space.
Reading the bones: Citi, BNY, Morgan Stanley invest in AI, alt data, & private markets
Investment arms at large US banks are taken with emerging technologies such as generative AI, alternative and unstructured data, and private markets as they look to partner with, acquire, and invest in leading startups.