Data Poisoning: An Emerging Threat for Machine Learning Adoption
Experts from IBM and Bank of China say they're on the lookout for this emerging threat, as machine learning gains in popularity.
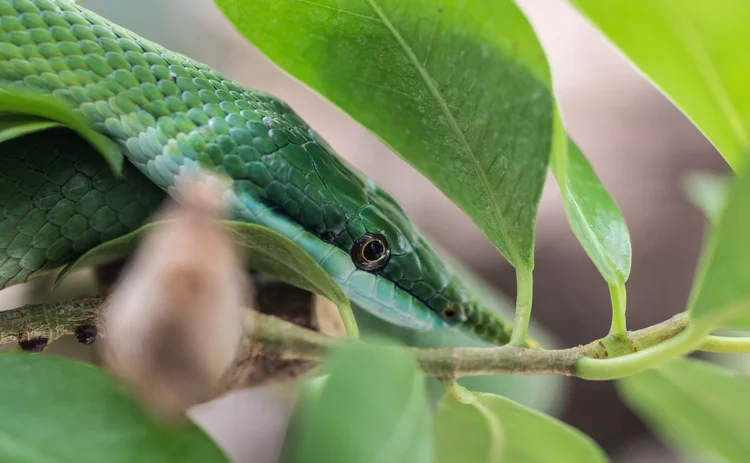
Slowly, banks are looking to incorporate machine learning into their front-end operations. As machine learning models become more prevalent in finance, experts warn that banks need to be on the lookout for a lurking threat: data poisoning.
Machine learning models are made by humans, and it’s those humans that bank executives need to be monitoring, says Gary Yiu, head of IT audit at Bank of China (Hong Kong), as “malicious users can inject false training data with the aim of corrupting the learning model.”
Yiu tells WatersTechnology that he expects the retail side of banks to be the most susceptible to these types of attacks, as that’s where a lot of the front-end ML development is occurring today.
“For investment banks, it may take a longer time when more artificial intelligence or machine learning applications comes to the operation,” he says. “There are many models established for retail, corporate, and investment banks, and more and more AI/ML applications are used for retail banking due to availability of massive data. As such, I would say the data poisoning or other data-based attacks would become more impactful in the future for retail banking.”
Although Yiu contends that data poisoning is more likely to be targeted at the retail side of the organization, machine learning is increasingly being used for portfolio building and forecasting, and quants are leaning on these algos to group assets more effectively.
The performance of machine learning very much hinges on the quality and accuracy of the data fed into the models. If someone were to tamper with the data, it could jeopardize the performance of the model.
David Cox, IBM director of the MIT-IBM Watson AI Lab, tells WatersTechnology that data poisoning is an “emerging threat frontier” that IBM is exploring. The answer might just be using AI to monitor other AI models. As an example, one algo could monitor for suspicious activities undertaken by a machine learning model where poisoned data was incorporated in order to help in a money-laundering scheme.
“How could you make other transactions that would obscure the fact that you are money laundering? That could either be done through [data] poisoning—you make transactions that you think will be in the dataset—or it could be [done] through what is called an adversarial attack,” he says. “The way that it works is you analyze the algorithm that is being used to detect the fraud, and then you carefully craft what you do to create data that evades that detector.”
He gives the example of a training model used for a self-driving car. If someone—say a hacker or compromised employee—were to feed an autonomous car training dataset with adverse examples, the system could be inadvertently taught to fail, thus endangering lives.
“It is more complicated to think about how that would work in the financial markets, but it is absolutely a threat model [that] we all need to be looking at and be on top of,” he says. “We actually have a fair amount of work going on in the lab where we are inventing these attacks—not because IBM wants to attack you; we absolutely do not want to attack anyone—the reason we are doing it is like the white hat hacking: we want to figure out the attacks, because if we do not figure them out first, a bad actor could.”
Editor’s note: The first quote provided by Yiu was given during a panel discussion at the inaugural WatersTechnology Innovation Exchange, the second quote was given in a separate interview after the event.
Further reading
Only users who have a paid subscription or are part of a corporate subscription are able to print or copy content.
To access these options, along with all other subscription benefits, please contact info@waterstechnology.com or view our subscription options here: http://subscriptions.waterstechnology.com/subscribe
You are currently unable to print this content. Please contact info@waterstechnology.com to find out more.
You are currently unable to copy this content. Please contact info@waterstechnology.com to find out more.
Copyright Infopro Digital Limited. All rights reserved.
As outlined in our terms and conditions, https://www.infopro-digital.com/terms-and-conditions/subscriptions/ (point 2.4), printing is limited to a single copy.
If you would like to purchase additional rights please email info@waterstechnology.com
Copyright Infopro Digital Limited. All rights reserved.
You may share this content using our article tools. As outlined in our terms and conditions, https://www.infopro-digital.com/terms-and-conditions/subscriptions/ (clause 2.4), an Authorised User may only make one copy of the materials for their own personal use. You must also comply with the restrictions in clause 2.5.
If you would like to purchase additional rights please email info@waterstechnology.com
More on Data Management
New working group to create open framework for managing rising market data costs
Substantive Research is putting together a working group of market data-consuming firms with the aim of crafting quantitative metrics for market data cost avoidance.
Off-channel messaging (and regulators) still a massive headache for banks
Waters Wrap: Anthony wonders why US regulators are waging a war using fines, while European regulators have chosen a less draconian path.
Back to basics: Data management woes continue for the buy side
Data management platform Fencore helps investment managers resolve symptoms of not having a central data layer.
‘Feature, not a bug’: Bloomberg makes the case for Figi
Bloomberg created the Figi identifier, but ceded all its rights to the Object Management Group 10 years ago. Here, Bloomberg’s Richard Robinson and Steve Meizanis write to dispel what they believe to be misconceptions about Figi and the FDTA.
SS&C builds data mesh to unite acquired platforms
The vendor is using GenAI and APIs as part of the ongoing project.
Aussie asset managers struggle to meet ‘bank-like’ collateral, margin obligations
New margin and collateral requirements imposed by UMR and its regulator, Apra, are forcing buy-side firms to find tools to help.
Where have all the exchange platform providers gone?
The IMD Wrap: Running an exchange is a profitable business. The margins on market data sales alone can be staggering. And since every exchange needs a reliable and efficient exchange technology stack, Max asks why more vendors aren’t diving into this space.
Reading the bones: Citi, BNY, Morgan Stanley invest in AI, alt data, & private markets
Investment arms at large US banks are taken with emerging technologies such as generative AI, alternative and unstructured data, and private markets as they look to partner with, acquire, and invest in leading startups.