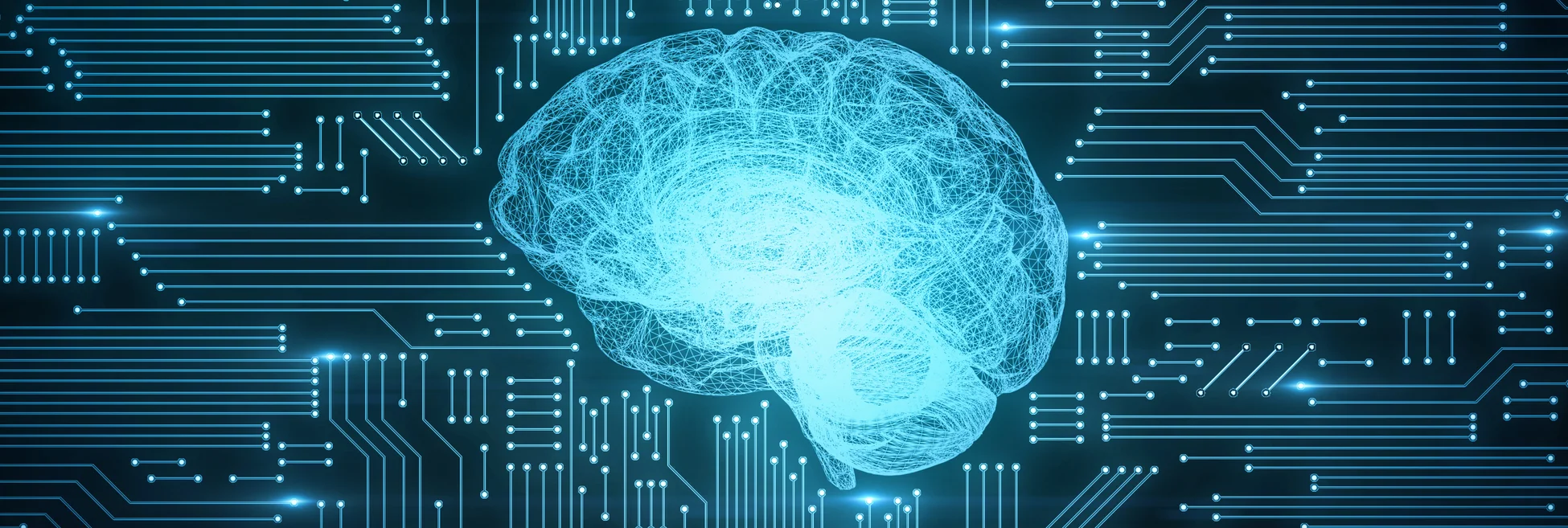
Deep Learning: The Evolution is Here
Advancements in AI have led to new ways for firms to generate alpha and better serve clients. The next great evolution in the space could come in the form of deep learning. WatersTechnology speaks with data scientists at banks, asset managers and vendors to see how firms are experimenting with this form of machine learning, and where challenges still exist.
While not quite ubiquitous, examples of deep learning’s evolution exist in parts of technologies we now use every day. From Facebook’s facial recognition software, to Tesla cars that assist with parallel parking, to Google Translate making Mandarin easy to understand for the unacquainted, to Amazon’s Alexa giving advice on the best way to fry a turkey, deep learning—in combination with other forms of artificial intelligence (AI) and mathematics—is seeping into everyday life.
Like a matryoshka doll, deep learning is a subset of machine learning, which itself is a subset of AI, although the terminology for the discipline has been bastardized in recent years. Much like how the word “blockchain” gets used to describe all forms of distributed-ledger technologies even though it is a specific kind of distributed ledger, deep learning and machine learning are increasingly being used interchangeably. Despite this, an important distinction exists—although all forms of deep learning are essentially machine learning, not all machine learning techniques can be classified as deep learning.
Deep learning—which, at its core, is a form of math—uses a computer system that mimics the workings of the human brain, called a neural network. Deep neural networks are opaque, but they can process massive amounts of data and can essentially “learn” on their own. Each layer of nodes in a neural network builds on the previous layer—the more layers, the deeper it is. They require massive volumes of data and when at their best, they can find non-linear correlations and can produce outputs that would take a team of humans months, weeks, or even years to figure out. At their worst, they’re subject to bias—even outward, unintentional racism—and are often not worth the trouble and expense when compared with using other forms of machine learning, such as decision trees, Bayesian networks or support vector machines. There’s a lot of experimentation and failure involved in using this still-growing technique.
But improvements are being made, and past what was once purely theoretical in the capital markets, deep learning is starting to find a home in finance—though it’s still very early days. Examples exist, though: deep neural networks are being experimented with in areas including risk modeling, market forecasting, customer relationship management, stress testing, surveillance and to tame the wild forest that is the alternative data space.
“In some ways, it’s truly like magic,” says Kathryn Guarini, vice president of IBM Industry Research. “We’ve learned—we’ve trained an IT system—to learn in much the same way that you and I learn; we learn based on experiences and examples, and that is what we’re using to train a system to be able to then make decisions and drive outputs based on that training information.”
Waters spoke with AI experts from banks, asset managers, vendors, and academia to find real-world use-cases for deep learning, where there are still roadblocks and to better understand what the field’s future evolution might yield. While explainability issues, bias and dataset size will continue to slow the pace of development, it would appear that the dawn of deep learning has arrived in the capital markets—but there are others who believe current approaches are still a bit hit-and-miss.
A Deeper Understanding
Deep neural networks require reams of data in order to produce reliable, actionable outputs. One place where it’s relatively easy to find a treasure trove of data is in communications.
In some ways, it’s truly like magic. We’ve learned—we’ve trained an IT system—to learn in much the same way that you and I learn.
Kathryn Guarini, IBM Industry Research.
Credit Suisse, through its global markets equities research team, decided to drill into its communications data—which, for this proof of concept, included over 3 million emails and 500,000 meeting notes, as well as Bloomberg Chat texts and service tickets—to better understand its clients and their desires, says Paras Parekh, head of the predictive analytics team within the global markets technology group at Credit Suisse.
“Usually banks have a voting process where they proactively reach out to the clients and solicit feedback on how they are doing,” says Parekh, who also oversees the bank’s AI/machine-learning platform. “Based on that, we know how we rank among our peers, but those awards either happen once or maximum twice a year. By mining all the email and communication data, this allows us to, on a near real-time basis, get a sense of what our clients want and desire. This will allow us to serve them better, in terms of better ideas and services.”
The bank is developing a platform that accepts email data in its original form as a text input and the backend algorithms generate the mentioned entities, stock and bond tickers, and Credit Suisse products as an output from the larger text. The deep neural networks then generate a summary of the text and provide a sentiment rating.
“We tried various models, such as random forest and a few other ones, and we obviously wanted to give deep learning a shot because we had enough data to try it out,” he says. “It happens that the deep learning model works fairly well compared to random forest.”
Parekh says the end goal is to have a platform—which, like most platforms that use neural nets, relies heavily on natural-language processing (NLP)—that can do four things: provide entity recognition, sentiment analysis, generate cross-sell opportunities and provide investment ideas.
The project is still in pilot with “a few analysts,” says Parekh, with the aim of a wider release for the first quarter of 2019.
“We’re still fine-tuning the model. It’s giving us enough insight, but there’s still some work needed, especially when we’re looking to find cross-selling opportunities,” he says. “But if we had to look for investment ideas for stocks that the client may be interested in, that’s working quite well. We’re also able to do a fair bit of entity recognition and trying to understand all the various entities involved for an individual or within a group at a client level or a macro level.”
There’s been a fair amount of trial and error as his team has gone down this path. Parekh says that when it comes to sentiment analysis, deep learning works relatively well. He says the sentiment analyzer they developed was trained on financial data, so the accuracy seems to be better than some of the other third-party options available in the market, which are not usually trained specifically on financial data.
The bank also tried to use neural nets to develop an auto-summarization tool for emails and research notes. The idea behind this was that it would take in a large piece of text that contains multiple paragraphs, and it would try and summarize that document into five lines. They used two different models for the experiment. The first was extractive, which would look for the top five, most important sentences from an article. This worked well and the bank is moving forward with the tool, Parekh says.
The second component would try to automatically generate those five sentences—which is not possible with traditional random forests or Bayesian models—but after trying to leverage deep learning for five months, the project stalled.
“Identifying the right use-case is important,” he says. “I think we were looking to stretch ourselves farther given that we had some good success on a couple of models before. And it’s still effort well spent—we learned quite a bit.”
Article continues after the box…
In the Sky
Deep learning’s first big break in the capital markets came in the alternative data space, and one of the biggest breakthroughs in that area has been through satellite imagery.
Alternative data is booming, its rise coming as hedge funds and asset managers are trying to find new sources of information to generate alpha. Satellite information is still in its own early days, but it’s growing rapidly. According to the website alternativedata.org, 29 percent of funds use satellite datasets, though that’s still behind web data, credit/debit card information, social/sentiment datasets, app usage, and web traffic.
Take, for example, Orbital Insight. The satellite analytics platform provides data to firms to, for instance, measure foot traffic at malls in order to assist with earnings predictions or to give commodities traders insights into oil production. So, as a satellite passes over a field of oil tanks, for example, it takes an image and sends it back down to Earth. From there, Orbital Insight collects these images from the satellite companies and runs them through deep neural networks to see if the lids—which float on top of the oil in the tank—are high or low, thus indicating whether they’re over-stocked or in high demand.
“We’re purely a software company using computer vision, normalization, data science, and deep learning neural nets to create insights on a massive scale,” says Ben Rudin, commercial business lead for the vendor.
According to Rudin, Orbital Insight is expanding the number of retailers it tracks and has added geolocation data to its offering so that it can layer these individual datasets on top of one another to create a more holistic understanding of a retailer’s footprint or performance. For example, for its consumer dataset, it can combine its car-counting dataset along with its geolocation data to provide a more informed signal.
“The number of images that we are getting of Earth is increasing exponentially,” Rudin says. “Five years ago, you were getting images every once in a while; today, you are getting imagery of the entire Earth’s landmass daily. It’s not a theory that we’re hoping for, it’s a reality that we are capturing the Earth’s landmass daily.”
All the Pieces Matter
For almost anyone who loves puzzles, Tetris is in the pantheon of greatest video games. And experienced data scientists have the opportunity to apply new technologies to old games.
Deep learning is really good for very large datasets that have stable relationships, which is actually a lot of the time not the case for us.
Norman Niemer, UBS Asset Management.
Norman Niemer, the chief data scientist for UBS Asset Management’s quantitative evidence and data science team, decided it would be fun to build a deep learning-based robotic agent that could learn how to play Tetris just by “watching” the game being played on a computer screen.
So, for the experiment, there was no human actively teaching the bot how to play; rather, the deep-learning model would learn to play the game just by seeing the pixels on the screen and then it would teach itself the best movements for the Tetris pieces to achieve the best score.
Over the course of a weekend, Niemer combined a mix of Python with Google’s open-source library TensorFlow and coded it with deep reinforcement learning formulae.
“In Tetris, normally the blocks just move down in a straight line,” he says. “I introduced some randomness in the way the block moves to see how the agent reacts to changes. I also introduced other ways of scoring points, such as by collecting bonus points by moving along the bottom while waiting for the block to fall.”
The project was a success, so he decided to try out the bot on trading. Over the course of a weekend, he built another experiment. Unfortunately, for trading, the bot was not effective.
“Even if you introduce some randomness in the game to have the blocks move around randomly—which is a little bit closer to what a trading environment would be like—it very quickly learned what to do. I was like, ‘Wow, this is great! Let me try and use this for trading stocks.’ I specifically used it for pairs trading and the machine, basically, wasn’t able to learn anything because the data is way too noisy and the so-called rules of the game change all the time,” he recalls. “So, after including transaction cost, the agent basically said that the best thing to do was to not do anything.”
Niemer’s team is encouraged to attend conferences and hackathons to learn about new technologies and experiment with what they learned to help improve the bank’s ability to leverage new datasets and data science techniques, so as to improve the investment decision-making process. He says deep learning is probably more useful for systematic investing where you have a lot of cross-sectional data with long histories. But for discretionary fundamental investing, for example, to help a fundamental analyst forecast sales, it’s often not the best tool.
“For us, it’s a matter of what are we trying to do—what does the data look like, and what’s the best tool for the job?” he says. “Typically, what the data looks like heavily informs what the best tool for the job is. Deep learning is really good for very large datasets that have stable relationships, which is actually a lot of the time not the case for us. Even for stock prices with longer histories where, in theory, deep learning might be useful, because the relationships change all the time and neural nets aren’t always the best at picking that up—especially in a robust way—that is sort of the downside.”
The size of the dataset is key in deciding the best tool to use, at least at the start. Then it’s a question of how best to visualize the data. So, Niemer says, if it’s something like a linear relationship, it’s probably best to stick with a linear model.
But for something that looks at the behaviors of different investment professionals, a linear model clearly won’t work, as the data points are extremely clustered because the human decision-making process functions much more like a decision tree type of model. So, just by looking at the dataset, it can be fairly obvious that deep learning isn’t the best choice. It’s an iterative process of trial and error, however.
“The jury is still out,” Niemer says. “Sometimes it’s not even clear if it outperforms a simple linear model, or a tree model. Sometimes it’s a little bit better; sometimes it’s a little bit worse. I think where a lot of people are right now is that a tool that is a little more complex than a simple linear regression, but not as complex as a deep learning net, is probably good enough for now.”
But advancements are being made to improve the viability of deep learning models in finance.
The Great Race
The race for deep learning supremacy has been heating up, with the largest tech companies in the world all looking to carve out their claims. Take IBM for example, which has been aggressively seeking breakthroughs in the time it takes to digest and analyze massive datasets and improve the explainability of deep neural nets.
AI is likely to present some challenges in the area of opacity and explainability.
Lael Brainard, Federal Reserve.
“If you think about all the ways that you interact with systems today that deal with speech, language and vision, most likely deep learning is the technique—and neural networks—behind them that is powering those kinds of capabilities,” says John Smith, manager of AI tech for IBM Research AI at the IBM TJ Watson Research Center. “Where deep learning has really excelled is on understanding of unstructured data, such as text, speech, and vision.”
It’s been a cocktail of advancements that have made deep learning more viable over the last three to five years. The ability to process huge datasets has improved, while the cost of doing so has gone down significantly. Thanks to public cloud providers like IBM, the ability to store huge datasets has also dramatically improved. Furthermore, the amount of data available—often called alternative data in the capital markets to distinguish it from market and reference data—has exploded. And on the academic side, the design of neural nets has become more sophisticated and, as a result, they are becoming more powerful, which makes them more enticing for investment firms to experiment with.
But for all the advancements, if it’s not possible to explain how a neural network arrived at its result, it limits what deep learning can be used for.
“This isn’t something that’s unbridgeable,” Smith says. “They are making deep learning models and neural networks more interpretable and more explainable. It’s a requirement that we’re all aware of, but one in which there’s still a lot of progress happening.”
Article continues after the box…
Examining the Unexplained
Generally, a normal algorithm can be explained by examining the code itself. For deep learning, the algorithm is written in run-time, and thus cannot be explained, though the idea that it’s a complete black box is a bit overstated. Much like how a neurologist can see which parts of the human brain “light up” under scans when making a decision, so too can a data scientist see which parts of a neural network are lighting up via visualization when it produces an output. As a result, you know exactly what it was looking at when it made a decision.
“A lot of people say advanced machine-learning models are not as easily explainable as a linear regression,” notes UBS’s Niemer. “While that’s technically true, I have not had many problems explaining advanced models. For a more complex model, I’m not trying to say, ‘Hey, here is exactly how the math works,’ it’s more about understanding how the system behaves and how it makes decisions. For tree models, you can still get good insights into what features drive a decision and how. Even for deep learning nets, for applications like autonomous driving, it can show you on a real-time basis where the machine-learning model is looking at in the picture. So as a car is driving, it highlights a stop sign or the tires of another car so you can see what the machine is paying attention to, and that goes a long way in terms of helping people to understand how the machine behaves.”
That can be extended, in time, to uses in finance. The reason why IBM and Google, such as with its TensorFlow Embedding Projector, are taking aim at the explainability issue—in finance, anyway—is because they want to win business from banks and asset managers, but it is also thanks to the fact that regulators are increasingly taking notice of this interpretability shortcoming.
Lael Brainard, a member of the board of governors of the Federal Reserve System, gave a speech on November 13, 2018, in which she talked about the increased use of AI—which she liberally used to encompass all forms of machine learning—in financial services, noting that “AI is likely to present some challenges in the area of opacity and explainability,” and that, “recognizing there are likely to be circumstances when using an AI tool is beneficial, even though it may be unexplainable or opaque, the AI tool should be subject to appropriate controls,” and that goes for both in-house proprietary tools and third-party models.
While no rule exists that was written specifically for the application of machine-learning techniques in financial services, SR 11-7, which was issued by the Federal Reserve and the Office of the Comptroller of the Currency (OCC), does address model risk management and could be used as the starting point to address the use of AI in risk modeling, even though it does not contain any direct references to machine learning, as was noted in a recent story published by WatersTechnology’s sibling publication, Risk.net.
Beth Dugan, the deputy comptroller for operational risk at the OCC, told Risk.net that SR 11-7, which is a principals-based rule, is general enough that their “existing model risk management covers it,” and that, “Machine learning can actually fit very neatly under that.”
Stressed
A key reason why regulators are taking a greater interest in machine learning generally is because these techniques are increasingly being used for regulatory reporting and stress testing and deep learning is the next frontier.
Take, for example, the Comprehensive Capital Analysis Review (CCAR), an annual exercise by the Fed, which is designed to assess whether or not “the largest bank holding companies operating in the US have sufficient capital to continue operations throughout times of economic and financial stress, and that they have robust, forward-looking capital planning processes that account for their unique risks.”
Deep learning could prove useful for stress testing, says Neeraj Hegde, a quantitative trading architect at Societe Generale, because it can handle the large swaths of data needed to run these tests. The implementation of CCAR is very complicated because there are so many different categories of risk, and the interrelationship of the risks itself is complex, he says. Additionally, in theory, deep learning can help to remove model bias.
“Normally, when you are testing for risk, the current models make an assumption on what the risk distribution is going to be,” Hegde says. “If you harken back to the 2007 days, people were talking about fat tails and the distributions used to model risk were not correct. So the intuition being that, with deep-learning models, you don’t necessarily have a pre-conceived distribution of risk; you don’t have a probability distribution that you are trying to model risk around. So it has a better ability to model really wide scenarios—many, many more scenarios.”
Beyond regulatory reporting, explainability issues persist for things like market forecasting and risk modeling.
“On the market side it’s a problem because people who would deploy that model to take risk don’t know exactly the parameters that are going to constrain that risk,” he says. “You’re taking positions on a market projection, but you don’t know what the constraints are on where this is going to project. Say it projects this total crash of S&P futures—and it might be right—but why is it doing that? So you are kind of a bit blind there. Whereas, if the same thing was done in a more traditional statistical model, you would say, ‘OK, we are going to reverse engineer this and go back to see which variables in the data caused the projection to go there.’ It might be complex, but it’s doable.”
But there are still unique benefits that deep learning can potentially deliver, hence the continued experimentation.
Take, for instance, traditional equity factor models that are used for measuring portfolio risk. These factor models are driven around correlation, Hegde notes. The biggest data companies in the space, such as MSCI Barra, have over a dozen factors on offer, which is excellent. But more correlated factors can lead to better portfolio risk modeling.
“With deep learning and big data, now, there is the ability to expand on that and come up with many more factors. The problem there being that [MSCI] Barra’s factors are very nice and explainable in English for [say], momentum, volatility, etc.—things people understand,” Hegde says.
But, again, he warns that for deep-learning models, the factors that come out are never explainable, which leads to uncertainty and distrust. Therefore, this is still highly conceptual and forward-thinking, as opposed to reflective of reality.
Jie Chen, managing director for corporate model risk at Wells Fargo, says that the bank has done a lot of testing of deep learning because it is capable of modeling and analyzing unstructured data and complex problems at speed and scale. This has certainly been seen, she says, for image or speed-pattern recognition, autonomous systems, and recommendation systems. And these advancements have led to improved services on the retail side of the bank.
“Deep learning is able to handle different learning problems, including supervised learning, unsupervised learning or reinforcement learning,” she says. “The applications of deep learning in our bank include but are not limited to, chatbots, complaint analysis, natural language processing, anomaly detection, et cetera. Moreover, deep learning can help extract the time-series features from histories via a recurrent neural network or a convolutional network, which are typically manually extracted from historical records in the banking industry.”
She says that there’s still much need for growth, though, before it becomes pervasive throughout the entirety of the bank. When modeling structured data, for instance, in most cases traditional machine learning algorithms are usually sufficient. But for raw time-series inputs, deep learning may prove to be better.
Additionally, to help push the evolution of deep neural networks, the bank is working to address issues of explainability. “We are working on explainable neural networks (xNN) imposing structure into the neural network model,” Chen says. “With xNN, the treatment of the input variables and their relationship to the output variable is clear to the user and explainable to others. At the same time, the xNN is able to take advantage of large datasets and large numbers of variables in the same way as other neural networks, whereas interpretable traditional statistical regression models cannot.”
Teaming Up
I think it’s going to take the industry some time just to get comfortable with deep learning and understand the pros and cons.
Andrew Chin, AllianceBernstein.
While some banks like Wells Fargo are actively engaging in the experimentation and actual evolution of deep learning, another reason for its slow development—at least when compared with other AI derivatives like NLP, robotics or even simple decision trees—is that in the vendor space, it’s still just the largest tech giants pushing for breakthroughs. A tipping point will come, but we’re not near there, it would seem.
AllianceBernstein (AB), which manages over $550 billion, uses a fair amount of machine learning techniques in its investment process. Andrew Chin, chief risk officer and head of quantitative research at AB, says that in some ways, using deep learning can be like having a hammer and looking for a nail. He says that “some of the harder techniques” won’t bear fruit for a few years.
“Simple regressions work a lot of the time and then we would introduce machine learning to see if we can get a better model, and usually we can—random forest, for example, is a popular technique that seems to yield interesting results,” he says. “I think it’s going to take the industry some time just to get comfortable with deep learning and understand the pros and cons. If we apply it to one problem, we might not understand the nuances as it applies to different problems. So I think the industry is still working through that.”
Chin says that he has spoken to some of the largest tech companies in the space about potential partnerships with the asset manager, but roadblocks are usually hit because the vendor doesn’t have enough domain expertise when it comes to the capital markets and asset management. But improved relations will come.
“They’re getting better, but they’re more focused on retail finance. So they know the private wealth space well, but for problems relating to investment management they don’t know that well—yet—but they’ll get better,” he says.
“I suspect that we’ll have to find a way to see how we can combine that technical expertise with the domain expertise that the industry has. I don’t know what the right model is—whether it’s some of those folks working with us directly or whether they hire some of us into that firm. I don’t know what the right model is but there has to be a better partnership between the two of us.”
For the time being, innovation will continue to trickle down from the largest firms. Gurvinder Singh, CEO, and founder of Indus Valley Partners, notes that there’s a lot of dead money in the deep-learning space, meaning you’re throwing a lot of capital at something that has a high likelihood for failure.
IVP, through its product offering and consulting services, incorporates many forms of AI into its suite of solutions. Internally, they lean heavily on things like NLP, robotic process automation and more traditional forms of machine learning. But it’s only through the company’s consulting services that they dabble in deep learning. And for those making big strides using deep neural networks in the investment process, they’re not likely to give any of those secrets away anytime soon.
Singh estimates that 80 to 90 percent of these experiments fail and, as such, investment in these projects need to be kept in check: He puts the number roughly at $40,000 to 50,000, but should not extend into the millions of dollars.
These are tough barriers and they will keep smaller companies away from building true deep neural networks because even when successful, it’s very hard to productize the wins and sell the solution at scale.
“I’m not sure how viable it is to even productize a lot of those things, at least in the near-term. Perhaps in the future some of these will get packaged out,” but that’s still a ways off, Singh says. “If you look at how our products have developed over the years, our consulting teams really have been the early adopters, experimental with data science. And once we have seen a pattern of three or four clients consistently finding value in a certain thing, then we start looking at productizing some of the learnings from there to see if it’s possible.”
And even the biggest tech and data companies in the market are taking it slow with deep learning.
Gary Kazantsev, head of machine-learning engineering at Bloomberg, says that his team of about 40 people work on three different areas of development. The first area looks at natural language understanding in the context of question answering on the Bloomberg Terminal. A user types in “chart price of Apple, Google, and Microsoft since 2000,” and, voila, the chart appears.
The second sector is for natural-language understanding in the context of financial markets, where they build indicators such as sentiment analysis, market impact predictions or clustering. So, for example, Bloomberg provides sentiment analysis for companies, for various commodities—in the future, it will do it for foreign exchange—for news, for social media and even produce sentiment findings in multiple languages.
The final piece is product incubation, which is open-ended exploratory work for idea generation. For it, the team uses everything from very simple things like linear regression all the way up to very modern applications of deep neural network embeddings, which can turn text into numbers.
“For a lot — and I do mean a lot — of client-facing applications, we stick with traditional machine-learning algorithms,” Kazantsev says. “Usually, our client-facing applications are ensembles of simpler models, such as support vector machines. It is mostly in the background that we use deep learning - and the models that we use are not really very ‘deep,’ per se.”
Not to beat a dead horse, but the reason for this, he explains, is deep learning’s lack of interpretability. They have to be able to describe to clients how the platform delivers its outputs. So for something like sentiment analysis, it can involve speech tagging, named entity recognition, parsing, and disambiguation into a taxonomy of entities. All of those sentiment prerequisites, he says, involve neural networks, they’re just not very deep and can be satisfactorily explained to a client or—if need be—a regulator.
But, again, improvements are being made.
“People are working on this very actively, and that is a reason why we can start working on deep neural nets for some client facing applications,” Kazantsev says. “For instance, our most recent work on market impact does use neural networks. But that model has rather different properties from [say] sentiment analysis—it is grounded directly in market behavior and can use, essentially, an arbitrary amount of data.”
Dawn of the Machines
Robert Huntsman, head of data science for consultancy Synechron, has been working with clients on all manner of machine learning-based projects. He says that it’s easy to see how deep neural nets could one day become ubiquitous in the capital markets, even though it will take time.
The applications of deep learning in our bank include, but are not limited to, chatbots, complaint analysis, natural language processing, anomaly detection, etc.
Jie Chen, Wells Fargo.
Deep learning could be used to predict volatility and stock prices, or to predict which customers will be their best/worst customers over a defined time period, or to predict which assets will become illiquid in the future, or how volatile a portfolio will be in the future. There are experiments that already exist for these—it’s more a matter of when, than if, it will happen.
“The key advantage is that neural networks are not pre-defined,” Huntsman says. “It’s much less structured than a traditional type of classification model or regression model. When you’re trying to predict [say] any type of value and you’re using traditional machine learning, you typically are using models that are mathematical and very good at handling linear relationships. But these models are structured, they’re mathematical to the form of the model that’s already done. You’re just basically finding the best parameters for that model. Deep learning makes no assumptions about what those relationships will be.
There’s a certain amount of flexibility there: you can have 10 nodes or 100 nodes; you can have three layers or six layers; you can have all kinds of variations in how it’s weighted. “But at the same time, its flexibility is also what makes it extremely difficult to use in any sort of regulated environment—there’s no way that, right now, you’re going be able to file a neural network as a credit-risk file,” due to explainability constraints.
To Huntsman’s point, these emerging tools could also usher in a new wave of investment opportunities for illiquid instruments. One example is in the bond market, which has always struggled with ways to incorporate new technologies to find new avenues for generating liquidity.
“One of my favorite topics—but it’s still very theoretical—is bond-price prediction: that’s where I foresee deep neural networks being useful and that’s where I want to use them,” says Usman Khan, co-founder and chief technology officer for bond-trading startup Algomi.
The way he sees it, you get data points around the price of a bond in terms of how it’s executed: Was that execution influenced by human relationships? Was it actually the result of the attributes of the bond based upon its maturity, its duration? What basis was it priced is a question you first ask, but then what should be the right basis? What is the right way of pricing a bond?
“Once you solve for that, then I want a machine to be able to implement that and price those bonds in accordance to the right pricing approach,” Khan says. “That takes so many different things into account; it has, like, hundreds of feature vectors. I believe deep learning can definitely be used for that. Once you have all the structured data from all of these different places, and I’ve got access to the venues, access to illiquid bonds and how they’re being traded, I have access to voice data—if I have access to all of that and I’ve come to a conclusion as to how to price a bond properly, then I want my machine to do the rest because there are millions of bonds.”
And beyond that, deep learning could underpin the trading experience of the future.
As noted before, rarely is deep learning an island; it’s used in conjunction with other forms of AI techniques and technologies, such as cloud. When combined with augmented and virtual reality, when coupled with chatbots and bleeding-edge visualization tools, deep neural networks could be the veins that connect—and make connections from—the sea of information flowing into a trader’s environment. But now we’re getting ahead of ourselves. Baby steps are still needed.
“In financial services, we’re still at the early days of deploying these AI and deep learning systems in production,” says IBM’s Guarini. “There’s a lot of experimentation going on, for sure. There’s a lot of potential, but in terms of wide-scale deployments for decision support, for identifying fraud, and more, these are still in their infancy. What I foresee over the next two years is that we’ll have more robust ability to ensure regulatory compliance, to be able to explain decisions and results, to be able to have confidence that the AI solutions here are appropriate to deploy for these different applications and then they’ll be done so at scale.”
The evolution is here, but there’s still a long way to go.
Only users who have a paid subscription or are part of a corporate subscription are able to print or copy content.
To access these options, along with all other subscription benefits, please contact info@waterstechnology.com or view our subscription options here: http://subscriptions.waterstechnology.com/subscribe
You are currently unable to print this content. Please contact info@waterstechnology.com to find out more.
You are currently unable to copy this content. Please contact info@waterstechnology.com to find out more.
Copyright Infopro Digital Limited. All rights reserved.
As outlined in our terms and conditions, https://www.infopro-digital.com/terms-and-conditions/subscriptions/ (point 2.4), printing is limited to a single copy.
If you would like to purchase additional rights please email info@waterstechnology.com
Copyright Infopro Digital Limited. All rights reserved.
You may share this content using our article tools. As outlined in our terms and conditions, https://www.infopro-digital.com/terms-and-conditions/subscriptions/ (clause 2.4), an Authorised User may only make one copy of the materials for their own personal use. You must also comply with the restrictions in clause 2.5.
If you would like to purchase additional rights please email info@waterstechnology.com
More on Data Management
New working group to create open framework for managing rising market data costs
Substantive Research is putting together a working group of market data-consuming firms with the aim of crafting quantitative metrics for market data cost avoidance.
Off-channel messaging (and regulators) still a massive headache for banks
Waters Wrap: Anthony wonders why US regulators are waging a war using fines, while European regulators have chosen a less draconian path.
Back to basics: Data management woes continue for the buy side
Data management platform Fencore helps investment managers resolve symptoms of not having a central data layer.
‘Feature, not a bug’: Bloomberg makes the case for Figi
Bloomberg created the Figi identifier, but ceded all its rights to the Object Management Group 10 years ago. Here, Bloomberg’s Richard Robinson and Steve Meizanis write to dispel what they believe to be misconceptions about Figi and the FDTA.
SS&C builds data mesh to unite acquired platforms
The vendor is using GenAI and APIs as part of the ongoing project.
Aussie asset managers struggle to meet ‘bank-like’ collateral, margin obligations
New margin and collateral requirements imposed by UMR and its regulator, Apra, are forcing buy-side firms to find tools to help.
Where have all the exchange platform providers gone?
The IMD Wrap: Running an exchange is a profitable business. The margins on market data sales alone can be staggering. And since every exchange needs a reliable and efficient exchange technology stack, Max asks why more vendors aren’t diving into this space.
Reading the bones: Citi, BNY, Morgan Stanley invest in AI, alt data, & private markets
Investment arms at large US banks are taken with emerging technologies such as generative AI, alternative and unstructured data, and private markets as they look to partner with, acquire, and invest in leading startups.