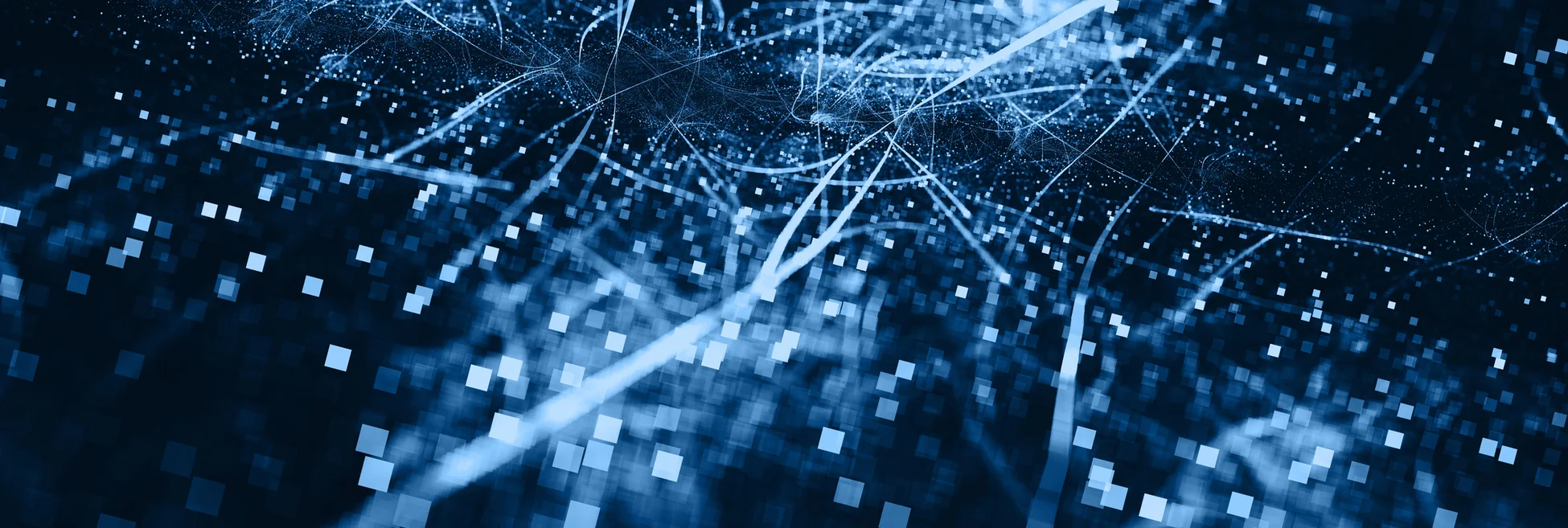
Deutsche Bank leverages AI for securities services
The bank’s client segmentation offering creates more targeted post-trade offerings, while its prediction engine can help reduce settlement failures.
Artificial intelligence is employed throughout the financial services industry—whether it’s to address trade execution issues, forecast returns in non-linear relationships, or better digest sentiment analysis; it’s used in the front office by portfolio managers or by exchanges targeting disclosure gaps. But executives at Deutsche Bank Securities Services, which sits under the bank’s corporate bank division, believe that more can be done with AI in the post-trade industry.
The division currently uses AI in two ways. The first is in client segmentation, which allows DB Securities Services to improve and tailor its offering for clients. The second use case helps it predict settlement failures.
First, the team drills down to individual clients’ requirements and determines what their future needs might be. Marcus Storm, head of AI products for product management at DB Securities Services, tells WatersTechnology that this involves taking apart client activity.
“To know the clients and what they’re doing with their hundreds—sometimes thousands—of accounts, in any organization with this much data available per account, the ability for account managers to crunch the huge volume of data in their accounts is very low. So almost by definition, you don’t have a good overview of what the clients are doing and what their future needs might be,” he says.
Machine learning algorithms allow the bank to automate that process. DB Securities Services uses classification-based algorithms, which classify or categorize data into distinct groups to reflect their similarities even if they are outside traditional client segments. Traditional client segments include global custodians, broker-dealers, insurance, traders, and various types of funds.
“We use algorithms to do that, and therefore we can take that high-level overview—whether you’re an ops or business development executive—and really figure out how we should categorize them. This helps us figure out our future product roadmap to serve these segments, and in the long-run, clients will get a better service,” Storm says.
One such example looks at client balances. “We see pockets of liquidity, which we think clients could put to better use,” he says. “If you think about it, how many accounts have how much liquidity in them that could be optimized?”
To facilitate this, DB Securities Services is working on a project to figure out a roadmap to encourage clients to deploy the liquidity in their interests. This would have been harder to achieve in the past, as taking that data out of various systems manually could have taken up to three months.
AI-driven client segmentation is also used to detect fraud and improve internal risk controls. Storm says DB Securities Services is applying the same principle of looking at all those client accounts and their activity over time, but now the bank doesn’t have to spend thousands of hours manually sifting through the data.
Boon-Hiong Chan, global head of securities market development and technology advocacy at DB Securities Services, says the client segmentation offering has shortened the time required to do analysis through proactive profiling.
“Through this profile, you can anticipate certain requirements, versus trying to construct the behavior from historical data points from scratch, which will take more time,” Chan says.
Getting to the root
Another way DB Securities Services is using AI is to predict settlement failures.
In 2019, the team began working on S2-Predict to tackle the challenge of determining the root cause of settlement failures. It uses historical failure-rate patterns and trends globally, providing a benchmark and insight for clients to judge current outcomes.
S2-Predict is based on an algorithm that uses supervised learning and is trained with historical data and calibrated with real-time information to detect anomalies. The system also monitors the status of in-flight transactions and provides the probability of failure in each case. It can also examine hypothetical transactions and estimate the chance of failure, with a breakdown of factors contributing to that risk.
According to Storm, the system’s response time is “less than a third of a second.”
“So you submit a trade instruction to us, and you’ll get a response right after you click it. You’ll get a prediction as to whether your trade instruction will fail or not. And that’s so powerful, because you can fix things quickly and it will have a huge impact on your ability to increase your settlement rate,” he says.
On top of that, the system can explain why a settlement is predicted to fail. Storm says S2-Predict uses a neural network to provide predictions. Then, another neural net effectively tells the user why the settlement is predicted to fail.
“That allows us to take that integration a step further. It depends on what type of client we’re talking about, but if [the instruction is handled internally, and not by the client] once we find out the predicted failure, we can verify that automatically. So, for example, if you submit a trade and your account balance isn’t enough to complete the trade, then that simple logic check can be integrated in as well. And specific alerts or specific action points can be taken to remediate that,” he explains.
Chan adds that with the prediction, operators can immediately identify false positives, too.
“Using the insufficient balance example, if there’s an insufficient balance, is it due to a timing difference, and there are stocks or cash coming in to facilitate settlement, and therefore it would be a false positive? Or is it really a positive? In that case, clients and our operations team will have at least more time to prepare, for example, to borrow or to cover the shortfall,” he says.
DB Securities Services aims to have S2-Predict in production in a few European markets later this year. Chan says Europe is the priority for the tool because of the availability of real-time post-trade settlement information. Also, there’s a regulatory push from the Central Securities Depositories Regulation (CSDR) to avoid settlement failures.
Meanwhile, in Asia, Chan says more work needs to be done with the CSDs to make post-trade information available on a real-time basis for real-time analytics.
“There are only a few Asia markets now—i.e., Singapore and Hong Kong—that provide such information, which is valuable to help mitigate settlement failure. We are testing internally in these markets. Batched post-trade settlement information with complete historical quality data overlaid with good data analytics can still deliver a level of benefits, although results would assume more historical weightage to underlie today’s flows,” he explains.
A fit-for-purpose data platform
None of the work that DB Securities Services has put into its client segmentation and S2-Predict offering would be possible without what Storm calls its “solid data foundation.”
“I have to emphasize that this enables any AI data offering in the first place, or analytics offerings. You can’t have that without a comprehensive, business-specific, global data platform. If you have a legacy setup, for example, and you have dozens of different data sources. If you’re going to conduct one AI pilot, it’s going to take at least six months in that sort of organizational setup. So it’s really important to have a solid data foundation upon which you can build your new products and offerings,” says Storm.
DB Securities Services started building a unified data warehouse about four years ago. This involved putting together its hundreds of data stores in one place for data reporting, analytics, and eventually AI. Storm says firms haven’t laid the groundwork that allows for future exciting developments without this type of initial investment.
Now, DB Securities Services has over 1,000 different data sources in that data warehouse and a “huge amount” of business-ready data available. Currently, the data warehouse is in a Hadoop/Cloudera stack, but DB Securities Services will be migrating it across to Google Cloud due to the bank’s strategic partnership with the tech giant.
From a data perspective, DB Securities Services is focused on three things. The first is real-time data streaming, which has resulted in it building a real-time data stream and search capability. Then, that stream goes into the data warehouse. Chan stresses that the warehouse has data governance that identifies and structures the data to understand which data is relevant for which use case.
“Informed data governance/management is key, especially as we apply it at an enterprise level, where all the data across product lines and business lines needs to be consistently structured to allow ‘re-usable’ data to support better cross-pollination of ideas and opportunities between divisions. If data is classified in silos and not consistently structured internally, the data would be less meaningful beyond Securities Services unless more effort and additional costs are put forward to ‘clean up’ the data,” he says.
The third part is where DB Securities Services runs the data internally to understand the relationships and ensure that it is commercially accurate and fit for purpose to be used before it is rolled out externally.
“To build value onto these data relationships, we run them through operations and business colleagues to identify API use cases or other business use cases that can benefit clients. This process and additional data points are important to us to continuously improve our AI-driven, ‘intelligent’ product roadmap,” he says.
Further reading
Only users who have a paid subscription or are part of a corporate subscription are able to print or copy content.
To access these options, along with all other subscription benefits, please contact info@waterstechnology.com or view our subscription options here: http://subscriptions.waterstechnology.com/subscribe
You are currently unable to print this content. Please contact info@waterstechnology.com to find out more.
You are currently unable to copy this content. Please contact info@waterstechnology.com to find out more.
Copyright Infopro Digital Limited. All rights reserved.
As outlined in our terms and conditions, https://www.infopro-digital.com/terms-and-conditions/subscriptions/ (point 2.4), printing is limited to a single copy.
If you would like to purchase additional rights please email info@waterstechnology.com
Copyright Infopro Digital Limited. All rights reserved.
You may share this content using our article tools. As outlined in our terms and conditions, https://www.infopro-digital.com/terms-and-conditions/subscriptions/ (clause 2.4), an Authorised User may only make one copy of the materials for their own personal use. You must also comply with the restrictions in clause 2.5.
If you would like to purchase additional rights please email info@waterstechnology.com
More on Emerging Technologies
This Week: Startup Skyfire launches payment network for AI agents; State Street; SteelEye and more
A summary of the latest financial technology news.
Waters Wavelength Podcast: Standard Chartered’s Brian O’Neill
Brian O’Neill from Standard Chartered joins the podcast to discuss cloud strategy, costs, and resiliency.
SS&C builds data mesh to unite acquired platforms
The vendor is using GenAI and APIs as part of the ongoing project.
Chevron’s absence leaves questions for elusive AI regulation in US
The US Supreme Court’s decision to overturn the Chevron deference presents unique considerations for potential AI rules.
Reading the bones: Citi, BNY, Morgan Stanley invest in AI, alt data, & private markets
Investment arms at large US banks are taken with emerging technologies such as generative AI, alternative and unstructured data, and private markets as they look to partner with, acquire, and invest in leading startups.
Startup helps buy-side firms retain ‘control’ over analytics
ExeQution Analytics provides a structured and flexible analytics framework based on the q programming language that can be integrated with kdb+ platforms.
The IMD Wrap: With Bloomberg’s headset app, you’ll never look at data the same way again
Max recently wrote about new developments being added to Bloomberg Pro for Vision. Today he gives a more personal perspective on the new technology.
LSEG unveils Workspace Teams, other products of Microsoft deal
The exchange revealed new developments in the ongoing Workspace/Teams collaboration as it works with Big Tech to improve trader workflows.