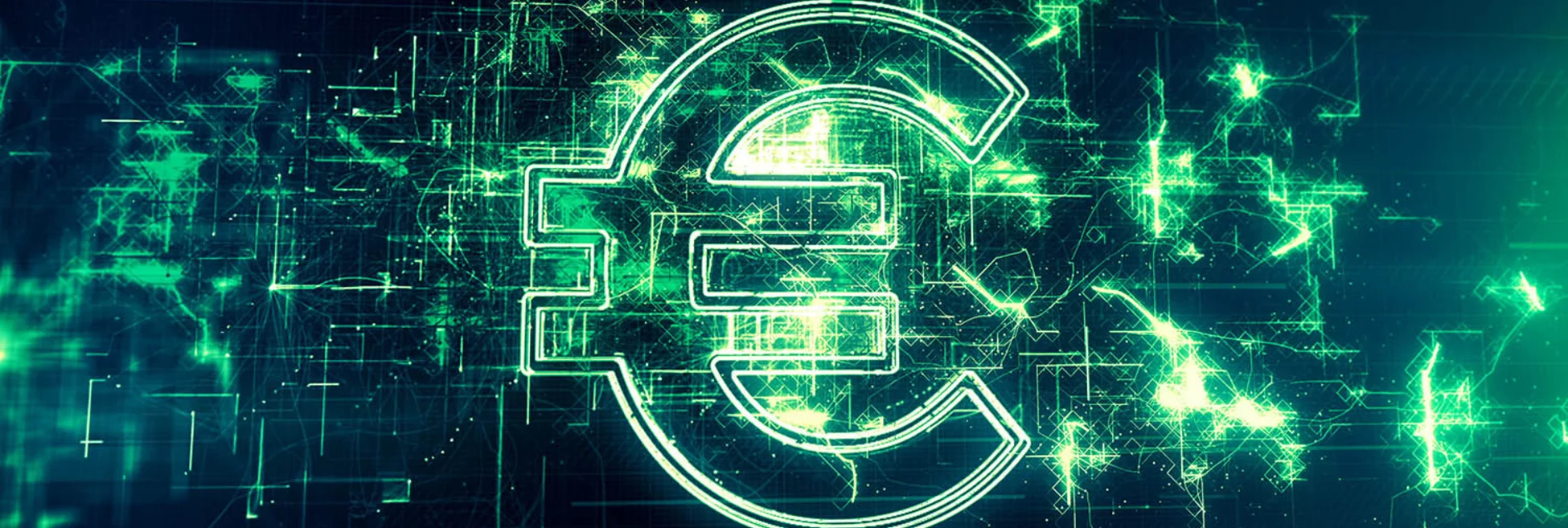
EBA to consult on banks’ machine learning use
The watchdog will set out a new stance on ML-based capital models at a time of conflicting guidance from other supervisors.
European regulators are to consult on banks’ use of machine learning (ML) techniques to calculate credit risk capital requirements—a move that some hope will correct perceived imbalances in the way that national regulators apply existing regulations, which some claim has led to an uneven playing field.
The European Banking Authority is working on a discussion paper to be followed by a consultation on the use of ML techniques. The focus will be on internal ratings-based (IRB) models used to calculate regulatory capital requirements for credit risk. The paper is expected to be published by the end of 2021.
“The aim of the discussion paper is to open a discussion on opportunities and challenges introduced by using more advanced machine learning techniques in the space of IRB models. This would encourage an appropriate use of such techniques and ultimately [mean] that the outcome—in terms of setting capital requirements in a prudent manner—continues to be harmonized across Europe,” says Roberta De Filippis, policy expert in prudential regulation and supervisory policy at the EBA.
The move comes as global regulators look to tighten existing guidance on the use of ML techniques, amid the growing complexity and attendant lack of ready explainability of certain approaches being adopted by banks. This is something that regulators are increasingly arguing makes more complex approaches inappropriate for applications that have a direct bearing on banks’ dealings with customers.
Although the second Capital Requirements Regulation (CRR) does not explicitly prohibit the use of ML-based models, some national regulators are understood to have taken the view that such techniques should not be applied to risk-based capital calculations.
According to banks, CRR Article 179(1)a, which covers rules for estimating credit risk capital using IRB models, contains language that some banks say supervisors have taken an extremely conservative view of. The clause states that estimates of credit risk “shall be plausible and intuitive and shall be based on the material drivers of the respective risk parameters”.
A machine interprets a report in the same manner every time, but if you give a report to 10 different analysts, you get 10 different opinions
Head of ratings methodologies at a large European bank
A large European bank says it has explored the use of machine learning-based models for text mining creditors’ annual reports to determine their probability of default. By automating the ratings process, the bank hoped to reduce inconsistencies in human judgement.
“A machine interprets a report in the same manner every time, but if you give a report to 10 different analysts, you get 10 different opinions,” says the head of ratings methodologies at the bank.
However, the bank was limited in the extent to which it could make use of the full potential of this approach by its national regulator’s narrow interpretation of CRR, it claims.
“A full-fledged AI system—i.e., self-learning without human supervision—would not be allowed today in Europe with the application of IRB for capital purposes,” says the head of ratings methodologies.
In order to overcome this obstacle, the bank obtained approval from its national regulator to use the model on condition that its outputs be reviewed by human experts, rather than rely purely on a dynamic, self-learning model. It therefore does not rely on a solely data-driven development, but has kept experienced credit analysts involved, performing sanity checks of the model.
The definition of machine learning is broad, and also includes modeling approaches that have been used for decades to assess the creditworthiness of clients and applied for regulatory capital purposes since the introduction of Basel II.
“[Regulators] are used to seeing ratings models based on logistic regression, and this model isn’t based on logistic regression, but on a random forest approach. So extra effort would be required to demonstrate that such an approach fits into the prudential framework,” says the head of ratings methodologies.
The EBA itself, in a 2020 report, had discouraged the use of ML-based models for regulatory capital purposes on the grounds that it would be difficult for banks’ supervisors and internal auditors to assess their inner workings, or ‘explainability’.
“From a prudential framework perspective, it is premature to consider ML an appropriate tool for determining capital requirements, taking into account the current limitations (eg, ‘black-box’ issues),” the report said.
The EBA discussion paper to be published later this year aims to provide clarity on the question of how national regulators should interpret the Capital Requirements Regulation as it pertains to machine learning. It should prevent situations where banks in one country are given free rein to innovate, while those in another country are not.
A report by the European Banking Federation, a trade association, noted that the adoption of artificial intelligence (AI) for internal models was hindered by the need for supervisors to understand the models, and called for a review of supervisory approaches to the use of AI.
“It is of paramount importance for supervisors to have a full understanding of the technology to assess and approve AI-based internal models,” said the report.
Further reading
Only users who have a paid subscription or are part of a corporate subscription are able to print or copy content.
To access these options, along with all other subscription benefits, please contact info@waterstechnology.com or view our subscription options here: http://subscriptions.waterstechnology.com/subscribe
You are currently unable to print this content. Please contact info@waterstechnology.com to find out more.
You are currently unable to copy this content. Please contact info@waterstechnology.com to find out more.
Copyright Infopro Digital Limited. All rights reserved.
As outlined in our terms and conditions, https://www.infopro-digital.com/terms-and-conditions/subscriptions/ (point 2.4), printing is limited to a single copy.
If you would like to purchase additional rights please email info@waterstechnology.com
Copyright Infopro Digital Limited. All rights reserved.
You may share this content using our article tools. As outlined in our terms and conditions, https://www.infopro-digital.com/terms-and-conditions/subscriptions/ (clause 2.4), an Authorised User may only make one copy of the materials for their own personal use. You must also comply with the restrictions in clause 2.5.
If you would like to purchase additional rights please email info@waterstechnology.com
More on Emerging Technologies
This Week: Startup Skyfire launches payment network for AI agents; State Street; SteelEye and more
A summary of the latest financial technology news.
Waters Wavelength Podcast: Standard Chartered’s Brian O’Neill
Brian O’Neill from Standard Chartered joins the podcast to discuss cloud strategy, costs, and resiliency.
SS&C builds data mesh to unite acquired platforms
The vendor is using GenAI and APIs as part of the ongoing project.
Chevron’s absence leaves questions for elusive AI regulation in US
The US Supreme Court’s decision to overturn the Chevron deference presents unique considerations for potential AI rules.
Reading the bones: Citi, BNY, Morgan Stanley invest in AI, alt data, & private markets
Investment arms at large US banks are taken with emerging technologies such as generative AI, alternative and unstructured data, and private markets as they look to partner with, acquire, and invest in leading startups.
Startup helps buy-side firms retain ‘control’ over analytics
ExeQution Analytics provides a structured and flexible analytics framework based on the q programming language that can be integrated with kdb+ platforms.
The IMD Wrap: With Bloomberg’s headset app, you’ll never look at data the same way again
Max recently wrote about new developments being added to Bloomberg Pro for Vision. Today he gives a more personal perspective on the new technology.
LSEG unveils Workspace Teams, other products of Microsoft deal
The exchange revealed new developments in the ongoing Workspace/Teams collaboration as it works with Big Tech to improve trader workflows.