Four years on, S&P's Kensho buy yields new automation tools, saving decades of manual data analysis
S&P-owned AI tool Kensho is leveraging its parent's massive datasets with its own machine learning to release a series of tools for analysts.
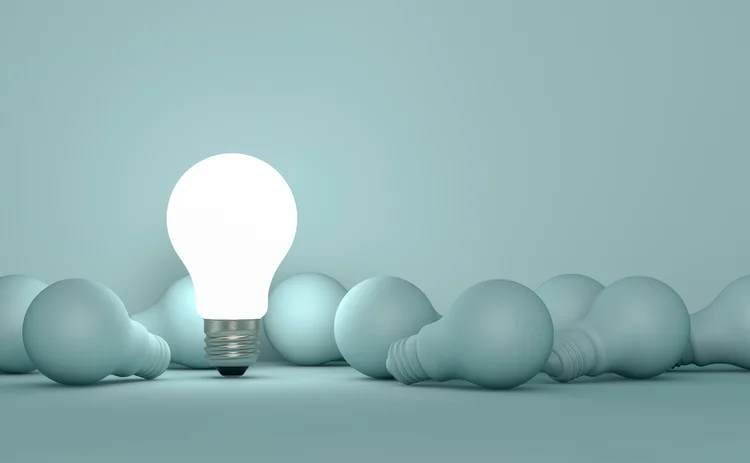
For decades, a team of editors and transcribers at S&P Global pored over transcripts of investor and earnings calls, management presentations, and acquisition calls from some of the most financially relevant companies to bring insights and actionable intelligence to the information giant’s consumers .
Over the years, as technology evolved and approaches like artificial intelligence and natural-language processing began to offer ways to automate data ingestion and cataloging. S&P invested in the Series B funding round of a startup called Kensho, founded in 2013 by Harvard PhD graduate Daniel Nadler, which focused on AI for finance and national security. Then in early 2018, S&P bought Kensho for $550 million.
At the time, S&P said its aim was to become a world leader in AI development with the buy focused on the talent and knowledge from Kensho’s engineers and the ability to infuse its technology stack with natural-language processing, machine learning, and other subsets of AI.
In the period following the acquisition, Kensho’s engineers looked at use cases across S&P where its expertise could be applied to automating tasks.
The first internal use was around data ingestion and the onboarding of company data into databases leading to the development of Kensho Link, a a machine learning capability that links data on companies to companies in S&P’s database. “Kensho Link was developed out of the need for data ingestion internally at S&P,” says Peter Licursi, head of strategic initiatives and partnerships at Kensho. “We quickly realized that externally clients are in great need of leveraging that capability to clean and enrich their databases and more efficiently onboard S&P data into their systems.”
Efforts in the beginning focused on private company data before expanding out. In 2018, Kensho said it could link private company data from private company funding database and news service Crunchbase to data from S&P Global Market Intelligence.
“Because of the massive amount of data that it’s been trained on, the enormous amount of manually curated proprietary data within S&P as an enterprise, we were able to leverage those human efforts to fuel the high-quality AI system that maps messy, erroneous, inconsistent databases to S&P Global’s company database via unique identifiers,” Licursi says. If a company name is incomplete or unclear in a client database, Link allows a user to compare that information with the data found in the S&P Global Capital IQ dataset.
Licursi says that users within S&P said they were able to accomplish in months what they would have once expected to take over a decade with manual work.
Link laid the groundwork for more. The result of S&P’s bet on Kensho so far has been a series of tools geared towards both internal and external client use cases and trained on expansive S&P datasets. One such tool is Scribe, which Kensho first rolled out internally among S&P teams and then in 2020, for clients.
Scribe is based on automated speech recognition technology, which uses machine learning and AI to process raw audio recordings of people talking—for example, an earnings call—and produce transcriptions.
“You’re getting a more accurate result from the transcription because it actually understands terminology that is being used in the context of business and finance,” Licursi says. The training data for Scribe consisted of the hours of transcripts created over the last decade by analysts who manually listened to and transcribed earning calls and management presentations, among other types of events.
In contrast, pre-trained open-sourced models like Google’s Bidirectional Encoder Representations from Transformers (Bert) and OpenAI’s GPT-3 are trained on more general data. When Google published Bert, it supplied the code and downloadable versions of the model already pre-trained on Wikipedia and a dataset called BookCorpus, about three million words in total. GPT-3 was trained on an even more gargantuan dataset—175 billion machine-learning parameters.
Researchers have also worked to specialize open-source models like BERT with models like SentenceBERT, FinBERT and SciBERT. Last year a group of researchers proposed a new model specifically for use in finance called Financial Embedding Analysis of Sentiment (FinEAS).
Kensho has also launched tools called Nerd and Extract. Nerd is an NLP capability for identifying contextual clues, abbreviations, and potential redactions. Similar to Link, users can match unstructured information from regulatory filings, earnings calls transcripts, press releases and the like with the structured information in the massive S&P Capital IQ database. Extract is a machine learning tool trained to carve out hidden data from sources of unstructured data like PDFs.
Kensho is currently developing another tool, Classify, a classification system that labels documents based on topics and themes. The aim is to provide a tool that an analyst could use to organize documents based on themes that interested them, even if those topics change day-to-day.
“We want to bring down the amount of time that people feel like they’re wasting reading irrelevant text and instead help them zero in on the most important content in that text relevant to their work, enabling them to focus their time deploying their analytical skills,” Licursi says.
Kensho’s tools were designed to be used together. An analyst could use Scribe to transcribe an investor’s call and then use Nerd to identify specific entities mentioned n the text. Last year, S&P rolled out a document viewer as part of enhancements in search and analytics to its Capital IQ Pro desktop platform. The viewer is AI-enabled, and combines capabilities from Link, Scribe, and Nerd, allowing for interchangeable use, and delivering better performance than if a firm tried to combine tools from different providers to perform those tasks, Licursi says.
Kensho has open-sourced some of its tech. Its speech recognition dataset, SPGISpeech is available on HuggingFace, a community platform for machine-learning engineers and data scientists. The dataset contains 5,000 hours of transcribed audio related to the financial services industry with two speakers, one a native English speaker and the other a second-language English speaker.
More industries to explore
In November 2020, S&P Global bought financial information provider IHS Markit for $44 billion, creating what many saw as a financial data powerhouse and one of the biggest mergers in recent years, including a wealth of non-financial datasets covering many different industries from the IHS side of the business.
IHS Markit’s chief technology officer and chief data scientist Yaacov Mutnikas told WatersTechnology in August 2020 that the company aims to upload about one million documents published by internal analysts over the past 10 years to its data lake. It was using Google’s Bert to classify and summarize the reports covering topics related to industries like financial services, agriculture, and chemicals, as well as country-specific risks.
Since then, Kensho has been exploring how it could leverage its own tools to derive value from these datasets. S&P CEO Doug Peterson said during the company’s Q2 earnings call that Kensho’s head of AI research, as well as teams of data scientists, machine learning engineers„ and software developers, participated in a hackathon of the data lake this year.
“In addition to finding ways that Kensho solutions can add commercial value to unstructured data sets across the data lake, we identified new data sets for training Kensho Scribe, Nerd, Link, and Extract, more than doubling current training data sets in some cases,” Peterson said.
Only users who have a paid subscription or are part of a corporate subscription are able to print or copy content.
To access these options, along with all other subscription benefits, please contact info@waterstechnology.com or view our subscription options here: http://subscriptions.waterstechnology.com/subscribe
You are currently unable to print this content. Please contact info@waterstechnology.com to find out more.
You are currently unable to copy this content. Please contact info@waterstechnology.com to find out more.
Copyright Infopro Digital Limited. All rights reserved.
As outlined in our terms and conditions, https://www.infopro-digital.com/terms-and-conditions/subscriptions/ (point 2.4), printing is limited to a single copy.
If you would like to purchase additional rights please email info@waterstechnology.com
Copyright Infopro Digital Limited. All rights reserved.
You may share this content using our article tools. As outlined in our terms and conditions, https://www.infopro-digital.com/terms-and-conditions/subscriptions/ (clause 2.4), an Authorised User may only make one copy of the materials for their own personal use. You must also comply with the restrictions in clause 2.5.
If you would like to purchase additional rights please email info@waterstechnology.com
More on Data Management
New working group to create open framework for managing rising market data costs
Substantive Research is putting together a working group of market data-consuming firms with the aim of crafting quantitative metrics for market data cost avoidance.
Off-channel messaging (and regulators) still a massive headache for banks
Waters Wrap: Anthony wonders why US regulators are waging a war using fines, while European regulators have chosen a less draconian path.
Back to basics: Data management woes continue for the buy side
Data management platform Fencore helps investment managers resolve symptoms of not having a central data layer.
‘Feature, not a bug’: Bloomberg makes the case for Figi
Bloomberg created the Figi identifier, but ceded all its rights to the Object Management Group 10 years ago. Here, Bloomberg’s Richard Robinson and Steve Meizanis write to dispel what they believe to be misconceptions about Figi and the FDTA.
SS&C builds data mesh to unite acquired platforms
The vendor is using GenAI and APIs as part of the ongoing project.
Aussie asset managers struggle to meet ‘bank-like’ collateral, margin obligations
New margin and collateral requirements imposed by UMR and its regulator, Apra, are forcing buy-side firms to find tools to help.
Where have all the exchange platform providers gone?
The IMD Wrap: Running an exchange is a profitable business. The margins on market data sales alone can be staggering. And since every exchange needs a reliable and efficient exchange technology stack, Max asks why more vendors aren’t diving into this space.
Reading the bones: Citi, BNY, Morgan Stanley invest in AI, alt data, & private markets
Investment arms at large US banks are taken with emerging technologies such as generative AI, alternative and unstructured data, and private markets as they look to partner with, acquire, and invest in leading startups.