From ChatGPT to BloombergGPT: In capital markets, who can make the most of large language models?
Capital markets are no strangers to hype machines. Among the buzz around generative AI, Nyela wonders who will be best suited to take advantage of the technology and deliver the right use cases to Wall Street.
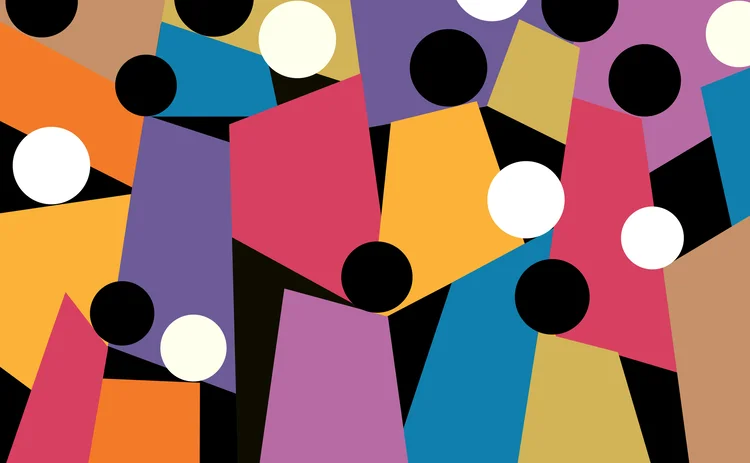
Artificial intelligence’s boogeyman status in the cultural zeitgeist is due in large part, I believe, to James Cameron’s 1984 sci-fi classic, The Terminator. In the film, Arnold Schwarzenegger plays the titular character, a cyborg from the year 2029, who is sent back in time to the 1980s by Skynet, a malicious artificial intelligence network that has gained self-awareness. The Terminator’s mission is to kill a woman named Sarah Connor because her future son, John, will lead a resistance movement against Skynet when it triggers a global nuclear war. Chilling, I know.
Narratives involving intelligent machines controlling, enslaving or terminating humans recur often in literary and theatrical works of fiction, but at least so far, any bleak prophecies espoused by dystopian soothsayers remain unfulfilled. As it stands in the year 2023 (a mere six years before the Skynet doomsday), artificial intelligence has largely useful applications: for instance as a handy assistant, or as a critical piece of technology that helps people automate otherwise arduous tasks and make connections that the human brain can’t—think Apple’s Siri and Amazon’s Alexa.
But 2023 has marked a tide shift, bringing a new AI wave. Thanks to the excitement, hype, and fascination with OpenAI’s ChatGPT chatbot that was rolled out last November, the world can’t seem to get enough of generative AI and the large language models (LLMs) that underpin it.
If you’ve been reading WatersTechnology the last few months, you’ve seen our stories touching on this new wave and what it might bring to capital markets. Currently, OpenAI’s chatbot and LLMs (GPT-3, GPT-3.5, GPT-4) are general purpose, meaning the data and information they’ve been trained on comes from all corners of the internet and they aren’t specialized in anything. It’s currently surmised that the training data includes Wikipedia, Common Crawl data, and a few other corpuses.
I use the word surmised because there is limited information about what the models have been trained on. GPT-3, which was released in 2020, is the last OpenAI model to have any sort of public training information. Since then, OpenAI has made the controversial decision to not disclose the training data and other information that is important in AI research. This is controversial for several reasons, one of which is that the company was originally founded as an open AI research laboratory that would compete with the likes of Google, which uses a closed approach.
Plenty of people are discussing OpenAI’s backpedaling and lack of disclosure, and I’m not looking to add to that discourse at the moment. Instead, I think it would be useful to attempt to unpack this generative AI and large language model hype machine in the context of capital markets and who stands to deliver the best utilization of this technology for Wall Street.
Hype machines
I’m familiar with capital markets hype machines. Last spring, Rebecca Natale and I set out to revisit the initial blockchain mania (2015–2018: gone but not forgotten) and answer the question: Where have all the blockchain startups gone? We went back through the Waters archives and sifted through stories that announced a plethora of banks, asset managers, exchanges and vendors that were keen to add their names to the ever-expanding list of those working with blockchain.
This AI hype bubble we are currently in is similar, in that firms are eager to find use cases for the technology. From a PR and marketing perspective, deploying AI makes firms look smart and cutting-edge. And they want to be familiar with it so they can be prepared when more mission-critical use cases emerge.
That’s what I think makes this different from the hype that surrounded blockchain. Artificial intelligence and its subsets—including machine learning and natural language processing—have already proven they can be applied to capital markets
It’s important to note that artificial intelligence is already widely utilized across capital markets. Just to name a few examples: BNY Mellon has deployed it alongside cloud tools for the back office; Brown Brothers Harriman has built out a suite of AI products; and banks are looking to it for settlement fails.
That’s what I think makes this different from the hype that surrounded blockchain. Artificial intelligence and its subsets—including machine learning and natural language processing—have already proven they can be applied to capital markets. Blockchain has yet to show that. Generative AI, chatbots and their underlying models can build on a foundation already laid.
“You’ve got an interface moment for AI, essentially,” says Matthew Cheung, CEO of Ipushpull, a no-code data distribution platform that offers chatbots. “AI has been around since World War II. It’s been around for a long, long time, but it’s been kind of exponential growth.” He says the pandemic helped speed along the embrace of more interface-oriented technology. For example, communication platforms like Microsoft Teams and Zoom are now essential components of the business day.
Cheung says this is what makes the chat component of chatbots like ChatGPT and Ipushpull’s own offerings appealing. And that increased appeal has put greater focus on what’s under the hood: the models.
Before there was ChatGPT, there was GPT-3. The public reacted strongly to the model and what it showed it could do. Last April, The New York Times wrote of GPT-3 and the moment large language models were starting to have in social consciousness: “Siri and Alexa had popularized the experience of conversing with machines, but this was on the next level, approaching a fluency that resembled creations from science fiction like HAL 9000 from [Stanley Kubrick's 1968 sci-fi movie] 2001: a computer program that can answer open-ended complex questions in perfectly composed sentences.”
As I wrote in January, for capital markets, the impact that ChatGPT and the technology underpinning it could have is not immediately clear since the tech is in what many see as its “first generation.” But as it evolves, everything from the role of the analyst to the way critical infrastructure is built could be disrupted along the way. People I spoke to across capital markets considered the possibility of training this technology on industry-specific data to fit more needs unique to the financial markets.
It turns out that some of that work is already in process.
Fit for purpose
At the end of March, data behemoth Bloomberg unveiled its own LLM: BloombergGPT. GPT stands for generative pre-trained transformer, a term that has entered the cultural lexicon as large language models and chatbots remain in the headlines.
Bloomberg is looking to have BloombergGPT assist—and provide more depth to—the Terminal’s sentiment analysis, named-entity recognition (NER), news classification, charting and question-answering capabilities, among other functions. Or, more simply, Bloomberg hopes it will become the engine that will supercharge its Terminal.
Shawn Edwards, Bloomberg’s CTO, told WatersTechnology that, initially, the enhancements to the Terminal will be more behind the scenes. “This is all very early days and we’re just scratching the surface—this is really Day One,” he says. “But the dream—and we’ve been working on this for years—has been for a user to walk up to the Terminal and ask any question and get an answer or lots of information, to get you to the starting point of your journey.”
BloombergGPT is a 50 billion parameter large language model. That’s smaller than GPT-4, which is reported to have 1 trillion parameters, and Google’s Language Model for Dialogue Applications (LaMDA) at 137 billion. The smaller parameter number might work to Bloomberg’s advantage. Scaling and training models is not an easy task and it can be incredibly expensive. In January, a machine learning engineer at a large financial data and technology provider told WatersTechnology that it can be like boiling the ocean.
But this doesn’t always have to be the case, says Matei Zahara, co-founder and CTO at Databricks, a data architecture company. Last month, the company unveiled its LLM, Dolly, which has only 19 billion parameters. Dolly is an open model and its 2.0 version was released last week for research and commercial use.
“These are a lot less expensive to train than something like GPT-3, and I think they’ll be quite good, especially in the enterprise setting where you have a more focused domain,” Zahara says of Dolly. GPT-3’s large parameter number correlates to how much of the internet it’s been trained on, something that wouldn’t be as necessary in a specialized model being used by a bank or asset manager. Hence BloombergGPT’s smaller parameter number.
But that size difference isn’t the only thing distinguishing Bloomberg’s model.
To train BloombergGPT, the data giant constructed a dataset dubbed FinPile, which consists of financial documents written in English including news, filings, press releases, web-scraped financial documents, and social media drawn from the Bloomberg archives. These documents have been acquired through Bloomberg’s business process over the past two decades, according to the BloombergGPT research paper. FinPile is paired with public datasets widely used to train LLMs, including The Pile, C4 and Wikipedia. FinPile makes up 54.2% of training while public datasets are 48.73%. The result is a training corpus of more than 700 billion tokens.
In the accompanying research paper, Bloomberg says that it trained its model on domain-specific and general-purpose data for a model that has balanced performance in both domains. Besides The Pile, C4 and Wikipedia, it’s also looked to datasets like YouTubeSubtitles, USPTO Backgrounds, and even one called Enron Emails. (It’s exactly what you think it is.)
Bloomberg developing a large language model makes sense. The company has been around and collecting data since 1981, making it a prime candidate for training data that underpin a financial services-specific model.
The question now: Will other data providers follow suit?
S&P Global has already invested in artificial intelligence via its 2018 purchase of Kensho. At the time, S&P said it wanted to become a world leader in AI development with the buy focused on the talent and knowledge from Kensho’s engineers and the ability to infuse its technology stack with natural language processing, machine learning, and other subsets of AI. Since then, Kensho has rolled out several tools for linking, named-entity recognition and disambiguation, sentiment, and cataloging. These are similar areas to what Bloomberg is looking to tackle within the Terminal.
More data and information providers going down the large language model path isn’t a wild hypothesis. “Whether it’s Moody’s or Refinitiv or Bloomberg, naturally, their fundamental job is to provide users with insight, information, and data,” says a chief data and AI officer at an Asia-based bank. “But largely, it’s there for you to do whatever you want with it. Now, suddenly, there is this additional layer. Bloomberg can say, ‘I’m not just providing you the data, I’m providing you the ability to interface with that data, to formulate your questions and get responses to what your interest is.'”
The Asia bank executive says this functionality should appeal to analysts because it allows for a more horizontal and holistic research and analysis process, instead of getting a pile of 1,000 papers dumped on their desk with a Post-It note attached that says, “Go figure it out.” Instead, it’s the ability to ask questions like, “What’s the key theme among all of these documents?”
Bloomberg’s Edwards isn’t sure if this will be a wave among providers. “I actually don’t know if it’s a wave across all these providers. I do think that this is a fundamental, giant leap in AI capabilities,” he says. “It’s probably the biggest leap in computer science AI in decades. And companies that know how to build it and use it safely and give good results and accurate information, I think, are the ones that will succeed.”
Gideon Mann, Bloomberg’s head of research and machine learning, agrees. “There’s been a big question about the degree to which we are moving to a unipolar world where there are a few big players, and they’re the only ones who can develop and deploy technology like that of OpenAI and Google,” he says. However, he adds, Bloomberg's research paper demonstrates that if you have the right team and data, it is possible to build a domain-specific model that is fit for purpose.
Mann says it’s not clear where the applications will come from, but he thinks the movement is toward a world where there will be many different language models fit for different purposes. Whether or not the machines will enslave the human race is a question for another day—at present, firms need to be more concerned with how this “giant leap in AI capabilities” will affect their product offering.
Additional reporting by Anthony Malakian and Wei-Shen Wong
Only users who have a paid subscription or are part of a corporate subscription are able to print or copy content.
To access these options, along with all other subscription benefits, please contact info@waterstechnology.com or view our subscription options here: http://subscriptions.waterstechnology.com/subscribe
You are currently unable to print this content. Please contact info@waterstechnology.com to find out more.
You are currently unable to copy this content. Please contact info@waterstechnology.com to find out more.
Copyright Infopro Digital Limited. All rights reserved.
As outlined in our terms and conditions, https://www.infopro-digital.com/terms-and-conditions/subscriptions/ (point 2.4), printing is limited to a single copy.
If you would like to purchase additional rights please email info@waterstechnology.com
Copyright Infopro Digital Limited. All rights reserved.
You may share this content using our article tools. As outlined in our terms and conditions, https://www.infopro-digital.com/terms-and-conditions/subscriptions/ (clause 2.4), an Authorised User may only make one copy of the materials for their own personal use. You must also comply with the restrictions in clause 2.5.
If you would like to purchase additional rights please email info@waterstechnology.com
More on Emerging Technologies
This Week: Startup Skyfire launches payment network for AI agents; State Street; SteelEye and more
A summary of the latest financial technology news.
Waters Wavelength Podcast: Standard Chartered’s Brian O’Neill
Brian O’Neill from Standard Chartered joins the podcast to discuss cloud strategy, costs, and resiliency.
SS&C builds data mesh to unite acquired platforms
The vendor is using GenAI and APIs as part of the ongoing project.
Chevron’s absence leaves questions for elusive AI regulation in US
The US Supreme Court’s decision to overturn the Chevron deference presents unique considerations for potential AI rules.
Reading the bones: Citi, BNY, Morgan Stanley invest in AI, alt data, & private markets
Investment arms at large US banks are taken with emerging technologies such as generative AI, alternative and unstructured data, and private markets as they look to partner with, acquire, and invest in leading startups.
Startup helps buy-side firms retain ‘control’ over analytics
ExeQution Analytics provides a structured and flexible analytics framework based on the q programming language that can be integrated with kdb+ platforms.
The IMD Wrap: With Bloomberg’s headset app, you’ll never look at data the same way again
Max recently wrote about new developments being added to Bloomberg Pro for Vision. Today he gives a more personal perspective on the new technology.
LSEG unveils Workspace Teams, other products of Microsoft deal
The exchange revealed new developments in the ongoing Workspace/Teams collaboration as it works with Big Tech to improve trader workflows.