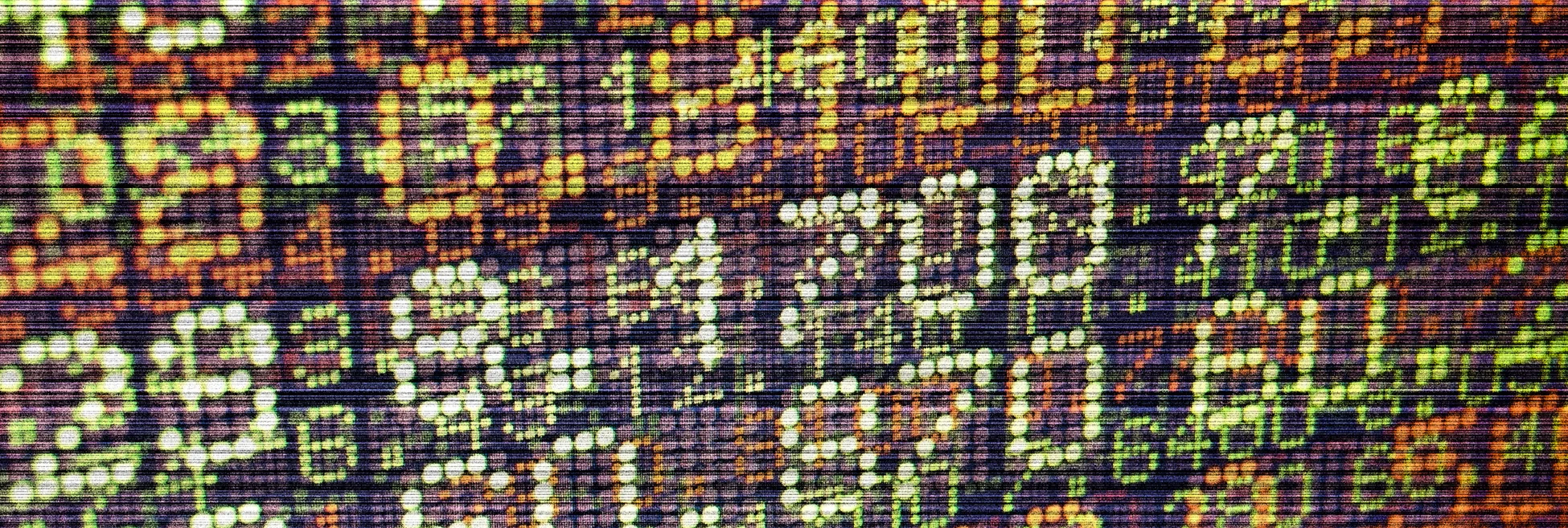
Fuzzy Data Stalls ESG Alpha Hunt
Quants searching for ESG signals have reached very different conclusions. Mostly they blame the data.
Need to know
- Efforts to find tradeable signals from environmental, social and governance data have made slow progress.
- In granular form and with the stock coverage quants need, ESG data goes back only about 10 years. That’s barely long enough to backtest quant strategies properly.
- The hunt for ESG alpha is further complicated by changes in the signals’ behaviour over time as ESG investing has gained popularity.
- Practitioners think it will take another five years before the data is good enough to reach solid conclusions about whether ESG signals work.
Quant investors who wield powerful but hard-to-understand models are used to dealing with sticky questions about cause and effect. It’s no different with ESG investing.
Experts are divided about whether screening investments by environmental, social and governance criteria influences returns. And if they see a link, they can’t always tell whether it’s coincidental or contributory.
Axa Investment Managers has found a “direct link” between board diversity and “a sustainable competitive advantage”, says Lise Renelleau, the firm’s director for sustainable investment solutions, and is using the signal and others relating to carbon emissions in its models.
Man Numeric says it has identified a set of signals unrelated to other quant factors that are predictive of positive returns, though the firm won’t disclose how powerful its ESG signals are.
Others are more doubtful about the connection.
Quants at Societe Generale, for example, found “no strong performance implication” when they looked at 10 years of ESG data, according to Giorgios Oikonomou, a quant with the firm.
BNP Paribas Asset Management, which runs multifactor funds that aim to lift ESG scores above their benchmarks, treats the ESG element of its investment process as an overlay, not a factor.
Academics are equally split. Some papers argue that companies with superior ESG practices attract and keep better staff and can access capital more cheaply – leading to outperformance. Others say so-called sin stocks are more profitable because they pay a premium to attract unwilling investors.
“No serious scholar at this point in time will say we have enough consistent evidence on whether ESG investing can relate to superior performance,” says Gianfranco Gianfrate, a professor of finance at Edhec Business School.
Quants do, however, agree on what’s to blame for the divergence in outlook. Data to rate and rank the ESG credentials of targets is patchy, covers too short a period and is plagued by bias. Quants also struggle to pin down what turns out to be a slippery target. The popularity of ESG investing is changing the power of ESG signals even as quants seek to measure them.
“Backtesting is challenging,” says Gerben de Zwart, managing director of quant strategies for Dutch pension fund APG. “ESG data providers have been around for many years but some have changed their definitions over time. Also, ESG might have been priced differently by the market a few years ago.”
Guesswork and gaps
ESG ratings from providers such as MSCI and Sustainalytics have existed for two decades and might be expected to provide consensus on measuring the effect of responsible corporate actions. But practitioners say the piles of data have turned out to be frustratingly hard to use.
A proliferation of data providers creates a sense that information is abundant, says Jennifer Bender, head of equity index research at State Street Global Advisors. “But the data is at least 50% estimated, meaning it’s not real data reported by the companies. There are a lot of gaps.”
In particular, the granular data required by quants and datasets covering a wide enough universe of stocks goes back only about 10 years. That’s a special problem in quant investing where convention is to backtest potential strategies over at least that period and to use a further two years of data for “out of sample” testing, ie with data unused in the construction and calibration of the strategy.
Quant models can only allow for about 5% of data fields to be empty before they break down, says Mike Hunstad, head of quantitative research at Northern Trust Asset Management.
Quants have found that raters’ different ways of refining the raw data they gather adds further to the confusion. Leading providers concur on only about half the companies they rate according to data from State Street Global Advisors. Some score companies by picking out the best and worst performers overall. Others pick out the best and worst by industry. “You can come up with an oil company that has a huge carbon footprint but good sustainability ratings,” says Gianfrate. Some raters score missed reporting fields as neutral, others penalise them.
Some quants think data vendors could be falling prey to a form of overfitting, by selecting data to gather and make available that appears to have a positive link to returns in the past – when the patterns in those data points may in fact have arisen by chance.
When one quant examined several commercially available datasets, he found the performance of backtested strategies to be “too good to be true”, he says, attributing the finding to this overfitting effect.
The volume of data points on offer – which include everything from water use efficiency to human rights concerns – makes it perilously easy for users to be misled by fluke patterns.
Elsewhere, practitioners have found that scores imply different things for different types of companies. Some quant funds have turned to machine learning to try and pin down which individual ESG characteristics are material for certain industries and in what macroeconomic conditions.
You can come up with an oil company that has a huge carbon footprint but good sustainability ratings
Gianfranco Gianfrate, Edhec Business School
Then there are what quants call structural challenges – in simple terms, different forms of bias.
In an ideal world, analysts would be able to compare two companies identical in every respect except for ESG, to verify the validity of criteria. In the real world, practitioners have discovered that financially sound companies tend also to have good ESG ratings and vice versa, robbing the analysts of many candidates for statistical cross-checking.
It’s also hard to gauge the effect of ESG signals in isolation because they tend to overlap with already-known quant factors such as quality or low volatility, which can explain some of the positive performance in ESG-tilted portfolios, says Raul Leote de Carvalho, deputy head of quantitative research at BNP Paribas Asset Management.
At the same time, the growing popularity of ESG investing has made the data harder to interpret and muddied the picture of likely performance going forward. To win and keep business from millennial investors, investment firms are having to “ESG-proof their portfolios”, in the words of Oikonomou. The resulting flows push up the price for good ESG stocks. Meanwhile, virtuous but neglected companies may become more attractive from a valuation point of view.
In simple terms, weak ESG stocks now may recover when bargain-hunting buyers start to pick them up.
Such widespread enthusiasm for ESG investing is a recent trend, which means quants also have laboured to find evidence in the historical data of signals that could work well today. “They’re looking back 10 years and ESG was irrelevant then,” Oikonomou says.
Not perfect, but good enough
Where does this leave investors? Mostly it leaves them waiting.
Some see value in persevering with ESG even though such strategies can’t reliably offer the same level of returns as other factors.
“We do as well as we can with the data we have,” says Tomas Morsing, head of quantitative strategies at AP2. The fund’s ESG-tilted strategies deliver about half the premium of more conventional quant strategies, he says, but that is good enough. AP2 plans to apply ESG signals in its fixed income portfolios later this year.
A few, such as APG, have sought to address the shortcomings of ESG data by collating their own. The firm uses machine learning to classify companies by compliance with UN sustainable development goals. And from January 1, it has released these sustainable classifications to other asset owners through a third-party data distributor, to encourage wider adoption. The second largest pension fund in the Netherlands, PGGM, has committed to using the classifications in its reporting.
For some investors, ESG scores contain too much in-built subjectivity to present a unified picture of companies’ strengths and weaknesses. But for others, this isn’t a deal-breaker. Leote de Carvalho likens ESG ratings to factor strategies where there are multiple ways to define a given factor and investors can choose based on their preferences.
BNPP AM found its strategies performed consistently in testing regardless of which ESG scores the team started with, he says, because the raw information underlying the scores is the same.
For the most part, though, quants see a resolution of the ESG data muddle as possible, but believe it will take time. How long? Kasper Lorenzen, chief investment officer at Danish pension fund PFA, thinks it could be five to 10 years before firms will be able to say for certain what ESG has to offer.
“Then we’ll get a sign of whether there’s true return predictability in the scores.”
Further reading
Only users who have a paid subscription or are part of a corporate subscription are able to print or copy content.
To access these options, along with all other subscription benefits, please contact info@waterstechnology.com or view our subscription options here: http://subscriptions.waterstechnology.com/subscribe
You are currently unable to print this content. Please contact info@waterstechnology.com to find out more.
You are currently unable to copy this content. Please contact info@waterstechnology.com to find out more.
Copyright Infopro Digital Limited. All rights reserved.
As outlined in our terms and conditions, https://www.infopro-digital.com/terms-and-conditions/subscriptions/ (point 2.4), printing is limited to a single copy.
If you would like to purchase additional rights please email info@waterstechnology.com
Copyright Infopro Digital Limited. All rights reserved.
You may share this content using our article tools. As outlined in our terms and conditions, https://www.infopro-digital.com/terms-and-conditions/subscriptions/ (clause 2.4), an Authorised User may only make one copy of the materials for their own personal use. You must also comply with the restrictions in clause 2.5.
If you would like to purchase additional rights please email info@waterstechnology.com
More on Data Management
New working group to create open framework for managing rising market data costs
Substantive Research is putting together a working group of market data-consuming firms with the aim of crafting quantitative metrics for market data cost avoidance.
Off-channel messaging (and regulators) still a massive headache for banks
Waters Wrap: Anthony wonders why US regulators are waging a war using fines, while European regulators have chosen a less draconian path.
Back to basics: Data management woes continue for the buy side
Data management platform Fencore helps investment managers resolve symptoms of not having a central data layer.
‘Feature, not a bug’: Bloomberg makes the case for Figi
Bloomberg created the Figi identifier, but ceded all its rights to the Object Management Group 10 years ago. Here, Bloomberg’s Richard Robinson and Steve Meizanis write to dispel what they believe to be misconceptions about Figi and the FDTA.
SS&C builds data mesh to unite acquired platforms
The vendor is using GenAI and APIs as part of the ongoing project.
Aussie asset managers struggle to meet ‘bank-like’ collateral, margin obligations
New margin and collateral requirements imposed by UMR and its regulator, Apra, are forcing buy-side firms to find tools to help.
Where have all the exchange platform providers gone?
The IMD Wrap: Running an exchange is a profitable business. The margins on market data sales alone can be staggering. And since every exchange needs a reliable and efficient exchange technology stack, Max asks why more vendors aren’t diving into this space.
Reading the bones: Citi, BNY, Morgan Stanley invest in AI, alt data, & private markets
Investment arms at large US banks are taken with emerging technologies such as generative AI, alternative and unstructured data, and private markets as they look to partner with, acquire, and invest in leading startups.