Ghost in the Shell: AI Expands Beyond Algos
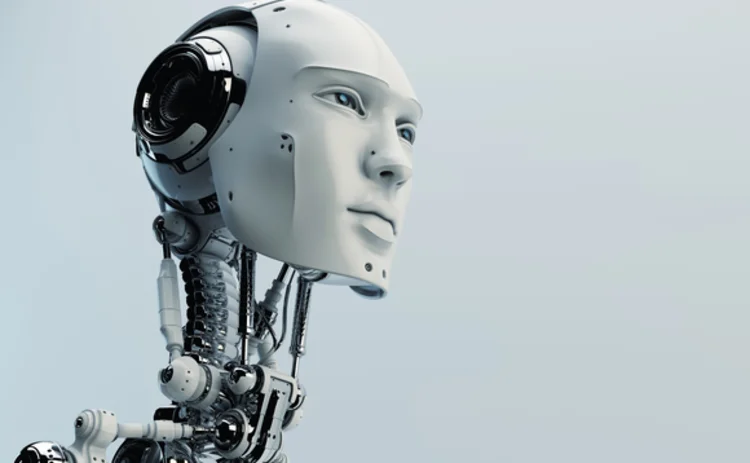
“You can look at it through the lens of science-fiction movies, where there’s a machine, and over time it starts thinking for itself—that’s exactly what this is,” says Sameet Gupte, vice president and managing director for Europe at Virtusa, outlining the development and growth of artificial intelligence (AI) within the financial services industry over the past few years. It’s not a new thing, he says, relaying a brief story about how he came across AI development in a major Asian bank in 1997. But pressures on human traders and infrastructure, whether from microsecond trading or a barrage of regulation demanding vast calculations on trades, are pushing banks and technologically adroit hedge funds into investigating the benefits of AI and its subfield, machine learning.
Put simply, machine learning does exactly what it says on the tin. Most programs are like wind-up soldiers, put in place and set on a path that they blindly follow according to their subroutines. It doesn’t matter if they walk into a wall, because the only direction they know is the one that they’ve been released on. Machine learning, on the other hand, allows the toy soldier to walk into a wall, realize that it’s not going anywhere, and then correct itself to turn 90 degrees and walk along the wall instead. It learns from data gleaned by past experience, and adjusts itself accordingly.
It’s hardly the stuff of Skynet and 2001: A Space Odyssey, but the applications of machine learning in the front office are clear. Algorithms that do not blindly follow their orders, but adjust venue, strategy and even stock selection based on market conditions and knowledge of how such orders interact on a typical basis with the market, are indeed extremely valuable pieces of technology.
Cerebral Simulation
It’s not a case of slapping some advanced event logic on top of an algorithm, giving it a target, and launching it like a smart munition at the stock exchange, however. Adoption of machine learning is taking tentative steps for most firms, centering on a few key areas of interest.
“We are focused on the quantitative side rather than the data warehousing side, but we’re beginning to see a degree of interest and specialization,” says Steve Wilcockson, industry manager for financial services at MathWorks, creators of the Matlab programming language. “The buy side is where I’ve seen it most discussed, albeit not necessarily applied, and the sort of tasks where it seems to be doing well include bond scoring, stock-selection processes, hedge fund tracking, and stock classification.”
“We truly believe that this is the way of the future—we believe that decisions are not going to be as manual as they currently are.” —Alfred Eskandar, Portware
The sort of insight machine learning gives in these areas is focused around nuance—picking out details of a bond for a fixed-income fund that may inspire a trading decision, for instance, and which competitors without the ability to crunch data in an intelligent fashion might not be able to replicate. Indeed, much of the discussion around machine learning’s potential is reminiscent of arguments surrounding the use of big data analytics and deriving value from noisy information.
While complex event-processing (CEP) engines and the like may have advanced analytic capabilities, though, they’re not the same as having an AI component. For this, increased interest is centering on neural networks.
“Even if you have the fastest chips, the lowest latency connections to the exchange and all of that, it’s difficult to manage all the parameters involved and keep them in scope,” says Virtusa’s Gupte. “So what we’re seeing is a lot of the technology arms of these banks looking at neural networks and artificial intelligence, which they’re leveraging by training them up.”
Put simply, a neural network is a collection of data structures and infrastructures that simulates the operation of the human brain through large numbers of processors working in parallel. It is usually “trained” using large volumes of historical data, which then enables it to react to events based on its experience from the information it has been fed, and continues to take in new data as it learns.
“The way a neural network and an AI generally works is that once you parse a particular problem, it trains itself and starts to become increasingly intelligent by adapting to multiple scenarios that could occur during that particular trade,” Gupte says. “A human brain can do that, but not at the speed and depth that AI can.”
Virtual Traders
Naturally, the establishment of machine learning, and approximating the human brain requires first-grade investment … and a lot of people with a lot of letters after their names. New York-based trading technology vendor Portware, for instance, has eight PhDs working on the machine-learning components of its offerings, and markets itself as one of the only execution management system (EMS) providers to include the use of commercialized AI. Rather than following the pattern of AI for specific tasks, however, Portware’s algorithms not only suggest trading strategies, but also communicate with the traders themselves.
“We truly believe that this is the way of the future—we believe that decisions are not going to be as manual as they currently are,” says Alfred Eskandar, CEO at Portware. “We believe that the end-user needs decision support in real time, and what we’ve essentially developed is a graphical user interface that lets the algorithms talk to the trader. As the AI is doing its job, it’s updating the trader in plain English—it’s telling them that they’re going to move from an aggressive peg to a passive dark strategy because of this; they’re going to shift from this venue to that venue or from this algorithm to that algorithm, and here’s why. The reason we spent so much time and money to develop that is that if you don’t give a trader confidence that the machine is working, then they will not trust a black box.”
The business case for using AI in decision support is clear, particularly considering the vast volumes of information that a trader must consider for a single order. These will typically include news about a particular company, for which a trader will check Bloomberg, Reuters and other news sources, and even pick up the phone and talk to a sales trader or someone with a similar remit who might have recently transacted that stock. Then there will be the algorithmic selection, which will involve looking at volatility and volume, block activity, and where liquidity is for that particular stock. After that, it’s monitoring the performance of the algorithm in terms of participation rates, and any post-trade analysis, all of which adds time and requires quick decisions, although much of this process can be automated. Indeed, so broad are the benefits of having a machine capable of crunching over 40 different factors in real time and outputting them within moments, that some of Portware’s clients are using them as virtual traders.
“The irony is that when it’s high vacation season, we get a lot of extra orders, because it can be a traders’ assistant,” says Eskandar. “So for the smaller firms—which for us are $3 billion hedge funds—they only have two or three traders. This handles orders while they work other areas.”
On the larger end of the scale, for asset managers with at least $100 billion in assets under management (AUM), AI handles noise orders—trades that tie up the time of expensive human traders, and aren’t worth that much to the firm. Portware’s technology is designed to typically execute such orders, and, using the AI, add value in some cases of up to five basis points for what have been in the past largely worthless transactions.
Risk and Reward
On the demographic front, in the experience of those interviewed for this article, it tends to be the small and mid-sized buy-side firms that are currently able to make the most of machine learning. Examples include shops such as New York-based Rebellion Research, with AUM in the single-digit millions, which trades purely from AI and machine-learning algorithms. Other examples include Cerebellum Capital, based in San Francisco with a similar AUM figure, and RGM Advisors in Austin, Texas.
These smaller firms can, after all, afford to take risks with new technologies, and the relatively modest amounts of capital at stake aren’t necessarily going to break the financial system if it all goes sideways. That’s not the case, however, with larger, tier-one investment banks, most of which are designated as systemically important financial institutions, and all of which trade on reputation, reliability and trust. Still, even though AI may not be widely used at such institutions—although it is certainly being used—there are avenues that some are exploring where a targeted application could reap rewards.
“Right now, we’re looking at machine learning proofs-of-concept for unstructured data,” says Michel Dinh, director and regional head of Asia-Pacific prime services and listed derivatives IT at Credit Suisse. “We use it for a number of purposes, one of which is unauthorized trading. Regardless of how many regulations come out, we still frequently see trading going wrong, whether that’s due to prices not being checked, fat fingers, flash crashes and so on, so we use it for that, and that is where we think that machine learning can help. It doesn’t rely on purely structured data in a database—you can tap into e-mail, you can discern patterns, and you can figure out the frequency of it. Other areas we’re playing with in the prime services business are short selling, borrowing and stock lending, as well as research.”
Indeed, entities such as stock exchanges are actively researching and developing AI-augmented—if not AI-based—systems that can be used for a variety of roles. One head of a technology arm at a global stock exchange group described how his staff is looking at ways in which AI can be used to automate and assist with a number of functions. As these business units provide technology to infrastructural organizations within the industry—clearinghouses, central counterparties, trade repositories and exchanges—AI could help alleviate the burden associated with manual testing that introducing new technology involves. Another clear area of value is in market surveillance, where big-data analytics can be combined with machine learning to more proactively detect market abuse and fraud.
Robot Traders
Despite the benefits, though, machine learning is very much a way of augmenting the trading process rather than superseding it. Even for vendors such as Portware, humans are still a key element in the chain, and while neural networks offer vast potential in crunching complicated equations, there needs to be a concomitantly large and sophisticated back-end to support it. More than anything else, though, is the risk of fully trusting a black box, regardless of how well it trains itself and how intelligent it may appear to be.
“We haven’t really done anything in machine learning to put on a trading desk yet, because there’s operational risk involved—we don’t want machines making decisions about short selling,” says Credit Suisse’s Dinh. Not everyone agrees, however. Hong Kong-based venture capital firm Deep Knowledge Ventures recently appointed an algorithm to its board, complete with full voting rights, although there is still a degree of skepticism about the technology, even if the expanded use of AI in the financial services industry is, for all intents and purposes, fait accompli.
Salient Points
- Machine learning is actively being explored by financial institutions due to a number of factors including the vast amounts of information that needs to be crunched for most trades, regulations around risk calculation and reporting, and a need to be first to market with new technologies.
- On the buy side, processes suited for machine learning include stock selection, bond scoring, hedge fund tracking, and decision support. The sell side is actively interested in neural networks and index tracking, although institutions are generally more cautious than those on the buy side.
- While there are instances of artificial intelligence (AI) being used as trading assistants, institutions have been reluctant to place complete transacting discretion in the hands of machines. A lot of work is being mooted around marrying big-data disciplines with machine learning.
- Other areas are being explored, however, such as incorporating AI within testing processes at market infrastructure organizations, and integrating machine learning with surveillance systems.
Only users who have a paid subscription or are part of a corporate subscription are able to print or copy content.
To access these options, along with all other subscription benefits, please contact info@waterstechnology.com or view our subscription options here: http://subscriptions.waterstechnology.com/subscribe
You are currently unable to print this content. Please contact info@waterstechnology.com to find out more.
You are currently unable to copy this content. Please contact info@waterstechnology.com to find out more.
Copyright Infopro Digital Limited. All rights reserved.
As outlined in our terms and conditions, https://www.infopro-digital.com/terms-and-conditions/subscriptions/ (point 2.4), printing is limited to a single copy.
If you would like to purchase additional rights please email info@waterstechnology.com
Copyright Infopro Digital Limited. All rights reserved.
You may share this content using our article tools. As outlined in our terms and conditions, https://www.infopro-digital.com/terms-and-conditions/subscriptions/ (clause 2.4), an Authorised User may only make one copy of the materials for their own personal use. You must also comply with the restrictions in clause 2.5.
If you would like to purchase additional rights please email info@waterstechnology.com
More on Trading Tech
Recent volatility highlights tech’s vital role in fixed income pricing
MarketAxess’ Julien Alexandre discusses how cutting-edge technology is transforming pricing and execution in the fixed income market amid periodic bouts of volatility
Banks fret over vendor contracts as Dora deadline looms
Thousands of vendor contracts will need repapering to comply with EU’s new digital resilience rules
Where have all the exchange platform providers gone?
The IMD Wrap: Running an exchange is a profitable business. The margins on market data sales alone can be staggering. And since every exchange needs a reliable and efficient exchange technology stack, Max asks why more vendors aren’t diving into this space.
This Week: Trading Technologies completes ANS deal; State Street; Equinix; and more
A summary of the latest financial technology news.
Interactive Brokers looks beyond US borders for growth opportunities
As retail trading has grown in volume and importance, Interactive Brokers and others are expanding international offerings and marketing abroad.
JP Morgan’s goal of STP in loans materializes on Versana’s platform
The accomplishment highlights the budding digitization of private credit, though it’s still a long road ahead.
As data volumes explode, expect more outages
Waters Wrap: At least for those unprepared—though preparation is no easy task—says Anthony.
This Week: ICE Bonds and MarketAxess plan to connect liquidity networks, TS Imagine, Bloomberg, and more
A summary of the latest financial technology news.