Goldman taps alt data for economic forecasts during pandemic
Economists at the bank leveraged a combination of public and third-party data to make conclusions about the future during uncertain times.
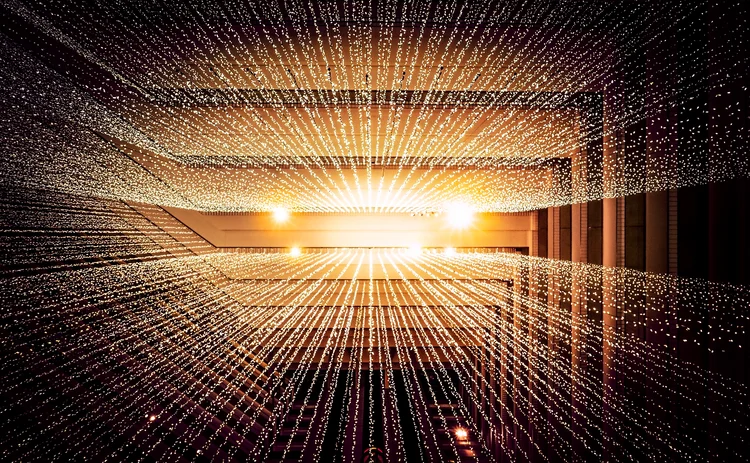
Like ski resorts or beach towns, the economies that surround large college campuses are highly reliant on their seasonal clientele. When Covid-19 struck in 2020, and colleges across the US ordered all or at least some of their students to stay inside—or even to return home—the bars, coffee shops, and retailers that were used to catering to hordes of students suffered.
For economists at Goldman Sachs, whose job it is to make economic predictions, the plight of these college-town businesses presented one way to understand how the economy was being hit by Covid-19. “College towns were particularly hard hit by the pandemic because students just were not going to them. These businesses remained, but a large share of the population wasn’t there anymore. What happened to them, and how are they doing today?” said Ronnie Walker, US economist at Goldman.
Walker was speaking on a webinar held by data provider SafeGraph, during which he presented examples of tools developed in the past year by Goldman that leverage alternative data to get a clearer picture of markets, and drive better decision-making at the bank.
This tool is nice because when you see a decline in consumer sentiment, you might not know what the reason is, but when you see a decline in our Twitter index, you can actually go look at the [individual] tweets and see what people are being negative about.
Ronnie Walker, Goldman Sachs
The tool that evaluates the impact of pandemic restrictions to businesses in college towns uses data from SafeGraph, which specializes in point-of-interest data—highly specific geospatial data on, for example, the square footage of hardware stores in Denver, or the geographic distribution of all coffee shops in San Francisco—to create a geofence around large colleges. The tool then analyzed foot traffic to businesses within that designated college town as a proxy for economic activity. As one might expect, the analysis proved that businesses near colleges that sent all their students home felt the biggest decline, while those that allowed some students to stay on campus did less poorly, Walker said.
This kind of specific case study feeds into the forecasts Goldman makes on 30 indicators of economic activity, including gross domestic product (GDP). In the novel circumstances of the Covid-19 outbreak, the bank’s economists turned to sources of alternative data to understand the world better. They were by no means alone in their increased interest in these datasets: the pandemic drove the financial services industry in general to consume more alternative data, as firms wanted to make macroeconomic forecasts or to assess the impact of the pandemic at the sector level.
A concoction
Goldman’s tools for pandemic forecasting were built using one of three strategies, according to Walker. Some solely pull in data from third-party vendors, such as SafeGraph, as well as free sources of data like Google mobility data, and public data such as census data or economic indicators provided by universities; other tools use a blend of alternative datasets mixed with traditional market and reference data sources; and still others are built from scratch using proprietary data.
In another example of a tool that Goldman built, economists analyzed bankruptcy data to make forecasts on the longer-term health of the economy. Economists believe that higher rates of bankruptcy have a major scarring effect on the long-term health of an economy (“scarring” is a term economists use to describe the effects of shocks to the economy), so higher rates of bankruptcies during the pandemic would indicate a poor outlook for the US economy.
Bloomberg bankruptcy data (accessible in the Terminal with the code BCY) indicated that there was a surge of bankruptcies among large companies in the early days of the pandemic. However, data from the American Bankruptcy Institute, which tracks a much wider range of firms than the Bloomberg data, showed that bankruptcies remained relatively stable in 2020. What this showed, Walker said, was that while economic disruption in the early days of the pandemic finished off companies that were already struggling, massive fiscal stimulus prevented mass bankruptcies.
“So, knowing that there haven’t been extreme scarring effects on the economy was helpful for us in maintaining an optimistic view about how quickly the US was able to recover, and will recover going forward,” he said.
Yet another tool developed by Goldman is a collection of third-party data on credit card spending and foot traffic, which its economists use to measure consumer spending.
“Consumer spending makes up two-thirds of GDP, so it’s pretty important to economic forecasters to get consumer spending right. You get that right, you’re going to get GDP at least partly right,” Walker said.
Their value-add in this case was that where the datasets provided a lot of granularity, Goldman’s team would try to match the data to the official national statistics. For example, if a credit card holder made a payment to a particular company, and the dataset was granular enough to include the company’s NAICS code, which identifies the sector to which a US business belongs, Goldman could match that transaction to the categories of consumer spending in the national accounts data that countries keep on their production, consumption, and savings, among other economic activity.
On average, in 2020, we outperformed consensus by slightly more than we normally do, and the indicators where we performed best were where we leveraged alternative datasets and we had invested in new resources.
Ronnie Walker, Goldman Sachs
“And then we would use those weights to re-stratify our dataset to get something more accurate,” Walker said. “This tool has been very helpful for us to keep track of consumer spending on a high-frequency basis, and to be able to react more quickly to news on the economy. So, if there’s been a new wave of shutdowns, [we ask] should we be worried about this and change our overall forecast? Well, if we wait a week, we can see what the consumer activity tracker does, and lo and behold, it didn’t have as much of a reaction, or it did start to react, and we were able to adjust our forecasts.”
Getting sentimental
The final tool that Walker described on the webinar was a Twitter sentiment index. For this tool, Goldman bought Twitter data filtered by a third-party provider for tweets specifically indicating an opinion on the economy, performed sentiment analysis on those tweets, and then built an aggregated index.
“[The index] ends up correlating fairly well with the University of Michigan index of consumer sentiment, and consumer sentiment correlates extremely well with consumer spending and happens to be fairly timely. So this was one of our first indications that there was going to be a bit of an issue with the economy, all the way back in February or early March, when we saw consumer sentiment tank, and we were able to get a read on actual consumer spending and activity,” he said.
“This tool is nice because when you see a decline in consumer sentiment, you might not know what the reason is, but when you see a decline in our Twitter index, you can actually go look at the [individual] tweets and see what people are being negative about.”
Walker said that when it comes to alternative data, Goldman is searching for information that is timely and that can make forecasts more accurate. To evaluate whether the data will achieve that, the researcher must come up with a model and then compare that to a baseline such as Bloomberg’s macroeconomic consensus data, which is derived from the predictions of a panel of professional forecasters. This form of backtesting can increase confidence in data that doesn’t go back a long time, Walker said.
He added that these tools helped Goldman’s economists make more accurate predictions.
“It took us a couple months to realize how big the pandemic was going to get, but we did end [up] cutting the Q1 and Q2 GDP forecast about a month before [the Bloomberg] consensus, and a few of the reasons we felt confident about cutting the forecasts were that we had a bunch of these timely, granular datasets to give us more in making those decisions. On average, in 2020, we outperformed consensus by slightly more than we normally do, and the indicators where we performed best were those where we leveraged alternative datasets and we had invested in new resources.”
Further reading
Only users who have a paid subscription or are part of a corporate subscription are able to print or copy content.
To access these options, along with all other subscription benefits, please contact info@waterstechnology.com or view our subscription options here: http://subscriptions.waterstechnology.com/subscribe
You are currently unable to print this content. Please contact info@waterstechnology.com to find out more.
You are currently unable to copy this content. Please contact info@waterstechnology.com to find out more.
Copyright Infopro Digital Limited. All rights reserved.
As outlined in our terms and conditions, https://www.infopro-digital.com/terms-and-conditions/subscriptions/ (point 2.4), printing is limited to a single copy.
If you would like to purchase additional rights please email info@waterstechnology.com
Copyright Infopro Digital Limited. All rights reserved.
You may share this content using our article tools. As outlined in our terms and conditions, https://www.infopro-digital.com/terms-and-conditions/subscriptions/ (clause 2.4), an Authorised User may only make one copy of the materials for their own personal use. You must also comply with the restrictions in clause 2.5.
If you would like to purchase additional rights please email info@waterstechnology.com
More on Data Management
New working group to create open framework for managing rising market data costs
Substantive Research is putting together a working group of market data-consuming firms with the aim of crafting quantitative metrics for market data cost avoidance.
Off-channel messaging (and regulators) still a massive headache for banks
Waters Wrap: Anthony wonders why US regulators are waging a war using fines, while European regulators have chosen a less draconian path.
Back to basics: Data management woes continue for the buy side
Data management platform Fencore helps investment managers resolve symptoms of not having a central data layer.
‘Feature, not a bug’: Bloomberg makes the case for Figi
Bloomberg created the Figi identifier, but ceded all its rights to the Object Management Group 10 years ago. Here, Bloomberg’s Richard Robinson and Steve Meizanis write to dispel what they believe to be misconceptions about Figi and the FDTA.
SS&C builds data mesh to unite acquired platforms
The vendor is using GenAI and APIs as part of the ongoing project.
Aussie asset managers struggle to meet ‘bank-like’ collateral, margin obligations
New margin and collateral requirements imposed by UMR and its regulator, Apra, are forcing buy-side firms to find tools to help.
Where have all the exchange platform providers gone?
The IMD Wrap: Running an exchange is a profitable business. The margins on market data sales alone can be staggering. And since every exchange needs a reliable and efficient exchange technology stack, Max asks why more vendors aren’t diving into this space.
Reading the bones: Citi, BNY, Morgan Stanley invest in AI, alt data, & private markets
Investment arms at large US banks are taken with emerging technologies such as generative AI, alternative and unstructured data, and private markets as they look to partner with, acquire, and invest in leading startups.