How GenAI could improve T+1 settlement
As well as reducing settlement failures, researchers believe generative AI can provide investment managers with improved research, prioritization, and allocation resources.
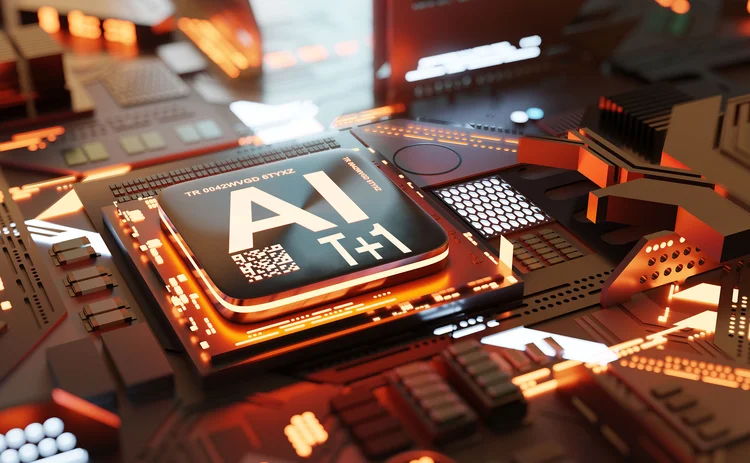
On May 28, 2024, the US Securities and Exchange Commission will implement a pivotal regulatory change, transitioning the trade settlement cycle from T+2 to T+1 for all US trades processed through the Depository Trust & Clearing Corporation. This directive necessitates that all trades be affirmed by 9:00 pm ET on T+0. Currently, the target is to attain 90% affirmation compliance by this deadline once the regulation takes effect.
Recent findings from a DTCC report reveal that as of December 2023, 69% of all trades have met this requirement. While this demonstrates promising strides in readiness toward meeting the imminent goal, there remains ample room for enhancement, with automation serving as the cornerstone.
Closing the gap between T+2 and T+1 largely pivots on leveraging existing technologies. For instance, investment managers equipped with TradeSuite ID numbers can capitalize on the DTCC’s automation mechanisms, such as the Central Trade Matching platform’s Match to Instruct workflow auto-affirmation capabilities—or similar automation solutions—to streamline operations and expedite trade affirmations. Additionally, exploring other automation avenues and fostering collaboration across industry stakeholders could further expedite the transition and ensure seamless compliance with the new settlement cycle.
Cross-border trades
Cross-border trading intricacies arise due to the disparate time zones of equity and foreign exchange markets. Currently, FX solutions are primarily driven by investors collaborating with FX providers and custodians to devise tailored strategies. However, a more streamlined cross-border solution would entail amalgamating equity trade and FX processing into a cohesive framework, a concept still in its nascent stage.
Cross-border trading introduces complexities from equity and FX markets operating in different markets in different time zones. For the present, the probable best approach for FX is left to the investors to work with their FX providers and custodians to get the solution that works best for them.
A better cross-border solution would be to combine equity trade and FX processing in a single solution yet to be defined. A few decades ago, we explored the idea of global straight-through processing for cross-border trades that was more of an event-driven approach with less emphasis on meeting specific time constraints. In recent years, a move to blockchain has been considered, which would change the process from T+1 to something more like T+10-minutes using a cryptocurrency exchange.
However, these advancements introduce additional complexities and challenges beyond the scope of this discussion, underscoring the need for thorough evaluation before implementation. There may also be other issues that are not within the scope of this article.
Meet generative AI
GenAI technology promises to address the further goal of getting much more than 90% compliance with T+1—possibly getting us close to 100%.
This involves using large language models (LLMs) built from carefully curated data, which are then fine-tuned to address a particular task, such as compliance checks and predicting when affirmations will fail in collaboration with traditional machine learning-based failure prediction models. If we can make that prediction sufficiently in advance of 9:00 pm on T+1, we have an opportunity to address the failure and prevent it from occurring.
The custodians would most likely implement this processing. We might also consider more flexibility in the settlement schedule. If an affirmation isn’t going to be there at 9:00 pm but can make it by, say, 9:30 pm, maybe that still works for T+1 settlement. This suggests some level of event-driven automation, perhaps with some boundaries, which may be fuzzy thresholds.
GenAI can also assist with additional steps in the settlement cycle. Using a unified, user-friendly interface, investment managers can give clients real-time visibility into their investments’ status, research the best course of action, prioritize critical risk items, and provide an allocation progress report, among other things.
Another area where genAI might be helpful is automating communication among several parties. Generative modeling, such as Generative Adversarial Networks (GANs), can also help handle exceptions during settlement. Improving resolution workflows, it finds anomalies independently and sorts them by impact, urgency, or severity. This method shortens the resolution time and reduces the likelihood of settlement failures caused by unresolved exceptions by making intelligent suggestions.
As a result of their proactive approach to compliance issues, LLMs significantly lessen the likelihood of settlement failures due to regulatory infractions and guarantee thorough and accurate reporting
The Deep Convolutional GAN (DCGAN) has been widely used and highly praised for its effectiveness as one of the most critical GAN implementations. Large language models are a lifesaver when generating regulatory reports and staying compliant with settlements. They analyze trade data against applicable regulatory frameworks, spot possible non-compliance concerns, and produce detailed reports to fulfill regulatory mandates.
As a result of their proactive approach to compliance issues, LLMs significantly lessen the likelihood of settlement failures due to regulatory infractions and guarantee thorough and accurate reporting. GenAI uses recurrent neural networks (RNNs) to check that completed transactions are accurate and complete after they’ve settled by doing audits and reconciliations.
Using RNNs, reconciling data from various clearing parties is compared with settled trade data. Discrepancies are then highlighted, making the reconciliation process faster and easier. This stage is critical for identifying missed or unsuccessful settlements to facilitate prompt resolutions.
Here is what the AI-infused settlement process looks like:
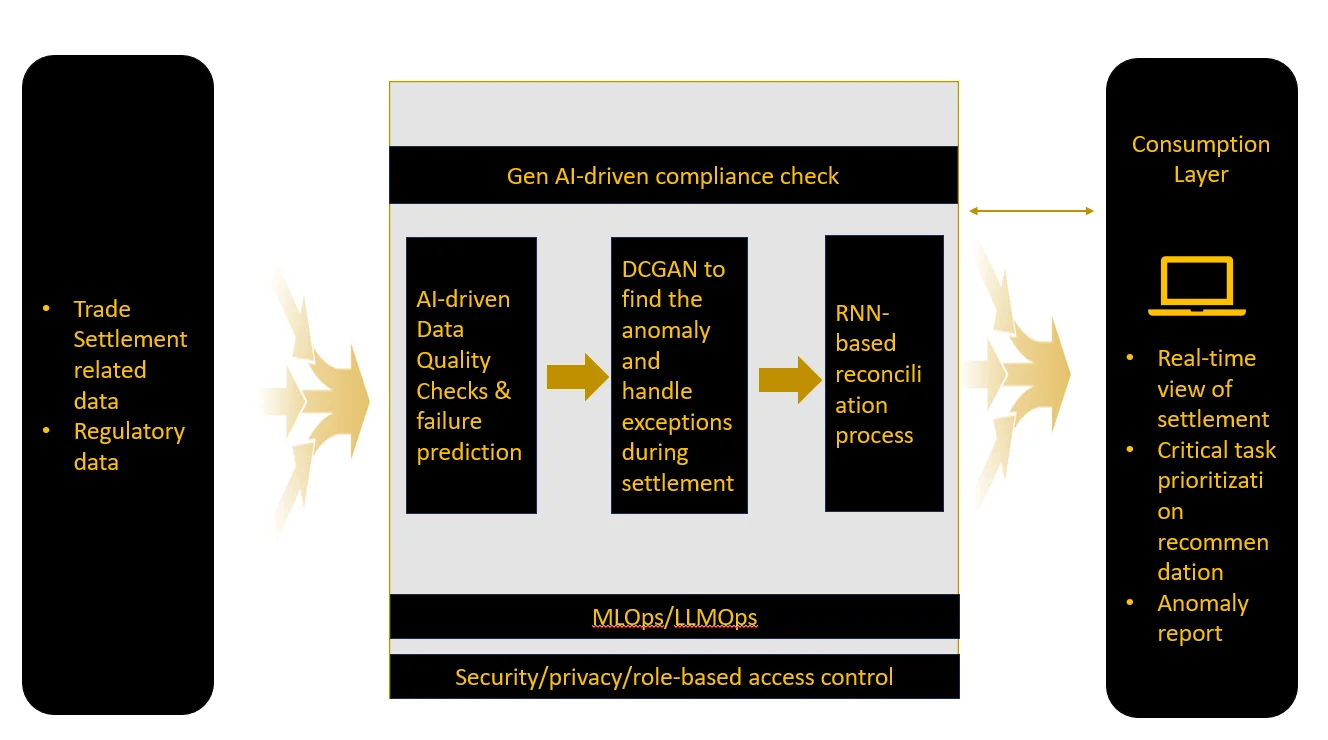
We should also explore potential AI solutions to the FX issues, perhaps bridging the gap between equity and FX processes.
Conclusion
T+1 is now imminent, and fortunately, there exists a considerable arsenal of technology ready to facilitate this transition. Integrating AI into existing systems holds promise in mitigating the complexities associated with this change. Adaptive trading systems, driven by the exploratory capacities of GenAI, engage in continual learning from real-time data, adeptly adapting to evolving market dynamics.
These systems actively integrate feedback, monitor algorithm performance, and make necessary adjustments to enhance the efficacy and accuracy of deployed ML models. Through this iterative learning process, they can preemptively identify and prevent increasingly intricate settlement failures, progressively enhancing their functionality over time.
Anjanita Das, Director AI Practice, Cognizant Technology Solutions
Kenneth Schoff, Principle Partner-Sustainability, IBM
Raja Basu, Business Architect (Financial Markets), IBM
Only users who have a paid subscription or are part of a corporate subscription are able to print or copy content.
To access these options, along with all other subscription benefits, please contact info@waterstechnology.com or view our subscription options here: http://subscriptions.waterstechnology.com/subscribe
You are currently unable to print this content. Please contact info@waterstechnology.com to find out more.
You are currently unable to copy this content. Please contact info@waterstechnology.com to find out more.
Copyright Infopro Digital Limited. All rights reserved.
As outlined in our terms and conditions, https://www.infopro-digital.com/terms-and-conditions/subscriptions/ (point 2.4), printing is limited to a single copy.
If you would like to purchase additional rights please email info@waterstechnology.com
Copyright Infopro Digital Limited. All rights reserved.
You may share this content using our article tools. As outlined in our terms and conditions, https://www.infopro-digital.com/terms-and-conditions/subscriptions/ (clause 2.4), an Authorised User may only make one copy of the materials for their own personal use. You must also comply with the restrictions in clause 2.5.
If you would like to purchase additional rights please email info@waterstechnology.com
More on Regulation
Off-channel messaging (and regulators) still a massive headache for banks
Waters Wrap: Anthony wonders why US regulators are waging a war using fines, while European regulators have chosen a less draconian path.
Banks fret over vendor contracts as Dora deadline looms
Thousands of vendor contracts will need repapering to comply with EU’s new digital resilience rules
Chevron’s absence leaves questions for elusive AI regulation in US
The US Supreme Court’s decision to overturn the Chevron deference presents unique considerations for potential AI rules.
Aussie asset managers struggle to meet ‘bank-like’ collateral, margin obligations
New margin and collateral requirements imposed by UMR and its regulator, Apra, are forcing buy-side firms to find tools to help.
The costly sanctions risks hiding in your supply chain
In an age of geopolitical instability and rising fines, financial firms need to dig deep into the securities they invest in and the issuing company’s network of suppliers and associates.
Industry associations say ECB cloud guidelines clash with EU’s Dora
Responses from industry participants on the European Central Bank’s guidelines are expected in the coming weeks.
Regulators recommend Figi over Cusip, Isin for reporting in FDTA proposal
Another contentious battle in the world of identifiers pits the Figi against Cusip and the Isin, with regulators including the Fed, the SEC, and the CFTC so far backing the Figi.
US Supreme Court clips SEC’s wings with recent rulings
The Supreme Court made a host of decisions at the start of July that spell trouble for regulators—including the SEC.