How NN IP uses machines to read the market—and itself
Dutch manager being acquired by Goldman uses machine learning to ‘augment’ its analysts
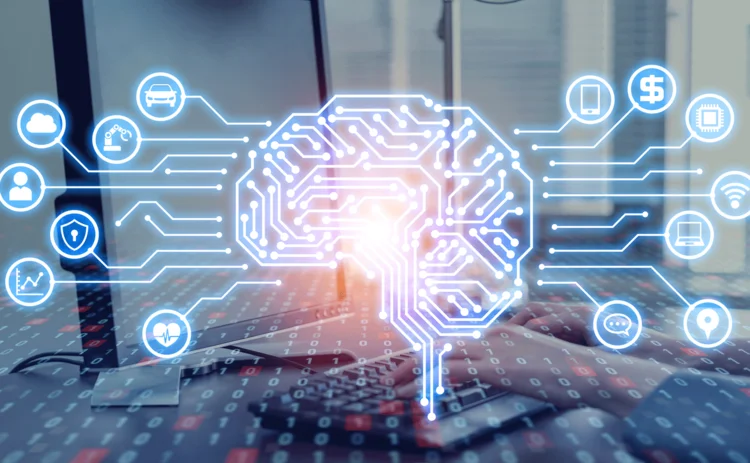
When energy companies began pumping bumper profits into a series of stock buybacks last year, analysts at NN Investment Partners had to decide whether to make a change to the firm’s dividend strategies. The funds use fundamental measures to pick undervalued companies that make regular payouts. If dividends were going out of style for energy companies, they may need to stop holding those stocks. So the analysts asked their quant colleagues to investigate whether energy companies that favoured dividends over buybacks still held value.
Using natural language processing (NLP), the quants were able to measure stock moves at times when announcements about dividends or buybacks occurred. The answer was yes.
This is an example of how quant and fundamental investing are mixing in the real world. Ambitious schemes based on complex quant signals are giving way to more practical and focused applications of data science, with the process led by the needs of fundamental analysts.
“We need to have an economic logic to incorporate a new signal in our process,” says Maarten Geerdink, who heads NN IP’s European equities team.
Geerdink joined the €298 billion ($335.6 billion) asset manager in 2018 shortly after the firm made a strategic pivot to so-called quantamental investing. The team he runs includes two quants on its staff of 19, managing about €6.5 billion ($7.4 billion) in long-only funds.
The shift to quantamental investing is something other asset managers are also trying. At the end of March, NN IP is due to be acquired by Goldman Sachs Asset Management, which itself has been a big pursuer of an edge from data in investing.
Like others, NN IP has pursued broader quant projects, too. The firm has built sentiment signals using news data, for example. But Geerdink and his team increasingly see NLP and other data science techniques as “tools” to “hunt alpha” in a more targeted fashion—devices to use in answering narrow, specific questions from the firm’s fundamental analysts.
A breakdown using NLP of earnings call transcripts during the early days of the Covid-19 pandemic, for example, showed a 12 standard-deviation fall in how much executives were referring to earnings, and a seven standard-deviation spike in talk about social issues. The exercise revealed a similar increase in firms talking about company culture on earnings calls, a nearly 20% uplift.
Equally, the team is turning its quant tools on itself. Geerdink’s unit runs an internal engine, Arrow, built with the input of behavioural analytics specialist Essentia Analytics, which monitors the actions of its own analysts and portfolio managers for bias.
Other firms have tried this too. The machine scoops up data on recommendations and trades and reports back to individuals where their idiosyncratic behaviour—holding losers too long or taking profits too soon—might be detracting from performance. “We’ve documented all this since I started,” Geerdink says.
At NN IP, the approach has shown that dividend fund portfolio managers tend to take on a value bias, Geerdink says, which can drag on performance if a manager also ignores market momentum.
“In a value fund, you have to cut your losers,” he says. Portfolio managers tend not to do that because they have bought stocks that seemed cheap and are slow to sell when those stocks seem to be getting cheaper.
Arrow highlights any such tilts and sends notifications to individuals to nudge them away from sub-optimal behaviours.
‘Oil and water’
Some of the pragmatism is born of necessity.
Global signals drawn from widely available data are often overtraded. In-house data by contrast is unique. “We wanted to keep track of everything, what everybody does, because that’s the only really proprietary data we have,” Geerdink says.
More pragmatic projects can be easier, too. NN IP has found that its NLP models require less human input for simpler jobs, such as determining what markets are focused on. The quants feed the model just five or six keywords and ask it to fill in the gaps using training data such as earnings call transcripts. The firm’s analysts and portfolio managers check the “extended” dictionary for anything nonsensical, and then the model effectively counts the occurrences of the wider terms in current data.
That contrasts with the heavy training burden when building more general NLP models to trawl text for signals of sentiment or something such as signs of corporate greenwashing, for example.
[Quant tools] empower analysts to do their work quicker, more efficiently, to be more robust. They create order from disorder
Maarten Geerdink, NN Investment Partners
This melding of man and machine can be difficult to pull off. At many firms, quants and data scientists working in silos sometimes build tools that miss the mark because they are sketchy on the details of what colleagues require. One investment manager has described mixing quants and fundamental analysts as like mixing “oil and water”.
To ensure NN IP’s data scientists follow the objectives of the firm’s fundamental investors closely, they form part of the same single team. In its 2018 pivot, the asset manager created a centralised “innovation platform”, Geerdink says, where quants work on data processing, data storage and keep up to speed with the latest technologies.
But the firm has deployed two-thirds of its quants among investing teams, where they now work directly with analysts and stock-pickers.
The portfolio managers determine what gets done. “The starting point is that the portfolio manager decides what they want to know—what’s relevant for the markets—and it’s the portfolio manager that interprets the outcome [from the models],” Geerdink says. Tellingly, he calls this “augmented” rather than quantamental investing.
Extra alpha
Has it worked? Geerdink says it has.
“We can prove that we’ve improved our investment process. It’s more robust. It generates more alpha,” he says. The European equities team’s three main strategies beat their benchmarks by 400 basis points last year on average.
Anecdotally, the analyst that covers energy enjoyed “stellar” outperformance, Geerdink says, and attributes that partly to insights from data science.
“I see that it works, and that people enjoy it and the overall results are better,” Geerdink says.
Geerdink says experience has taught him the combination of machines and humans will prove critical in the industry going forward. “I lived through the quant crisis in 2007,” he says. “But I’ve also seen that just running valuation-based fundamental investing on stocks, you’re prone to missing things as well.”
Quant tools “empower analysts to do their work quicker, more efficiently, to be more robust. They create order from disorder,” he says.
For an analyst to rank 25 or more companies on a given criteria would be “almost impossible”. NLP, though, can be “very good at giving you that kind of outcome”.
At NN IP, it will be stock-pickers that decide what questions get posed to the machines.
Further reading
Only users who have a paid subscription or are part of a corporate subscription are able to print or copy content.
To access these options, along with all other subscription benefits, please contact info@waterstechnology.com or view our subscription options here: http://subscriptions.waterstechnology.com/subscribe
You are currently unable to print this content. Please contact info@waterstechnology.com to find out more.
You are currently unable to copy this content. Please contact info@waterstechnology.com to find out more.
Copyright Infopro Digital Limited. All rights reserved.
As outlined in our terms and conditions, https://www.infopro-digital.com/terms-and-conditions/subscriptions/ (point 2.4), printing is limited to a single copy.
If you would like to purchase additional rights please email info@waterstechnology.com
Copyright Infopro Digital Limited. All rights reserved.
You may share this content using our article tools. As outlined in our terms and conditions, https://www.infopro-digital.com/terms-and-conditions/subscriptions/ (clause 2.4), an Authorised User may only make one copy of the materials for their own personal use. You must also comply with the restrictions in clause 2.5.
If you would like to purchase additional rights please email info@waterstechnology.com
More on Emerging Technologies
This Week: Startup Skyfire launches payment network for AI agents; State Street; SteelEye and more
A summary of the latest financial technology news.
Waters Wavelength Podcast: Standard Chartered’s Brian O’Neill
Brian O’Neill from Standard Chartered joins the podcast to discuss cloud strategy, costs, and resiliency.
SS&C builds data mesh to unite acquired platforms
The vendor is using GenAI and APIs as part of the ongoing project.
Chevron’s absence leaves questions for elusive AI regulation in US
The US Supreme Court’s decision to overturn the Chevron deference presents unique considerations for potential AI rules.
Reading the bones: Citi, BNY, Morgan Stanley invest in AI, alt data, & private markets
Investment arms at large US banks are taken with emerging technologies such as generative AI, alternative and unstructured data, and private markets as they look to partner with, acquire, and invest in leading startups.
Startup helps buy-side firms retain ‘control’ over analytics
ExeQution Analytics provides a structured and flexible analytics framework based on the q programming language that can be integrated with kdb+ platforms.
The IMD Wrap: With Bloomberg’s headset app, you’ll never look at data the same way again
Max recently wrote about new developments being added to Bloomberg Pro for Vision. Today he gives a more personal perspective on the new technology.
LSEG unveils Workspace Teams, other products of Microsoft deal
The exchange revealed new developments in the ongoing Workspace/Teams collaboration as it works with Big Tech to improve trader workflows.