JP Morgan is testing quantum deep hedging
Researchers say the timeline has shortened for the use of models in production.
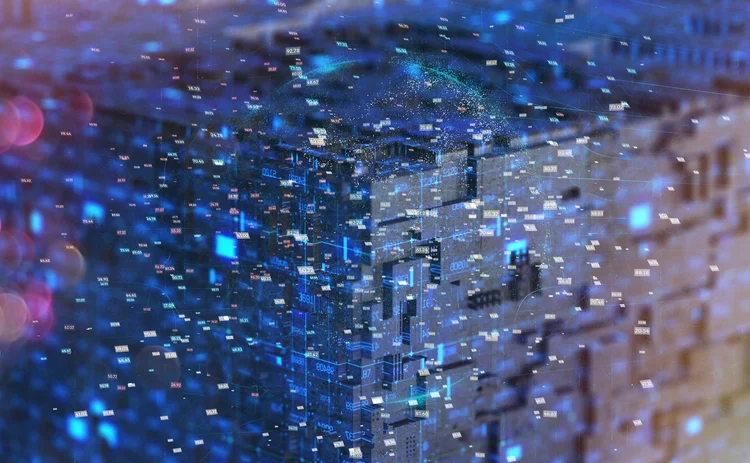
Quantum computing could nearly double the effectiveness of machine learning-based hedging strategies, according to researchers at JP Morgan.
The US bank worked with quantum software company QC Ware to complete a study of quantum deep hedging detailed in a new paper, Quantum Deep Hedging, released March 30.
“Now we actually have a solution that gives us confidence that in the future this work will be usable in production,” says Marco Pistoia, head of global technology applied research at JP Morgan Chase.
Deep hedging uses machine learning to work out from raw data how to hedge derivatives books, factoring in real-world market frictions such as transaction costs. The technique has been shown to perform 6–7% better than conventional models such as Black Scholes, measured in terms of profit and loss, according to Iordanis Kerenidis, head of quantum algorithms at QC Ware. The quantum version can improve performance by 11% over such models, he says.
Now we actually have a solution that gives us confidence that in the future this work will be usable in production,
Marco Pistoia, JP Morgan
Using a quantum computer allowed the researchers to train the deep hedging model with more training scenarios than has been possible before.
Units of data in classical computers – bits – exist in one of two states, allowing computers to store data in ones and zeroes. Quantum computers are far more powerful. Quantum bits – ‘qubits’ – exist in multiple states simultaneously, meaning quantum computers can manage vast sets of calculations.
JP Morgan’s existing deep hedging engine looks at what could happen to the price of a stock or option over a period of time – say, 10 days – and decides when to buy or sell. Computational limits mean the model can consider only a subset of possible “futures”, however – perhaps 1,000 – and must aggregate them to come up with the best strategy, Kerenidis says. A quantum model can look at all possible futures at the same time.
“We don’t look only at the most possible scenario, but at all scenarios at the same time, in parallel,” Kerenidis says.
JP Morgan’s quants tested the idea using a quantum “actor-critic” reinforcement learning model. In such models, the actor element generates strategies that the critic element evaluates, optimising through multiple iterations given possible future scenarios.
The quantum circuits used were small in size and the model limited to short-dated options, a restriction imposed because of the need to simulate the training on a classical computer for benchmarking. In principle, however, a quantum model could be trained directly on a quantum computer with existing hardware, or hardware that is expected to be available in the next few years, for a maturity time of a month or more, the researchers say.
Models could be implemented on so-called noisy intermediate-scale quantum (Nisq) hardware, Kerenidis says, a term for the current generation of quantum machines.
JP Morgan developed deep hedging in 2018 as an upgrade to conventional parametric models, which produce outputs that traders must repeatedly adjust to account for real-world market frictions. The technique uses a neural network to learn the optimal strategy to hedge an options book from historical and synthesised pricing and market data. JP Morgan has put the model to work hedging vanilla options and more recently cliquet options that have more complex path-dependent payoffs.
Last year, the bank rolled out a new version of the model that learns how to hedge generally, meaning it can be trained to hedge any book of options, rather than a specific portfolio. This made it faster and easier to apply at scale.
Around that time, Pistoia says there was potential to reduce the amount of approximation required in machine learning through quantum computing, but he said deep hedging was complex and current quantum computers might not be powerful enough. Pistoia says the quantum computers available a year ago would not have made possible a small experiment such as the one just undertaken, but hardware is advancing at a fast pace.
JP Morgan is using Honeywell joint venture Quantinuum’s “trapped ion” hardware which has the largest quantum volume of qubits, at 32,768.
Kerenidis says over the course of the past year the algorithms used have also improved. “We have shortened the timeline a lot to be able to actually productise this in the near future,” he says.
The researchers say quantum reinforcement learning methods have applications beyond deep hedging, for example for algorithmic trading or option pricing.
Banks are taking a close interest in quantum computers, believing the technology could be used in regulatory stress tests, derivatives pricing, fraud detection and encrypting data.
Pistoia says the focus of JP Morgan’s latest work was less on speeding up models and more on getting higher quality results.
As of end-2022, JP Morgan Chase employed over 55,000 technologists globally and annually spent $14 billion on tech.
Only users who have a paid subscription or are part of a corporate subscription are able to print or copy content.
To access these options, along with all other subscription benefits, please contact info@waterstechnology.com or view our subscription options here: http://subscriptions.waterstechnology.com/subscribe
You are currently unable to print this content. Please contact info@waterstechnology.com to find out more.
You are currently unable to copy this content. Please contact info@waterstechnology.com to find out more.
Copyright Infopro Digital Limited. All rights reserved.
As outlined in our terms and conditions, https://www.infopro-digital.com/terms-and-conditions/subscriptions/ (point 2.4), printing is limited to a single copy.
If you would like to purchase additional rights please email info@waterstechnology.com
Copyright Infopro Digital Limited. All rights reserved.
You may share this content using our article tools. As outlined in our terms and conditions, https://www.infopro-digital.com/terms-and-conditions/subscriptions/ (clause 2.4), an Authorised User may only make one copy of the materials for their own personal use. You must also comply with the restrictions in clause 2.5.
If you would like to purchase additional rights please email info@waterstechnology.com
More on Emerging Technologies
This Week: Startup Skyfire launches payment network for AI agents; State Street; SteelEye and more
A summary of the latest financial technology news.
Waters Wavelength Podcast: Standard Chartered’s Brian O’Neill
Brian O’Neill from Standard Chartered joins the podcast to discuss cloud strategy, costs, and resiliency.
SS&C builds data mesh to unite acquired platforms
The vendor is using GenAI and APIs as part of the ongoing project.
Chevron’s absence leaves questions for elusive AI regulation in US
The US Supreme Court’s decision to overturn the Chevron deference presents unique considerations for potential AI rules.
Reading the bones: Citi, BNY, Morgan Stanley invest in AI, alt data, & private markets
Investment arms at large US banks are taken with emerging technologies such as generative AI, alternative and unstructured data, and private markets as they look to partner with, acquire, and invest in leading startups.
Startup helps buy-side firms retain ‘control’ over analytics
ExeQution Analytics provides a structured and flexible analytics framework based on the q programming language that can be integrated with kdb+ platforms.
The IMD Wrap: With Bloomberg’s headset app, you’ll never look at data the same way again
Max recently wrote about new developments being added to Bloomberg Pro for Vision. Today he gives a more personal perspective on the new technology.
LSEG unveils Workspace Teams, other products of Microsoft deal
The exchange revealed new developments in the ongoing Workspace/Teams collaboration as it works with Big Tech to improve trader workflows.