JP Morgan pulls plug on deep learning model for FX algos
The bank has turned to less complex models that are easier to explain to clients.
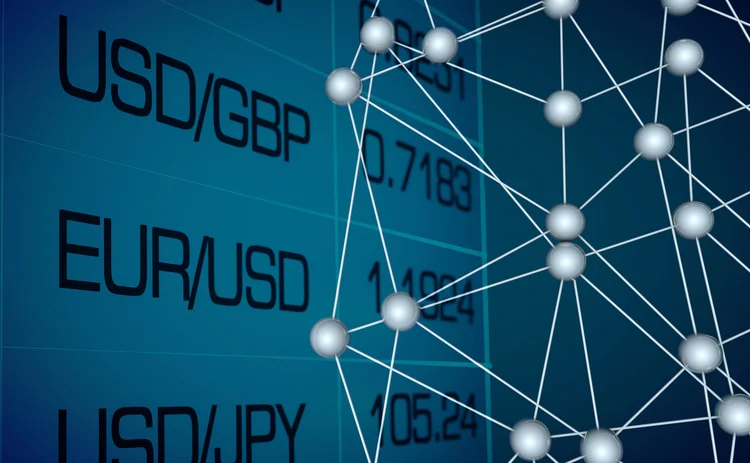
JP Morgan has phased out a model that leverages machine learning technology for foreign exchange algorithmic execution, citing issues with data interpretation and the complexity involved.
The US bank had implemented what it calls a deep neural network for algo execution (DNA), which uses a machine learning framework to optimize order placement and execution styles to minimize market impact.
Launched in 2019, JP Morgan said at the time that the move would replicate reinforcement learning techniques used for trading equities. After several years of running the model, however, the bank decided to wind it down.
“We used [DNA] for venue selection and some of the placement options within the algo. But that has now been phased out,” said Carlos Gomez Gascon, head of macro algorithmic execution at JP Morgan, speaking at the FX Markets USA event on October 17.
We were able to extract the ideas of the modelling behind the scenes into simpler, classical models which were more consistent
Carlos Gomez Gascon, JP Morgan
“The reason for that was because we were able to draw out a lot of the conclusions for the artificial intelligence [AI] into more deterministic behavior that was more consistent and robust.”
Many banks have sought to develop a new generation of FX algos that use machine learning or AI technology to improve the quality of order fill rates or respond to real-time market movements.
JP Morgan’s DNA framework differed in its plan to create one algorithm backed by reinforcement learning. By using deep data pools on past trades to simulate multiple market scenarios, the technology would train the algo to learn from various scenarios to determine the best way to execute a client order.
This also marked a shift from early generation algos, which were primarily built using human programming or rule-based executions.
But deep neural networks and complex machine learning models can pose challenges for algo providers when interpreting the data. Gomez Gascon said it proved difficult to explain the past performance of the algo to clients. Instead, the bank determined it was able to extract the same conclusions using less complex models.
“From a performance perspective, we were able to extract the ideas of the modeling behind the scenes into simpler, classical models which were more consistent,” said Gomez Gascon.
“There’s always a risk/reward—if you’re running higher complexity, there has to be a reason why you’re running that," he said. “That complexity… was great at the time because it enabled us to increase the performance, [but] we were able to get the same performance with a lower level of complexity.”
Swayam Thacker, Americas head of fixed income, currencies and commodities trading solutions at Goldman Sachs, said there are trade-offs between the complexity of the technology behind an algo and its performance.
“There are trade-offs with going more complex and there are trade-offs with going too simple. The exact use case that you’re trying to solve matters a lot, the context matters a lot, and its interpretability,” said Thacker, speaking on the same panel.
Buy-side firms are also somewhat reluctant to completely rely on AI for trade execution, according to Phillippe Burke, a portfolio manager at Apache Capital Management. While the firm uses a variety of models for its trades, when it comes to execution it still requires human judgement on whether the trade will improve the portfolio.
Burke, who was also speaking on the panel, added that firms must be careful when using machine learning models that rely largely on historical data sets.
“It’s not uncommon that when you start out in machine learning, you get very excited about the possibilities and you start using more and more complex models. But then you revert back to using simpler models,” he said.
Only users who have a paid subscription or are part of a corporate subscription are able to print or copy content.
To access these options, along with all other subscription benefits, please contact info@waterstechnology.com or view our subscription options here: http://subscriptions.waterstechnology.com/subscribe
You are currently unable to print this content. Please contact info@waterstechnology.com to find out more.
You are currently unable to copy this content. Please contact info@waterstechnology.com to find out more.
Copyright Infopro Digital Limited. All rights reserved.
As outlined in our terms and conditions, https://www.infopro-digital.com/terms-and-conditions/subscriptions/ (point 2.4), printing is limited to a single copy.
If you would like to purchase additional rights please email info@waterstechnology.com
Copyright Infopro Digital Limited. All rights reserved.
You may share this content using our article tools. As outlined in our terms and conditions, https://www.infopro-digital.com/terms-and-conditions/subscriptions/ (clause 2.4), an Authorised User may only make one copy of the materials for their own personal use. You must also comply with the restrictions in clause 2.5.
If you would like to purchase additional rights please email info@waterstechnology.com
More on Emerging Technologies
This Week: Startup Skyfire launches payment network for AI agents; State Street; SteelEye and more
A summary of the latest financial technology news.
Waters Wavelength Podcast: Standard Chartered’s Brian O’Neill
Brian O’Neill from Standard Chartered joins the podcast to discuss cloud strategy, costs, and resiliency.
SS&C builds data mesh to unite acquired platforms
The vendor is using GenAI and APIs as part of the ongoing project.
Chevron’s absence leaves questions for elusive AI regulation in US
The US Supreme Court’s decision to overturn the Chevron deference presents unique considerations for potential AI rules.
Reading the bones: Citi, BNY, Morgan Stanley invest in AI, alt data, & private markets
Investment arms at large US banks are taken with emerging technologies such as generative AI, alternative and unstructured data, and private markets as they look to partner with, acquire, and invest in leading startups.
Startup helps buy-side firms retain ‘control’ over analytics
ExeQution Analytics provides a structured and flexible analytics framework based on the q programming language that can be integrated with kdb+ platforms.
The IMD Wrap: With Bloomberg’s headset app, you’ll never look at data the same way again
Max recently wrote about new developments being added to Bloomberg Pro for Vision. Today he gives a more personal perspective on the new technology.
LSEG unveils Workspace Teams, other products of Microsoft deal
The exchange revealed new developments in the ongoing Workspace/Teams collaboration as it works with Big Tech to improve trader workflows.