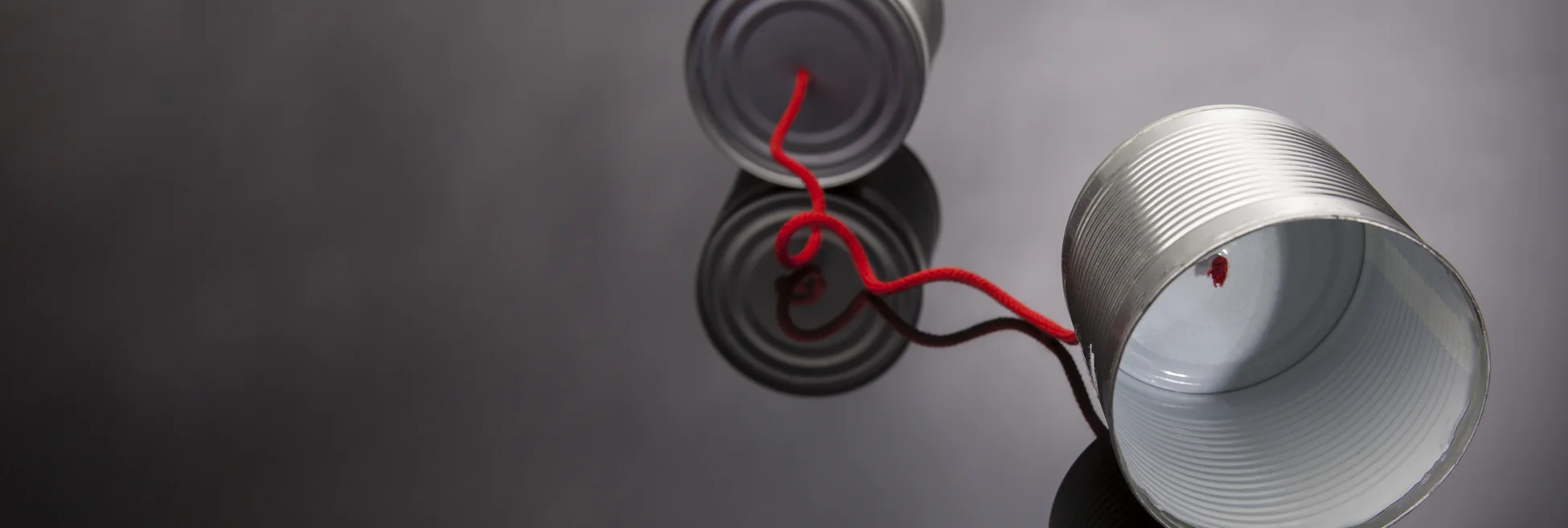
Listen Up: How Tech is Turning Voice into a Valuable Dataset
Voice is arguably one of the most interesting—yet largely untapped—alternative datasets in existence today.
Need to know
Rebecca Natale explores how new technologies are allowing firms to capture what people say and quickly turn that information into actionable insights.
In advance of launching the Disney+ streaming service, the Walt Disney Company quarterly earnings call on November 7 was heavily anticipated. As expected, earnings fell but managed to beat analyst expectations, though revenue was below consensus estimates. Prattle, an alternative data provider that was recently bought by Liquidnet, scored the sentiment of the earnings call as slightly above average, landing in the 55th percentile compared to all prior earnings calls from Disney.
Prattle’s score was driven by positive comments in the prepared remarks by CFO Christine McCarthy.
“We expect the continued investment in our DTC services, specifically Disney+, which will launch in just a few days, and the consolidation of Hulu to drive an adverse impact on the year-over-year change in segment operating income of our direct-to-consumer businesses of approximately $850 million,” she said. The comment encouraged analysts to focus on the prospect of the new Disney+ business line, and explained away any negative accounting as transient.
Prattle observed the Q&A portion of the call was also slightly positive. Despite missing the revenue target and barely beating earnings, Disney’s stock rose 5% at the market open on November 8.
The effect of words can extend well beyond the room—or in this case, phone line—in which they are spoken. An executive’s language can tell a trader or analyst more than what meets the ear. In business and trading, what is said is proving to be valuable information—and firms are leveraging technology, such as natural-language processing (NLP), to capture hidden meanings, find alpha, and augment tedious tasks.
The most natural use for the analysis of such communications has been compliance and surveillance and, closely related to that, trade reconstruction, a post-trade process that involves gathering all the phone, text and system records from one trade, usually for the purpose of handing over to a regulator.
These hinge on a firm’s ability to stitch together vast amounts of structured and unstructured data to produce one result, says Danielle Tierney, a senior compliance and surveillance advisor at Greenwich Associates. For the use of voice in actual investment and trading, that need holds true—and the technology that can intertwine the two has several use cases.
Finding a Fit
“The first thing that comes to mind is earnings conference calls,” Tierney says. “If you had a system with the technological ability to apply natural-language analysis to those calls, then that’s something else entirely. A lot of surveillance algorithms for natural-language analysis detect tone [and] things like that. And internally, it could be useful for being able to search your own firm’s prior investment decisions.”
Since the financial crisis in 2008, banks and asset managers have been working to diversify their portfolios through multi-asset trading, she adds, contributing to a boom in trade and, especially, quote data. In the thick of it, firms want to leave no stone unturned when it comes to finding alpha—even if to gain just a percentage point over their peers.
That’s exactly where Anthony Tassone, founder and CEO of GreenKey Technologies, finds an opportune chance to play ball.
“The problem with thinking of voice as just trade surveillance is … that’s a very narrow view of how fraud gets done and how bad behavior gets done,” he says. “It’s not realistic to say that trade surveillance is the only use case for NLP, and I’m not sure that it’s even the best one. I think that voice as an interface to drive automation, and voice as a source of data and analytics to drive revenue are far bigger priorities right now.”
Right now, GreenKey is busy analyzing audio files for users. A common request is for the vendor to take about six months of audio across different desks, such as fixed income, credit or emerging market bonds, and analyze those calls and conversations. After that, the hope is that firms can understand what quotes occurred versus what trades, and why certain quotes do or don’t turn into trades.
“Did they not have enough size and a better offer? Did they not have the product that the customer was asking for? Was the response time too slow?” Tassone says. And shifting demographics on trading desks don’t make those questions any easier to answer. The number of seats at trading desks has shrunk in recent years; veterans in the space retire or are let go; the rise of chat bots, which continue to get more sophisticated, has created more data to sift through.
After chat bots, Tassone says voice assistants—like Apple’s Siri or Amazon’s Alexa, but which can’t understand and speak technical trading jargon—are not that far behind. “Really, what they’re building are the future little market-makers and baby traders of the future,” he says.
Voice as Alternative Data
Before tapping into voice, text had to be conquered first.
Liquidnet is currently in the process of building out a new business unit targeted at long-term investors, dubbed Investment Analytics, which is the culmination of its last three acquisitions: OTAS, RSRCHXchange, and Prattle.
Adam Sussman, head of market structure and liquidity partnerships at Liquidnet, is one of those heading up the initiative, and on his mind is automation. He adds that NLP-based technologies stand to play a role in automating not just execution, but—someday—the whole investment strategy, though first machines will have to wrangle with shorter investment timeframes.
“There are a lot of variables and a lot of unknown things that machines are just not good at handling,” Sussman says. “But what this type of automation can do is help focus the analysts and portfolio managers on companies and datasets where something significant has happened.”
As an example, he offers the corpus of regulatory reports that each company must file with the SEC each quarter, and the volume of literature published about how to decode their hidden meanings. “It takes people years to read through them. … So one of the things we’re working on is how do we leverage natural language processing to help point analysts and portfolio managers to those documents where opportunities are most likely to appear.”
It would still be the analyst’s and manager’s jobs to then go read them, and decide whether or not a document was helpful, but as of right now, Sussman says there isn’t really a technology that helps them find the diamonds in the rough.
For furthering its capabilities in NLP and NLP’s cousin, natural-language generation (NLG), Liquidnet will turn to Prattle, whose flagship analytics platform measures sentiment and predicts market impact using NLP on unstructured data, such as company earnings calls and company filings.
Prattle’s process is mathematical, and works by looking at the words and phrases used by a company’s executives and then tracking how the stock price moves from quarter to quarter. Then the company removes all other factors that might have led to price fluctuation, boiling it right down to the directly impactful language.
“It’s really about the patterns of the words and how they map to the price in the past,” Sussman says.
For example, a CEO can serve for any number of years, but their time in their role always comes to an end, and in their place appears a new person. “A frequent question to us is, how does that impact [the company]?’ What studies have shown is that the person adapts to the role; the person doesn’t change the role. These companies have investor relationship officers, who are the ones that guide the language that the company uses to communicate out to the investor public. And even if we switch from CEO A to CEO B, CEO B gets trained to use the same language as CEO A.”
Behind the Scenes
Though there is a certain science to, for example, analyzing an earnings call and determining value in it, transcription can be trickier, particularly in real-time. Before a company can even apply NLP, the model has to first transcribe a conversation into something readable. But that ingestion and interpretation requires a lot of man hours, not only to understand what’s said at face value, but also the nuanced, technical nature of trading jargon.
NICE Actimize, founded in 1999, is another firm using its expertise in surveillance and compliance technology to generate business insights for managers and supervisors to better understand the conversations their employees are having with clients. Steve LoGalbo, director of communications surveillance product management at NICE Actimize, says his company’s models require a boatload of data—and luckily, they have it.
“Having this experience in compliance has enabled us to really teach these models how to understand the financial services lingo and how to understand the different trading desks we’re monitoring conversations for,” LoGalbo says. “There are a lot of similar-sounding words, right? ‘USD’ is a simple example. If somebody says ‘USD’ in an FX conversation, you want the model to output US dollar, not the word ‘you’ and then ‘SD’ or something. Same as if you hear ‘FX’—[a] model might output ‘effects,’ but it’s actually the acronym.”
Then they have to teach the NLP model how to understand relevant information, LoGalbo says, such as company names, products, asset classes or currencies. And given that trading and finance is a global business, there’s another layer to account for: different languages.
NICE Actimize is expanding its presence in Asia-Pacific at the moment, and the company is increasingly having to train its models with languages other than English or common European ones. They have some experience with Japanese, for example, but notice a rising demand for Cantonese.
“There’s a lot of mixed English and Chinese in those conversations,” LoGalbo says. “Building a new language model requires getting audio files from a diverse set of speakers, genders, dialects and accents. Then you have to physically transcribe those calls to map the sound to the words. Just to give you an idea, it takes two working days to transcribe one hour of recording.”
To have a highly-effective model, they need 30 hours to even get to the baseline. The process takes up to a couple months just to transcribe all the audio that allows for a good acoustic and transcription model. Next they go to work on building an NLP capability that knows the entities of interest they want to monitor for.
Prime Prospects
Primarily, the compliance data NICE Actimize gathers consists of firms’ phone and chat logs, but they’re working on a solution with clients that would capture and analyze the conversations that take place in a firm’s office. In addition to compliance, an opportunity could be even more operational alpha and better business insights, particularly for more non-regulated entities.
“For regulated users, we already have all of this data. Then there’s the non-regulated users, who are also having conversations and have data that isn’t necessarily being captured,” LoGalbo says. “Maybe a meeting room conversation is an example. So do you mic those meeting rooms, and can you actually capture that data and store it? Then what to do you do with it once you have it? Is it now a record that you need to keep? Are there any GDPR issues to think about? Some firms are questioning if they’re even allowed to use this compliance data for this purpose. Or is it really business data that we’re free to use?”
While there’s opportunity, it may be a double-edged sword. “If you’re analyzing the full conversation, and you happen to be storing some results of that analytics, which happen to be a regulatory problem or compliance issue you weren’t looking at, and it turns up somewhere—we have to be a bit careful about what we’re storing and listening for,” he says.
Adoption of voice surveillance technology still has a fair way to go on both the buy and sell sides. According to a chart published in July by consultancy and research firm Opimas, the majority of both still rely on spot checking done by a human—98% of full-service global investment banks and 75% of hedge funds, with that figure decreasing with assets under management. After interviews with more than 140 compliance teams, they concluded that advanced analytics for surveillance, including automated voice-to-text, voice biometrics, emotion detection and auto-language detection, all hover between 0% and 20%.
But these numbers might be prime to increase due to regulatory needs and more willingness to turn to technology to drive automation. Should more firms move further into the surveillance tech realm, they may find that their own voices and communications—and unlocking them—are more of an asset than they think.
Only users who have a paid subscription or are part of a corporate subscription are able to print or copy content.
To access these options, along with all other subscription benefits, please contact info@waterstechnology.com or view our subscription options here: http://subscriptions.waterstechnology.com/subscribe
You are currently unable to print this content. Please contact info@waterstechnology.com to find out more.
You are currently unable to copy this content. Please contact info@waterstechnology.com to find out more.
Copyright Infopro Digital Limited. All rights reserved.
As outlined in our terms and conditions, https://www.infopro-digital.com/terms-and-conditions/subscriptions/ (point 2.4), printing is limited to a single copy.
If you would like to purchase additional rights please email info@waterstechnology.com
Copyright Infopro Digital Limited. All rights reserved.
You may share this content using our article tools. As outlined in our terms and conditions, https://www.infopro-digital.com/terms-and-conditions/subscriptions/ (clause 2.4), an Authorised User may only make one copy of the materials for their own personal use. You must also comply with the restrictions in clause 2.5.
If you would like to purchase additional rights please email info@waterstechnology.com
More on Data Management
New working group to create open framework for managing rising market data costs
Substantive Research is putting together a working group of market data-consuming firms with the aim of crafting quantitative metrics for market data cost avoidance.
Off-channel messaging (and regulators) still a massive headache for banks
Waters Wrap: Anthony wonders why US regulators are waging a war using fines, while European regulators have chosen a less draconian path.
Back to basics: Data management woes continue for the buy side
Data management platform Fencore helps investment managers resolve symptoms of not having a central data layer.
‘Feature, not a bug’: Bloomberg makes the case for Figi
Bloomberg created the Figi identifier, but ceded all its rights to the Object Management Group 10 years ago. Here, Bloomberg’s Richard Robinson and Steve Meizanis write to dispel what they believe to be misconceptions about Figi and the FDTA.
SS&C builds data mesh to unite acquired platforms
The vendor is using GenAI and APIs as part of the ongoing project.
Aussie asset managers struggle to meet ‘bank-like’ collateral, margin obligations
New margin and collateral requirements imposed by UMR and its regulator, Apra, are forcing buy-side firms to find tools to help.
Where have all the exchange platform providers gone?
The IMD Wrap: Running an exchange is a profitable business. The margins on market data sales alone can be staggering. And since every exchange needs a reliable and efficient exchange technology stack, Max asks why more vendors aren’t diving into this space.
Reading the bones: Citi, BNY, Morgan Stanley invest in AI, alt data, & private markets
Investment arms at large US banks are taken with emerging technologies such as generative AI, alternative and unstructured data, and private markets as they look to partner with, acquire, and invest in leading startups.