Machine Learning: A Math Problem or a Workflow Problem?
For good reason, machine learning has a highly technical focus. But less talked-about challenges lie in managing the human capital and workflows associated with the tech.
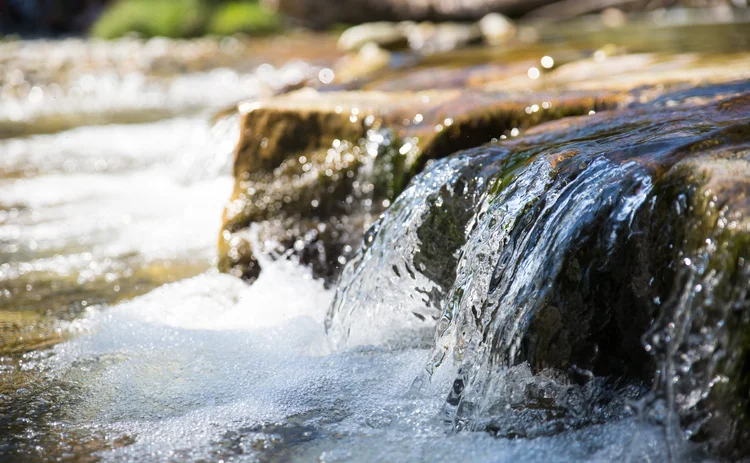
As machine learning (ML) use-cases expand to include building risk models and trading algorithms, and to finding connections in a fog of data, capital markets firms are also experiencing growing pains when it comes to building some semblance of structure around what can be largely experimental artificial intelligence (AI) projects.
This is true of many jobs that require specific skillsets, but it’s common for a talented machine-learning engineer to get sidetracked with issues that don’t involve the actual building and training of models, providing an architecture around those models, and then deploying the models. The people who are best at building ML functions often end up getting waylaid by front-end web design, data validation, or, worse yet, arguing with business managers over product strategy and return on investment (ROI), says the head of machine-learning engineering at a data provider.
“Often, companies don’t even know what machine learning is. You’ll have some manager say, ‘Hey, I put a million bucks into AI last year; what did it get me? I don’t know—nothing? So why should I put another million bucks into it?’” they say.
Inevitably, support at the C-level wanes, talented engineers get tired of not working on actual projects or of simply putting fixes in place to myriad requests and problems, which leads to talent attrition, and before long, the ML team is back to square one, says the engineer.
Robert Cooke, founder and CTO of data management vendor 3Forge, says misunderstanding of machine learning and machine-learning engineers holds trading firms back from the efficiency those tools are intended to provide.
“Whenever I work with these engineers I ask them: What percent of the time do you think you’re actually focusing on this machine-learning thing? And sometimes they’ll laugh and say never,” Cooke says.
3Forge works with large sell-side banks and proprietary trading firms, helping them to manage and monitor their trading systems that use machine-learning algorithms. Cooke is often involved with firms’ greenfield projects.
Cooke says 3Forge has run studies that show that about 70% of machine-learning costs are spent on hiring human capital and IT staff. Despite the price tag, those engineers end up spending about 20% of their time on actual programming and ML modeling. About 80% of their time is spent building the front-ends, scraping log files, validating data, and producing P-factors, which constitute profiles for each instrument or stock.
So, for example, a machine-learning system might cover 10,000 instruments, for each of which the system produces hundreds of numbers that indicate market share, volatility, and other factors that make up a stock, Cooke says.
“Imagine that these machine-learning models are producing those numbers, putting them into a database, putting them into a file, and no one can see them. No one has any idea what’s going on with those numbers, and then trades start coming in,” Cooke says. “And the models are using those pre-computed numbers to determine how to trade stocks. And this is the real world that I think a lot of us still live in.”
3Forge built its flagship analytics platform, AMI, as a front-end where users can see all the different factors—more of which are continually being added—to help high-frequency traders better understand why the machines are making certain trading decisions, and quickly override it if the decisions are wrong ones.
Perhaps, though, the biggest adversary to machine-learning engineers is the time spent fighting production issues like outages. In the IT space, Cooke says, so much time is wasted when firms bring in high-end technical staff, but lack the fundamental tooling developers need, though he thinks it is “most egregious” in the field of machine learning.
Engineers are often the ones providing feedback into the system, a role that requires understanding of the stock market and trading world. As a result, the best machine-learning experts in finance often become financial experts, though that’s not necessarily a good thing.
“It should be that they’re focusing on the models, and the people who are experts in the field should be providing that input,” Cooke says.
This year, 3Forge is working on tighter integrations with firms’ machine-learning models, so that instead of having a generic API, firms can more easily deploy their models directly into the platform. At the same time, the business is focusing on finding better ways to calculate and display constantly growing datasets.
Further reading
Only users who have a paid subscription or are part of a corporate subscription are able to print or copy content.
To access these options, along with all other subscription benefits, please contact info@waterstechnology.com or view our subscription options here: http://subscriptions.waterstechnology.com/subscribe
You are currently unable to print this content. Please contact info@waterstechnology.com to find out more.
You are currently unable to copy this content. Please contact info@waterstechnology.com to find out more.
Copyright Infopro Digital Limited. All rights reserved.
As outlined in our terms and conditions, https://www.infopro-digital.com/terms-and-conditions/subscriptions/ (point 2.4), printing is limited to a single copy.
If you would like to purchase additional rights please email info@waterstechnology.com
Copyright Infopro Digital Limited. All rights reserved.
You may share this content using our article tools. As outlined in our terms and conditions, https://www.infopro-digital.com/terms-and-conditions/subscriptions/ (clause 2.4), an Authorised User may only make one copy of the materials for their own personal use. You must also comply with the restrictions in clause 2.5.
If you would like to purchase additional rights please email info@waterstechnology.com
More on Trading Tech
Recent volatility highlights tech’s vital role in fixed income pricing
MarketAxess’ Julien Alexandre discusses how cutting-edge technology is transforming pricing and execution in the fixed income market amid periodic bouts of volatility
Banks fret over vendor contracts as Dora deadline looms
Thousands of vendor contracts will need repapering to comply with EU’s new digital resilience rules
Where have all the exchange platform providers gone?
The IMD Wrap: Running an exchange is a profitable business. The margins on market data sales alone can be staggering. And since every exchange needs a reliable and efficient exchange technology stack, Max asks why more vendors aren’t diving into this space.
This Week: Trading Technologies completes ANS deal; State Street; Equinix; and more
A summary of the latest financial technology news.
Interactive Brokers looks beyond US borders for growth opportunities
As retail trading has grown in volume and importance, Interactive Brokers and others are expanding international offerings and marketing abroad.
JP Morgan’s goal of STP in loans materializes on Versana’s platform
The accomplishment highlights the budding digitization of private credit, though it’s still a long road ahead.
As data volumes explode, expect more outages
Waters Wrap: At least for those unprepared—though preparation is no easy task—says Anthony.
This Week: ICE Bonds and MarketAxess plan to connect liquidity networks, TS Imagine, Bloomberg, and more
A summary of the latest financial technology news.