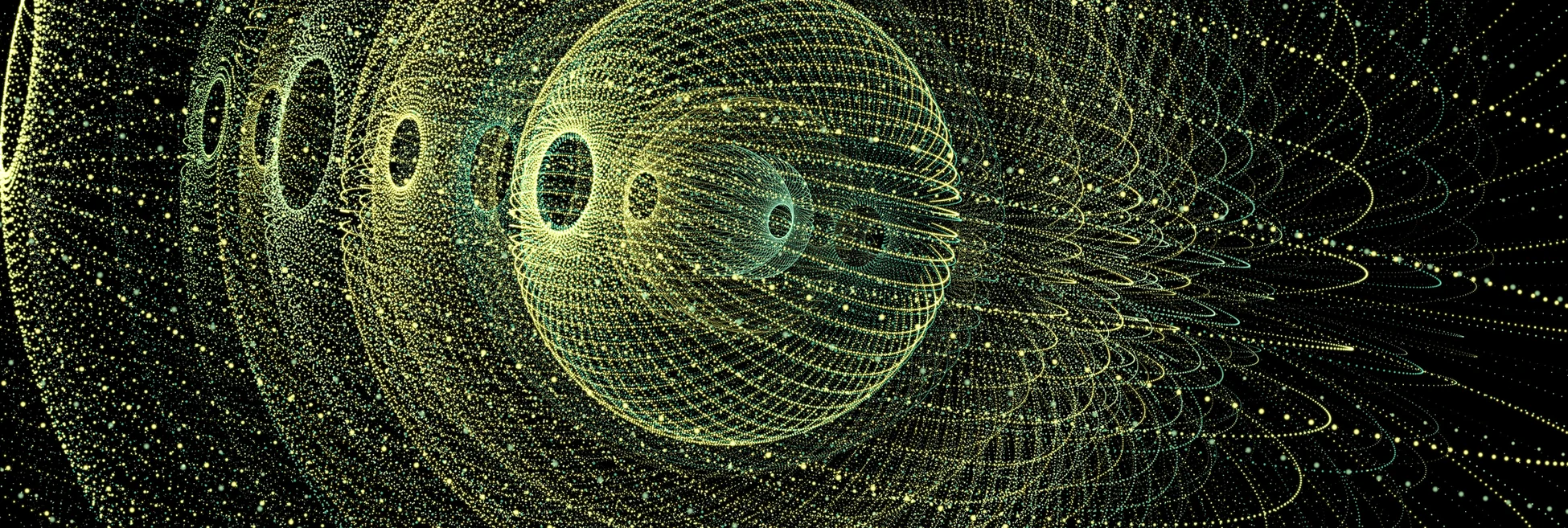
Machine learning & NLP in the capital markets: Some examples from 2021
To show how ML and NLP are spreading across the industry, WatersTechnology highlights 20 stories from the last 12 months that feature unique uses of AI.
As firms demand more data to find alpha, better manage risk, and more efficiently handle regulatory needs, they are having to turn to artificially-intelligent tools, namely, algorithms that incorporate the disciplines of machine learning and natural language processing.
Below are some examples as to how banks, asset managers, exchanges, regulators and vendors are using ML and NLP. While this is not an exhaustive list of stories we wrote about AI in 2021, we hope it provides a good snapshot of some of the more interesting projects currently underway in the capital markets.
BlackRock
Quants are using machine learning-based natural language processing engines to find alpha signals in text, or help fundamental analysts cut through informational noise. Training the models to make sense of financial language, however, is labor-intensive. While doing the job badly can lead to wasted time and effort; few shortcuts exist.
BlackRock has been working on its sentiment model for five years. Simple elements of training, such as mapping company names to stock tickers, can also go awry. Haris Chalvatzis, a researcher in the firm’s core portfolio management team, describes automating the mapping process as an “open problem” in NLP and says none of the mechanical solutions is close to perfect. “It sounds like an easy thing to do, but in fact is not straightforward,” he says. Apple is the obvious example. A computer struggles to work out whether a document refers to the company or the fruit. Bloomberg news comes ready-tagged. But in other articles, “it’s kind of difficult,” says Calvatzis; “not impossible, but you do get false positives”.
BlackRock is also using machine learning to improve its ESG investment process.
RBC
In the fall of 2020, RBC Capital Markets launched its AI-powered trading platform, Aiden. It uses deep reinforcement learning to, for instance, calculate volume-weighted average price (VWap) and to reduce slippage against the VWap benchmark.
In 2021, RBC built off its VWap algo and is developing a second algo that targets arrival price, which is the midpoint between the bid/ask prices at the time an order is placed. It can be difficult to hit that midpoint because as a trader starts buying up certain shares, sellers can get a sense of that movement, causing the price to rise and effectively minimizing the alpha that a portfolio manager wants to capture for their own clients. Though RBC’s live or in-testing implementations of deep reinforcement learning are currently limited to execution, the firm is also considering how it can be used to navigate the fragmented network of liquidity pools and how to best deliver timely and consumable information to clients.
While Google wears a lot of hats when it comes to advancements in the field of machine learning—and its experience in the area was a leading driver behind the partnership with CME Group—the tech giant believes its AI tools have a multitude of applications across some of the complex data challenges of financial markets, and it’s starting with the manual and complicated world of corporate actions. Some believe it will be an uphill battle.
JP Morgan
JP Morgan Asset Management is using an artificial intelligence tool to help its internal portfolio managers and analysts build thematic funds. The tool, called ThemeBot, was created to help JPMAM’s portfolio managers generate a list of stocks associated with a theme. For example, it was used to construct JPMAM’s Genetic Therapies Fund, which aims to provide diversified exposure to companies in developed and emerging markets working on genetic treatments to address the underlying cause of diseases. Since the fund’s inception in October 2019, it has grown about 58% to a market value of $1.3 billion.
Yazann Romahi, managing director and CIO for quantitative beta solutions at JPMAM, said one of the issues with using AI in finance is that a lot of the models are essentially black boxes. “A crucial part of understanding what a model is giving you is actually being able to look under the hood, so to speak,” he tells WatersTechnology. According to Romahi, this is where ThemeBot’s strengths come in. It first creates an initial seed query—for example, “gene,” “cell,” and/or “therapy.” That is enough for the AI engine to analyze articles and other data sources and create an initial portfolio.
Goldman Sachs
Goldman Sachs has been working with IBM to improve the process of pricing derivatives using quantum computing. William Zeng, head of quantum research at Goldman Sachs, told WatersTechnology there are three broad categories that suit quantum exploration in finance. First, there’s the field of simulation, which is where derivatives pricing falls, but there are also many risk calculations where the bank would like to better understand probabilistic behavior and how calculations can be modeled more accurately. The second category is optimization, such as portfolio optimization.
And in the third group there’s quantum machine learning. “The first two, we have a pretty clear handle in theoretical quantum computer science, at least on the first advantages, and we also have pretty concrete classical applications,” he says. “Machine learning is of interest, but it’s a little more nascent. … There has been some interesting heuristic work and motivating intuition, but quantum machine learning is a much younger field than optimization and simulation.”
Brown Brothers Harriman
Brown Brothers Harriman’s investor services unit—which was recently acquired by State Street—has delivered three large-scale tech platforms underpinned by various forms of artificial intelligence, and rolled out a fourth to its internal users in July. The most recently implemented project, Guardrail, was completed in 2020 and is part of an ongoing endeavor to radically transform its fund accounting process. It expanded upon one of BBH’s first AI projects in the division—the Anomaly NAV Tracking System (Ants), which was developed using supervised machine learning to help BBH’s analysts weed out thousands of false anomalies during end-of-day net-asset-value (NAV) strikes.
The Guardrail application monitors those NAV movements against a benchmark and historical performance and flags variances. It goes a step further than Ants does by identifying possible reasons for abnormal deviations, and brings those automated insights straight to BBH’s analysts through natural language generation—a rising priority for the bank, which is using the emerging tech to generate “smart alerts” that act as idea generation for research projects.
Deutsche Bank
Deutsche Bank Securities Services is looking to AI to improve its post-trade processes. The division currently uses AI in two ways. The first is in client segmentation, which allows DB Securities Services to improve and tailor its offering for clients. The second use case helps it predict settlement failures. First, the team drills down to individual clients’ requirements and determines what their future needs might be. Marcus Storm, head of AI products for product management at DB Securities Services, told WatersTechnology that this involves taking apart client activity.
“To know the clients and what they’re doing with their hundreds—sometimes thousands—of accounts, in any organization with this much data available per account, the ability for account managers to crunch the huge volume of data in their accounts is very low. So almost by definition, you don’t have a good overview of what the clients are doing and what their future needs might be,” he says. Machine learning algorithms allow the bank to automate that process. DB Securities Services uses classification-based algorithms, which classify or categorize data into distinct groups to reflect their similarities even if they are outside traditional client segments.
Acadian Asset Management
Acadian Asset Management, which runs $110 billion in assets, is training its natural language processing algorithms to root out so-called greenwashers. The AI tool screens public companies for green credentials—and then engages with firms that it suspects of failing to live up to their promises. The system sends automated emails to the companies in question to present them with its results and invite a response.
LSEG-Refinitiv
LSEG Labs—formerly Refinitiv Labs—is using machine learning for a new pre-trade market impact analysis tool for equity traders. The model will show the likely impact trades have on the market based on the stock for any particular volume size and time of day. LSEG Labs used the I-Star model—a standard market impact model that estimates the instantaneous trading cost for orders—as a baseline and then introduced a machine learning-based model to predict the market impact. The company used six months of historical tick data from the S&P 500 and Russell 1000 indexes, as well as data from other markets
BNP Paribas Asset Management
BNP Paribas Asset Management is using machine learning to estimate carbon emissions for companies that do not report their carbon footprint. The firm’s modeling of carbon emissions will provide estimates for some 10,000 companies. To model carbon emissions, the fund is using different types of machine learning, such as elastic net, XGBoost, and random forests.
“The model is built by finding the factors that can predict the emissions of companies that [have] already published data—this is our training dataset—which are then used to come up with carbon emissions estimates for the companies that currently do not publish their carbon emissions,” said Raul Leote de Carvalho, deputy head of the quant research group at BNP Paribas Asset Management. The models use factors such as the scale of operations of the company, which can predict emissions. For example, if the factor was company size and there were two oil companies with one producing significantly more oil, that company is likely to emit more carbon, even if it isn’t reported. The gaps in the data, as well as the lack of a standard approach to reporting carbon emissions data, make some in the industry cautious about the potential of machine learning at this stage.
Mizuho
Sam Clapp, Mizuho Americas equities, and Don Hundley, Japan head of Mizuho equities electronic trading, explain how the bank is using deep learning to more accurately predict equity market volumes. While AI can be viewed as more of a marketing buzzword in the electronic trading world, Mizuho’s AI research team is focused on applying cutting-edge science to the real-world conditions of the financial markets to develop algorithms that are smarter, more adaptable—and most importantly, that add value to trading operations.
PanAgora Asset Management
The $38 billion quant shop is using AI to assess how public companies are adhering to the United Nation’s Sustainable Development Goals (SDGs). The natural language processing algorithm can analyze upwards of 4,000 corporate sustainability reports to unearth if a firm’s actions meet the UN’s 17 objectives. The 17 goals—which are broken down into 169 targets—were adopted by the UN in 2015 with the aim of providing a blueprint for transforming society.
Ping An Asset Management
Ping An of China Asset Management (PAAMC) in Hong Kong—the asset management arm and a wholly-owned subsidiary of China’s largest insurer, Ping An Insurance (Group) Company of China—is looking to upgrade its natural language processing (NLP) models, particularly to account for Chinese sentiment analysis. The firm’s machine-learning framework combines deep-learning neural networks, gradient boosting machines, and advanced regression models. It also contains non-linear models, which help PAAMC capture factor interactions and non-linear patterns hidden in alpha signals.
On top of that, it provides low correlations among multiple models that can further increase Sharpe and information ratios—measurements that help investors determine the risk-adjusted returns of a security or portfolio. It uses historical structured and unstructured data—including news, price movement information, macroeconomic inputs, and company-specific accounting information—to train its AI algorithms. It monitors more than 300 factors and selects between 20 and 50 factors to construct its portfolios every month.
Finra
The Financial Industry Regulatory Authority plans to revamp its enterprise search capabilities using tools from Amazon Web Services to make it easier for its internal examinations staff and third parties, such as brokers and investors, to find information in its filings and reports using natural-language search to generate more relevant results. Finra’s goal is to build “regulatory connected intelligence by leveraging artificial intelligence and machine learning,” that will not only return lists of documents as search results, but will also provide links between entities in the results, a “factory” written summary of the results, and analytics that deliver personalized results based on a user and what organizations, individuals, stock symbols, or other criteria they are searching for.
Charles River
State Street’s Charles River Development is integrating its fixed-income order execution management system (OEMS) with start-up Wave Labs’ eLisa credit execution platform. The credit trading platform provides execution analysis, automated best execution documentation, and data on trading performance. The system uses a proprietary machine learning algorithm designed to study the behavior of the trader and provide recommended trading strategies, price settings, and smart choice trading protocols.
Symphony
Financial collaboration platform Symphony Communications Services is planning to leverage AI and data-sharing technology from Google, after confirming it will switch to the tech giant as its main cloud provider. Symphony initially decided to switch cloud providers after assessing its existing deal with Amazon Web Services (AWS) to be too expensive, Dietmar Fauser, chief information officer at Symphony, told WatersTechnology in July.
Six Group
Six is working on a prototype to mine data on US dividends. The tool reads and filters out press releases on dividends from news feeds Six consumes, and transforms the text messages into a structured record that can be processed electronically. If the prototype goes live—which could happen in 2022—customers will save time by not having to wait until the corresponding exchanges release dividend announcements.
APG Asset Management
The Dutch firm, which manages $690 billion of assets, is using decision tree-based machine learning to predict the movement of G10 currencies. “We are making predictions on currencies using market data, economic data, and fundamental data. For example, we are trying to predict if in a week or a month, how different currencies are likely to go up and down,” said portfolio manager Luiz Felix. Besides economic and market data, APG also uses predictions arrived at using nowcasting—predicting the present or the very near-term future—among the inputs into its machine learning model for forecasting future currency movements. The team uses principal component analysis (PCA) to streamline lots of daily economic data to use in nowcasting for different regions.
Creighton AI
San Francisco-based Creighton AI, which has just under $200 million in assets under management, specializes in using machine learning for systematic global equity long/short and long-only strategies. The firm’s data scientists are trying to predict excess returns of a stock relative to a market, but it is getting close to introducing a second form of machine learning at a different stage of the predictive process, as it wants better prediction accuracy. For that, it is looking at two different methodologies. One is a derivative of a support vector machine, a machine learning method that can offer an “optimal separation” between stocks expected to outperform the market on one side and stocks that should underperform on the other side. The fund is also exploring the possibility of adding a neural network to the prediction process.
Quoniam
The Frankfurt-based firm, which manages more than $30 billion of assets, is using machine learning to forecast returns where there are non-linear relationships between stock characteristics and the returns on these assets. Quoniam augments its linear predictive models with machine learning when forecasting returns. To model the relationships, the asset manager uses gradient boosted trees, a machine learning technique for optimizing the predictive value of a model using an ensemble of decision trees. The firm relies on open-source software for basic machine learning algorithms, though it does not use complete off-the-shelf solutions, as these tools don’t provide the firm with enough flexibility and transparency.
Only users who have a paid subscription or are part of a corporate subscription are able to print or copy content.
To access these options, along with all other subscription benefits, please contact info@waterstechnology.com or view our subscription options here: http://subscriptions.waterstechnology.com/subscribe
You are currently unable to print this content. Please contact info@waterstechnology.com to find out more.
You are currently unable to copy this content. Please contact info@waterstechnology.com to find out more.
Copyright Infopro Digital Limited. All rights reserved.
As outlined in our terms and conditions, https://www.infopro-digital.com/terms-and-conditions/subscriptions/ (point 2.4), printing is limited to a single copy.
If you would like to purchase additional rights please email info@waterstechnology.com
Copyright Infopro Digital Limited. All rights reserved.
You may share this content using our article tools. As outlined in our terms and conditions, https://www.infopro-digital.com/terms-and-conditions/subscriptions/ (clause 2.4), an Authorised User may only make one copy of the materials for their own personal use. You must also comply with the restrictions in clause 2.5.
If you would like to purchase additional rights please email info@waterstechnology.com
More on Emerging Technologies
This Week: Startup Skyfire launches payment network for AI agents; State Street; SteelEye and more
A summary of the latest financial technology news.
Waters Wavelength Podcast: Standard Chartered’s Brian O’Neill
Brian O’Neill from Standard Chartered joins the podcast to discuss cloud strategy, costs, and resiliency.
SS&C builds data mesh to unite acquired platforms
The vendor is using GenAI and APIs as part of the ongoing project.
Chevron’s absence leaves questions for elusive AI regulation in US
The US Supreme Court’s decision to overturn the Chevron deference presents unique considerations for potential AI rules.
Reading the bones: Citi, BNY, Morgan Stanley invest in AI, alt data, & private markets
Investment arms at large US banks are taken with emerging technologies such as generative AI, alternative and unstructured data, and private markets as they look to partner with, acquire, and invest in leading startups.
Startup helps buy-side firms retain ‘control’ over analytics
ExeQution Analytics provides a structured and flexible analytics framework based on the q programming language that can be integrated with kdb+ platforms.
The IMD Wrap: With Bloomberg’s headset app, you’ll never look at data the same way again
Max recently wrote about new developments being added to Bloomberg Pro for Vision. Today he gives a more personal perspective on the new technology.
LSEG unveils Workspace Teams, other products of Microsoft deal
The exchange revealed new developments in the ongoing Workspace/Teams collaboration as it works with Big Tech to improve trader workflows.