Machine Learning's Many Tentacles
Artificial intelligence is being applied in numerous functions used in financial services data management
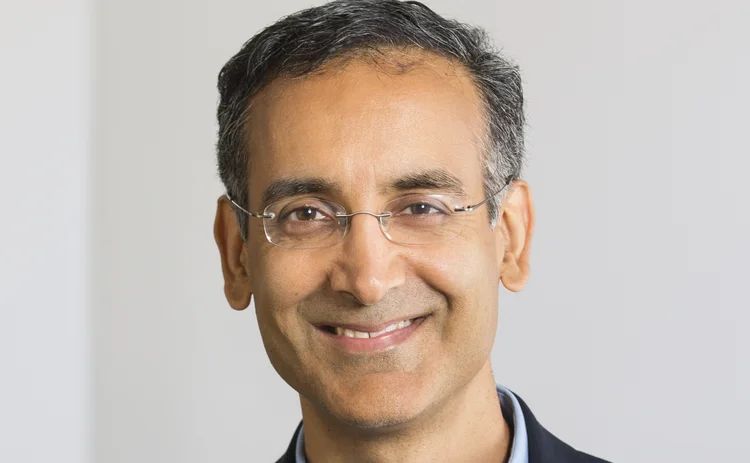
Machine learning, the application of artificial intelligence in computerized processes, has gained a foothold in data operations in the financial services industry.
The way machine learning is set up to analyze and work with data to yield insights, analysis and decision support is still developing, as executives from several service providers and machine-learning users tell Inside Reference Data. In particular, know-your-customer (KYC) applications for machine learning are emerging, along with already established uses.
The layers of decision-making that comprise portfolio management, starting with the origination of investment ideas on to the creation of trades, management of risk and execution of trades, can all be improved with machine learning, according to Henri Waelbroeck, director of research at Portware, a trade automation solutions provider owned by data services and software provider FactSet.
Of course, everything begins with the quality of the data fed into machine-learning systems, notes Waelbroeck. "Machine learning can help identify errors in the data by understanding statistical properties of the expected data, and identifying data points that are clearly violating those statistical properties and are therefore likely wrong," he says. "There are applications of data cleansing along those lines."
In this context, machine learning using the proper model can illustrate the impact of traders' actions on prices, and even produce an "impact-free price" that is a truer measure of the value of a security, Waelbroeck says.
Rules to direct the application of artificial intelligence can find and indicate data quality issues, explains Neil Vernon, chief technology officer at Gresham Computing, a UK-based provider of regulatory compliance, risk control and data integrity services, which deploys artificial intelligence (AI) in its products.
"AI can really automate exception management," he says. "For example, if three out of four tell you a figure is $1 million and the fourth says half a million, then it's probably $1 million. You can use AI to either completely solve this problem or at least guide people involved in the process toward the right solution. So far, our answer is to use AI to establish rule sets given to institutions and keep those rule sets as up to date as possible."
Artificial intelligence can keep a data integrity solution from "going stale," Vernon continues. "AI can determine whether the business rules and how the solution understands them are no longer the way the organization does business—and that it's time for those business rules to be updated," he says. "AI can suggest improvements to those business rules."
Although artificial intelligence can improve on the quality of data, Paul McInnis, head of enterprise data management at Eagle Investment Systems, cautions this must be approached carefully. "Organizations should have a clearly defined approach on where and how to apply this technology," he says. "There is a greater ROI when you're routinely applying it to large data sets. Over time, the technology will continue to be more sophisticated, which will allow for broader application across the enterprise."
Relationship Application
Aside from finding incorrect data, Gresham Computing applies machine learning and AI to group data and find relationships within it, according to Vernon. "This can be as simple as looking at a transaction in the front office, then the same one in the back office," he says. "There can be 50 different systems between the front office and the back office, so finding that relationship can be very difficult. We use machine learning to figure out that a stream of data and the transactions in it have a relationship with the transactions in another stream."
Using data management and data integrity solutions to accomplish this same task takes much longer if done manually, Vernon observes. "Organizations stopped using enterprise data management stores because they were too difficult to configure," he says. "The tool they reach for is Excel because it's the easiest to enter data [into], but they're subject to all the vagaries of Excel and all the problems that can occur."
Applying natural language to machine learning, however, can turn contracts "into a set of data and executables," says David Saul, senior vice-president and chief scientist at State Street, who works with data semantics issues. "Contracts... have a limited vocabulary and limited syntax. Lawyers reuse the same terms over and over again. The variability is in the data; who are the parties and [in the case of interest-rate swaps] what defines interest rates—fixed or floating."
Still, machine learning must be directed at producing a defined outcome, as Roy Singh, chief technology officer at AltX, a machine-learning services provider, explains: "The companies and projects that are successful actually link specifically to an outcome. It comes down to whether we helped a pension fund make a good or a bad investment decision. When they were making the decision, they either had data points that informed them and an insight they wouldn't have had before or [they] were alerted to something relevant in a fund where they were already invested.
"We drive to apply machine learning at the point where they have an important decision and outcome, as opposed to just giving them information that is interesting," adds Singh.
To this end, more companies are using machine learning and artificial intelligence to manage data, according to McInnis of Eagle Investment Systems. "For overall enterprise data management strategy, firms that are not already thinking about machine learning need to start," he says. "It has an impact on the overall data management strategy, what data sets they use and how they support that operationally as an organization."
Structuring Data
AltX's user base began with hedge funds, but has since widened to other types of investment firms. Pension funds and investors who invest with hedge funds demand insights that require detailed analysis of multiple data sets, including regulatory data, quantitative financial performance data, news, legal data and more, says Singh.
"Even though it is messy and conflicting, we need to turn it into something that is actually coherent and sane for our customers to search, interrogate and screen in an easy way," he explains. "We also need to derive insight from that and synthesize it into information that matters to them."
As Google uses artificial intelligence to power the ‘auto-complete' feature of its search bar, AltX uses machine learning to perform tasks such as organizing unstructured regulatory data acquired in HTML format to produce a data set that is structured. AltX can then match that data set with entities in a knowledge graph containing representations of all relevant companies in the hedge fund industry, along with the relationships between them and the funds they manage, as well as the personnel of those companies, says Singh. This requires language-processing capabilities besides machine learning.
After these steps, the foundation is set to then predict funds' success or failure, whether assets will trend up or down, and what the returns will be, according to Singh.
KYC and Identification Uses
The ability to map "smart contracts," as State Street's Saul describes those that can be mapped in machine-executable form, also makes it possible to automate compliance functions and automatically verify compliance. This provides another type of regulatory data, along with insight into corporate relationships—one focus of AltX's offerings.
Linking data, which Gresham Computing and AltX use machine learning to do, in different ways, is especially useful for KYC applications and identification.
"With KYC, the biggest obstacle we hear [of] is being able to link data sets together around the customer and build up a single, integrated view, so the banks and wealth managers really have a good understanding—a 360-degree view of the customer," says Singh. "You can link these data sets together via a multi-year warehousing initiative, where you manually map the data silos against each other. We focus on the application of machine learning to link data sets together, so a single picture of the customer can be presented."
State Street looks at legal entity identifier (LEI) data in a KYC context and applies machine learning to clean it up, says Saul. "We found cases where we duplicated the same legal entity, because two different people had done it in two different applications and put different addresses. The tool we use identifies those as potential duplicates. I'm not saying a human being couldn't have found these, but some had been sitting around a very long time."
Saul emphasizes that while this is a simple, small task that can be accomplished with machine learning, it is still an important one. David Blaszkowsky, a former colleague of Saul's at State Street, echoes this sentiment, saying precision and accuracy, as benefits of artificial intelligence, carry more importance than grand projects, especially when applied to financial industry operations.
"Big data is very exciting and wonderful for building it into the artificial intelligence and machine learning to get smarter," he says. "It still comes back down to dealing within the financial system, where there isn't unlimited data. Precision is important, not approximation. The better your data is, the better governed it is, the more precise and defined it is, [and] the better you will be able to use it to make smarter and better decisions, and create applications that will learn better."
Only users who have a paid subscription or are part of a corporate subscription are able to print or copy content.
To access these options, along with all other subscription benefits, please contact info@waterstechnology.com or view our subscription options here: http://subscriptions.waterstechnology.com/subscribe
You are currently unable to print this content. Please contact info@waterstechnology.com to find out more.
You are currently unable to copy this content. Please contact info@waterstechnology.com to find out more.
Copyright Infopro Digital Limited. All rights reserved.
As outlined in our terms and conditions, https://www.infopro-digital.com/terms-and-conditions/subscriptions/ (point 2.4), printing is limited to a single copy.
If you would like to purchase additional rights please email info@waterstechnology.com
Copyright Infopro Digital Limited. All rights reserved.
You may share this content using our article tools. As outlined in our terms and conditions, https://www.infopro-digital.com/terms-and-conditions/subscriptions/ (clause 2.4), an Authorised User may only make one copy of the materials for their own personal use. You must also comply with the restrictions in clause 2.5.
If you would like to purchase additional rights please email info@waterstechnology.com
More on Data Management
New working group to create open framework for managing rising market data costs
Substantive Research is putting together a working group of market data-consuming firms with the aim of crafting quantitative metrics for market data cost avoidance.
Off-channel messaging (and regulators) still a massive headache for banks
Waters Wrap: Anthony wonders why US regulators are waging a war using fines, while European regulators have chosen a less draconian path.
Back to basics: Data management woes continue for the buy side
Data management platform Fencore helps investment managers resolve symptoms of not having a central data layer.
‘Feature, not a bug’: Bloomberg makes the case for Figi
Bloomberg created the Figi identifier, but ceded all its rights to the Object Management Group 10 years ago. Here, Bloomberg’s Richard Robinson and Steve Meizanis write to dispel what they believe to be misconceptions about Figi and the FDTA.
SS&C builds data mesh to unite acquired platforms
The vendor is using GenAI and APIs as part of the ongoing project.
Aussie asset managers struggle to meet ‘bank-like’ collateral, margin obligations
New margin and collateral requirements imposed by UMR and its regulator, Apra, are forcing buy-side firms to find tools to help.
Where have all the exchange platform providers gone?
The IMD Wrap: Running an exchange is a profitable business. The margins on market data sales alone can be staggering. And since every exchange needs a reliable and efficient exchange technology stack, Max asks why more vendors aren’t diving into this space.
Reading the bones: Citi, BNY, Morgan Stanley invest in AI, alt data, & private markets
Investment arms at large US banks are taken with emerging technologies such as generative AI, alternative and unstructured data, and private markets as they look to partner with, acquire, and invest in leading startups.