Mapping a successful data journey: strategy, execution and sustainability
A well-planned data journey can positively impact an organization’s long-term trajectory. However, it is important to have clarity not only in the strategy but also in successful execution and sustainability for the long haul, argues data veteran Subbiah Subramanian.
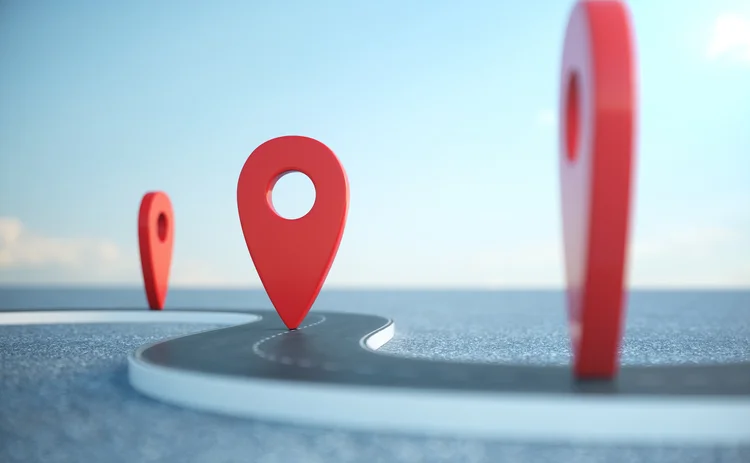
An organization must have a clear and coherent enterprise data strategy to achieve its goals. In the financial sector, different business areas have diverse data requirements and preferences, depending on their level of development, scale, market presence, and regulatory compliance.
For instance, an investment management firm may have different data priorities for its core business area that handles traditional assets (such as equity, fixed income, and derivatives), its alternative asset management team that deals with non-standardized datasets (such as real estate and private equity), and its outsourced chief investment office (OCIO) business that strives to offer maximum transparency to its clients.
An OCIO helps large pension plans and employers to manage money without needing to set up their own investment management offices. These business areas may also have different criteria for measuring success, such as revenue growth, client satisfaction, product innovation, or regulatory adherence.
Given this diversity, it may seem easier for each division or business unit to address their data needs separately. However, this would only exacerbate the data silos that hamper today’s data infrastructure. Furthermore, this would result in costly and inefficient solutions that benefit only a fraction of the organization. For example, a large pension fund client’s portfolio may consist of a wide range of assets, including traditional and alternative investments.
A vital piece of the puzzle is to measure the progress of the data strategy continuously, but even more importantly, to communicate that progress to business stakeholders
Without a holistic enterprise data strategy, the organization may end up investing in capabilities that are not shared or leveraged across different areas. A typical use case is building a data lake to support traditional assets, while leaving alternative data in outdated technology. This would create inconsistency and disparity in the client’s interaction with different data domains.
Therefore, an enterprise-wide data strategy is essential in the current scenario. I have witnessed many enterprise data strategy initiatives that started with high expectations and enthusiasm but failed to deliver in the long run. This article aims to highlight the key factors that contribute to a successful data strategy implementation.
There are many facets to a data journey. The following are my top three.
Anchor data strategy to business goals and directly engage business teams
The output of the data strategy should be essential for achieving business success, as most business units have clear and long-term business goals. These goals usually include increasing revenue by a certain amount, expanding their customer base with new products, enhancing customer satisfaction with better service levels, and complying with regulatory requirements.
For instance, if the business goal is to launch a new mutual fund investment product in Europe, the data strategy should provide an operating model that supports European time zones and reporting tools that meet enhanced regulatory reports. This way, the data strategy becomes a “must-have” rather than a “nice-to-have” for the business, and also motivates business partners to be invested in the data strategy.
Another key component of data strategy is to adopt data governance best practices by using tools and procedures, such as data quality measurement, data lineage and catalog, as part of the overall implementation. To continue with the example of launching a new mutual fund in Europe, data quality and timeliness checks of all data sources should be recorded and reported at the time of data capture. The data operations team should have access to these results, so that validating data is part of their daily workflow.
This method ensures that the quality of data used to build the new mutual fund is not left to chance. Data lineage that is fundamental to mutual fund trading should be tracked and cataloged in a enterprise tool. This tool will be useful for managing and communicating with clients and regulators. By making data governance part of the overall “new mutual fund project,” it will have a higher chance of success than as a separate implementation. This also makes the data governance initiative fit naturally into the element.
A vital piece of the puzzle is to measure the progress of the data strategy continuously, but even more importantly, to communicate that progress to business stakeholders, who will want to know what’s in it for them. The measurements should be easy to understand and ongoing. Common measurements include service-level agreement (SLA) levels, error rates, and time to introduce new product, among others. These statistics must be converted into business benefits and communicated clearly and regularly to business partners.
This step is often overlooked by data programs. To go back to mutual fund example, data availability for start of trading, new security set up times, error rate with Index data etc. should be tracked and communicated on a daily basis. This will give business leaders visibility into the benefits of overall data strategy.
‘Best execution’: Central governance with federated responsibility
A data strategy should be aligned with the business teams, but not centralized in its execution. Different business areas have the expertise and knowledge of data needed to carry out a data strategy effectively. Organizations have tried to centralize data teams by moving people from different areas, but this usually leads to a loss of synergy and communication with the business users.
The industry solution to this problem is to federate the implementation to the relevant business teams and oversee it centrally. This means that the data program sets and monitors the data management methodology, roadmap, process, and tools that are agreed upon by all stakeholders.
However, the execution of this process should be done by the individual teams. This allows the teams to work on their priorities from the roadmap and involve the right business users.
It is often said that data should be a key tool for decision-making. This does not mean that only senior management should use data
For example, if the new European mutual fund has equity and fixed-income securities, the equity and fixed-income security reference, indices, and so on should be handled by the respective business and technology teams, as they are the experts on these datasets. However, these teams should use the same tools for data processing, data quality checks, lineage cataloging, etc. This ensures a consistent data governance and technology architecture, but a distributed implementation across the organization.
Sustainability: Aligning data culture and operating model
Transforming an organization into a data-driven one is not a short-term project but a long-term journey that takes several years. Therefore, it is essential to establish the operating model and cultivate a data culture that can last for a long time. Data culture means the shared values and practices of people in an organization for using data to enhance business performance.
To nurture this culture, data must be made available to the users with simplified processes to access it. When designing a data roadmap, it is important to focus on initiatives that have a big impact on employees’ daily work life. It is often said that data should be a key tool for decision-making. This does not mean that only senior management should use data.
To create a data culture, data should improve the work experience of every employee. A real-life situation at a financial institution involved core processes running on mainframe platforms. The mainframe reports did not show key data and accessing the data directly slowed down the platform.
The operations team struggled to support the platform without the data. As a part of the data strategy, data from the mainframe was transferred to a cloud-based database with appropriate governance and access control. This allowed the analysts to access the data systematically. The operations team took it upon themselves to learn to use a cloud-based reporting tool, and improved their efficiency. The data strategy team gained a loyal ally in the organization.
Establishing a clear operating model is critical to the success of a firm’s data journey. Collecting, defining, prioritizing, and funding data initiatives across the organization is fundamental. Setting up a robust process to develop a roadmap that captures requirements across the organization is key to sustaining the success of data strategy programs. A well-developed roadmap becomes a great tool to prioritize initiatives that have the most business value and provide leadership transparency into the backlog.
To sum up, a good data strategy is one that aligns with the overall business strategy. This alignment ensures that the business teams are fully committed to the data strategy’s success. Another key to success is to maintain the data strategy for a long time, as data maturity is not a quick fix but a continuous process. Last but not least, data strategy should have a direct and positive impact on the work life of fellow employees.
Subbiah Subramanian has held senior data roles at the Depository Trust & Clearing Corp. (DTCC), State Street, and BNY Mellon.
Only users who have a paid subscription or are part of a corporate subscription are able to print or copy content.
To access these options, along with all other subscription benefits, please contact info@waterstechnology.com or view our subscription options here: http://subscriptions.waterstechnology.com/subscribe
You are currently unable to print this content. Please contact info@waterstechnology.com to find out more.
You are currently unable to copy this content. Please contact info@waterstechnology.com to find out more.
Copyright Infopro Digital Limited. All rights reserved.
As outlined in our terms and conditions, https://www.infopro-digital.com/terms-and-conditions/subscriptions/ (point 2.4), printing is limited to a single copy.
If you would like to purchase additional rights please email info@waterstechnology.com
Copyright Infopro Digital Limited. All rights reserved.
You may share this content using our article tools. As outlined in our terms and conditions, https://www.infopro-digital.com/terms-and-conditions/subscriptions/ (clause 2.4), an Authorised User may only make one copy of the materials for their own personal use. You must also comply with the restrictions in clause 2.5.
If you would like to purchase additional rights please email info@waterstechnology.com
More on Data Management
New working group to create open framework for managing rising market data costs
Substantive Research is putting together a working group of market data-consuming firms with the aim of crafting quantitative metrics for market data cost avoidance.
Off-channel messaging (and regulators) still a massive headache for banks
Waters Wrap: Anthony wonders why US regulators are waging a war using fines, while European regulators have chosen a less draconian path.
Back to basics: Data management woes continue for the buy side
Data management platform Fencore helps investment managers resolve symptoms of not having a central data layer.
‘Feature, not a bug’: Bloomberg makes the case for Figi
Bloomberg created the Figi identifier, but ceded all its rights to the Object Management Group 10 years ago. Here, Bloomberg’s Richard Robinson and Steve Meizanis write to dispel what they believe to be misconceptions about Figi and the FDTA.
SS&C builds data mesh to unite acquired platforms
The vendor is using GenAI and APIs as part of the ongoing project.
Aussie asset managers struggle to meet ‘bank-like’ collateral, margin obligations
New margin and collateral requirements imposed by UMR and its regulator, Apra, are forcing buy-side firms to find tools to help.
Where have all the exchange platform providers gone?
The IMD Wrap: Running an exchange is a profitable business. The margins on market data sales alone can be staggering. And since every exchange needs a reliable and efficient exchange technology stack, Max asks why more vendors aren’t diving into this space.
Reading the bones: Citi, BNY, Morgan Stanley invest in AI, alt data, & private markets
Investment arms at large US banks are taken with emerging technologies such as generative AI, alternative and unstructured data, and private markets as they look to partner with, acquire, and invest in leading startups.