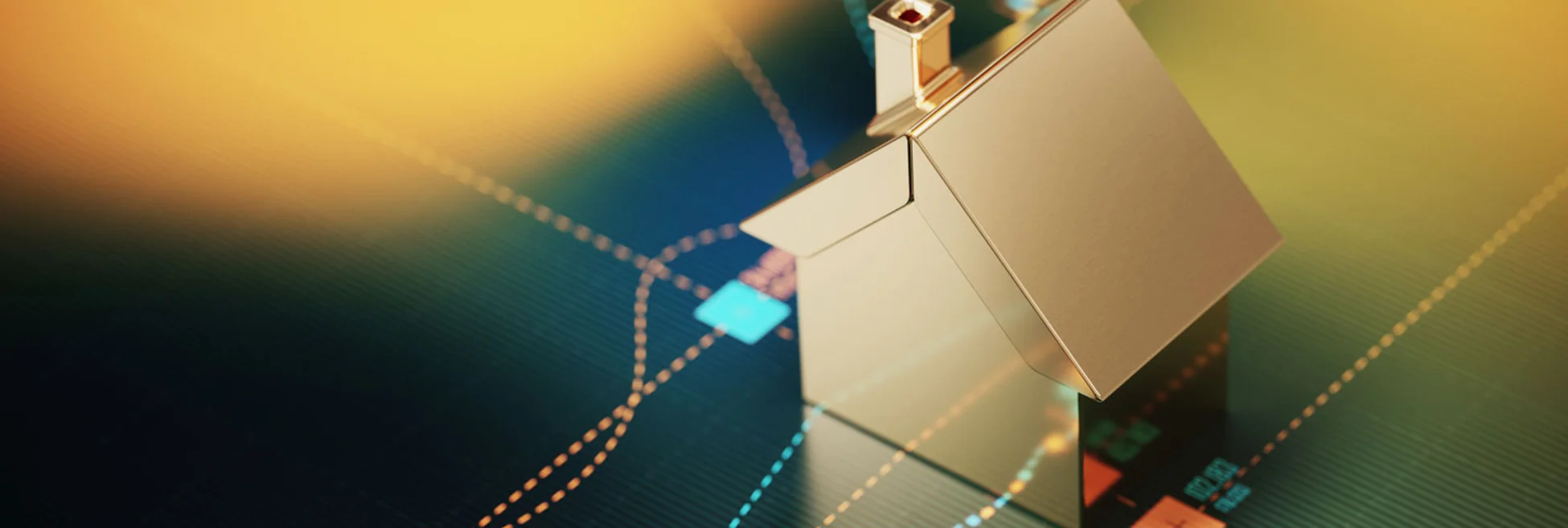
Messy MBSs? Startup uses deep learning to predict mortgage-backed securities markets
As interest rates rise and house prices fall after a steady period of the opposite dynamic, investors are looking for more accurate ways to price these factors into the value of mortgage-backed securities.
While the globe bakes in record high temperatures, there’s one thing cooling down: the US housing market, where inflation and rising interest rates have led to a distinctly chillier climate.
In November, housing price data provider Zillow predicted write-downs of more than half a billion dollars on mortgage-backed securities (MBSs) it issued as a result of winding down its Zillow Offers house-flipping business, which overpaid for homes it expected to resell for a profit, at the same time that the housing market began to cool. Zillow’s shares took a 40% plunge last November as a result and have continued to decline since then.
If an acknowledged specialist data provider has trouble pricing market fluctuations, how are other industry participants expected to succeed? A startup, spun out of a project at Stanford University, claims its approach delivers a 10x improvement in the accuracy of predictions around MBS valuations and changes. The vendor, Infima, already has more than 30 bulge-bracket sell-side and buy-side firms testing its system, and just raised $5 million in seed funding from Radical Ventures, an AI-focused private equity firm, along with other investors including Franklin Templeton and ThirdStream Partners.
“We harness data of unprecedented size and granularity—billions of single data points—on an enormous data cloud,” which utilizes both Amazon Web Services (AWS) and Microsoft Azure, says Infima chairman and chief scientist Kay Giesecke.
“It allows us to explore how borrowers and homeowners behave, beyond just looking at old measures like Fico scores. And if you combine that data with this non-parametric deep learning, then we get to the point where we can see hidden patterns in borrower behavior that traditional approaches miss,” Giesecke says.
This is important because since borrowers are people, each person is affected differently by macroeconomic changes, says Giesecke, who set up the company in 2020 while serving as a professor and director of the Advanced Financial Technologies Laboratory at Stanford University’s School of Engineering.
“There are patterns of behavior that you can identify around that, which allows us to make very accurate predictions of how people will behave in the future,” he says. “So, we can see, for example, if someone is falling behind in their payments, or coming back out of delinquency, or making pre-payments, or whether the loan has been modified.”
The model uses traditional metrics such as loan-level data and macroeconomic factors, but combines them with deep analysis of more than 20 years of historical data on borrower behavior, collected on tens of millions of individual borrowers in the US on a monthly basis. Infima’s platform makes predictions for each mortgage holder, not just at the MBS level, then presents the data at the security level while also allowing users to drill into the underlying data.
“If you take an MBS, it’s a bond with a coupon, and it’s backed by a certain number of individual mortgages—which could be just a handful or could be hundreds of thousands. So we show information on the security and on the people backing it. Then we predict how the security will behave on a monthly basis in terms of pre-payments until the maturity date, which could be up to 30 years,” Giesecke says.
The result, he says, is a 10x improvement in predictive performance compared to widely used existing models. He says this has been “particularly pronounced” during the pandemic and during recent changes in interest rates.
“For a portfolio manager or trader, that 10x improvement means that if you have more accurate insights into how securities and markets behave, you’ll be able to identify more deals, avoid more bad deals, and gain more clarity around what is a good or bad deal,” he says. “So, it can be used to make decisions around portfolio construction, security selection, and hedging with greater accuracy.”
Franklin Templeton, which originally invested in the startup in 2020 as part of its Silicon Valley fintech incubator program, is one of the early adopters of the technology.
“We are currently engaged with Infima on multiple strategic levels including as an investor and early user of their predictive deep learning platform,” says Margaret King, vice president of fintech partnership and corporate strategic investments at Franklin Templeton. While declining to give specific details of what results the firm has achieved by using Infima’s data, she says the firm is “encouraged by the early results and remains actively engaged with Infima.”
Though the vendor believes its technology can be applied broadly across all areas of fixed income, Giesecke decided to focus initially on the MBS market for three main reasons: the size of the market, the amount and richness of data available, and his opinion that it had been under-served by tools currently available.
“I’ve been in fixed-income markets for the past 20 years, and mortgages—right up there next to corporate bonds—is one of the biggest verticals. Every fixed-income investor has an allocation to mortgages alongside other areas such as government debt, so it’s an area of interest to every fixed-income investor,” he says.
Next stages of growth
The vendor can supply the data via APIs that can integrate with firms’ existing in-house systems, such as pricing or risk systems, but also offers a web-based interface that provides access to all its data and allows users to search for specific securities by Cusip number. In addition, it supports exporting the data for use in spreadsheets, Python, or Jupyter Notebooks.
Aside from predictive data, the vendor also provides prescriptive tools. So, for example, it can make decision recommendations based on the platform’s predictions and identify a sector of the market that appears attractive.
These predictive capabilities are what attracted Franklin Templeton as a client and investor.
“The key advantage of Infima’s deep learning model (vs. conventional models) is predictive accuracy,” King says. “This comes mainly from the deep learning model’s ability to capture the significant non-linear effects tied to mortgage borrowers’ behaviors. Combining the deep learning system with computational algorithms, Infima is able to deliver the predictions at unprecedented speeds, including at intra-day frequencies.”
The company was initially staffed by former students who worked with Giesecke at Stanford, though the company has more recently made hires in client solutions, sales, marketing, and product management, bringing it to 15 employees in total. Also, in conjunction with its new funding, the company has appointed Hendrik Bartel CEO, taking over the role from Giesecke. Bartel was most recently senior vice president of ESG strategy at FactSet Research Systems, which he joined via its purchase of ESG data provider Truvalue Labs, where he was co-founder and CEO.
The funding will allow Infima to continue to make new hires as it moves from stealth mode to openly targeting more sales to financial institutions, as well as to build out support for other verticals within fixed income, Bartel says, adding that the vendor is also getting requests to add support for climate-based and ESG securities, and other areas, such as the mortgage originators, who would find the data useful to identify potential borrowers who might want to refinance, or to serve as the basis for lending decisions.
Audrey Blater, senior analyst covering risk and financial markets regulation at research firm Coalition Greenwich, says the approach has obvious applicability in other areas of fixed income, such as more liquid, investment-grade securities that also have rich datasets available, but could also benefit investors analyzing high-yield securities issued by companies that may be less well known, or privately held companies—subject to identifying the right underlying datasets.
“For MBSs, that’s individual data on borrowers … whereas in the corporate bond world, you’re relying on analyst ratings. There’s a lot of macroeconomic data that goes into that, but each sector has its own nuances, with sector-specific factors,” Blater says.
Giesecke says that to serve other verticals, such as municipal and corporate bonds, Infima will apply the same data-agnostic template it developed for the mortgage space but will use datasets specific to each asset class.
“Take, for example, corporate bonds, which many of our clients and prospective clients have highlighted as another important area of interest besides mortgages. Here we’ll predict credit rating transitions (e.g., AA to A) and the associated changes in credit spreads at the individual bond level. While based on a different dataset, this is structurally similar to predicting mortgage borrower state transitions (e.g., from current to prepayment), allowing us to re-use our framework in this new vertical,” Giesecke says, adding that the vendor has already tested this use case.
Further reading
Only users who have a paid subscription or are part of a corporate subscription are able to print or copy content.
To access these options, along with all other subscription benefits, please contact info@waterstechnology.com or view our subscription options here: http://subscriptions.waterstechnology.com/subscribe
You are currently unable to print this content. Please contact info@waterstechnology.com to find out more.
You are currently unable to copy this content. Please contact info@waterstechnology.com to find out more.
Copyright Infopro Digital Limited. All rights reserved.
As outlined in our terms and conditions, https://www.infopro-digital.com/terms-and-conditions/subscriptions/ (point 2.4), printing is limited to a single copy.
If you would like to purchase additional rights please email info@waterstechnology.com
Copyright Infopro Digital Limited. All rights reserved.
You may share this content using our article tools. As outlined in our terms and conditions, https://www.infopro-digital.com/terms-and-conditions/subscriptions/ (clause 2.4), an Authorised User may only make one copy of the materials for their own personal use. You must also comply with the restrictions in clause 2.5.
If you would like to purchase additional rights please email info@waterstechnology.com
More on Data Management
New working group to create open framework for managing rising market data costs
Substantive Research is putting together a working group of market data-consuming firms with the aim of crafting quantitative metrics for market data cost avoidance.
Off-channel messaging (and regulators) still a massive headache for banks
Waters Wrap: Anthony wonders why US regulators are waging a war using fines, while European regulators have chosen a less draconian path.
Back to basics: Data management woes continue for the buy side
Data management platform Fencore helps investment managers resolve symptoms of not having a central data layer.
‘Feature, not a bug’: Bloomberg makes the case for Figi
Bloomberg created the Figi identifier, but ceded all its rights to the Object Management Group 10 years ago. Here, Bloomberg’s Richard Robinson and Steve Meizanis write to dispel what they believe to be misconceptions about Figi and the FDTA.
SS&C builds data mesh to unite acquired platforms
The vendor is using GenAI and APIs as part of the ongoing project.
Aussie asset managers struggle to meet ‘bank-like’ collateral, margin obligations
New margin and collateral requirements imposed by UMR and its regulator, Apra, are forcing buy-side firms to find tools to help.
Where have all the exchange platform providers gone?
The IMD Wrap: Running an exchange is a profitable business. The margins on market data sales alone can be staggering. And since every exchange needs a reliable and efficient exchange technology stack, Max asks why more vendors aren’t diving into this space.
Reading the bones: Citi, BNY, Morgan Stanley invest in AI, alt data, & private markets
Investment arms at large US banks are taken with emerging technologies such as generative AI, alternative and unstructured data, and private markets as they look to partner with, acquire, and invest in leading startups.