Morningstar Chronicles AI Quest to Replicate Analyst Ratings
With the fund industry growing at exponential rates, Morningstar turned to disruptive technologies to keep pace and ensure it could deliver sufficient coverage without compromising the processes used by its human analysts. Max Bowie chronicles the five-year project that led to the vendor’s new AI-based Quantitative Rating.
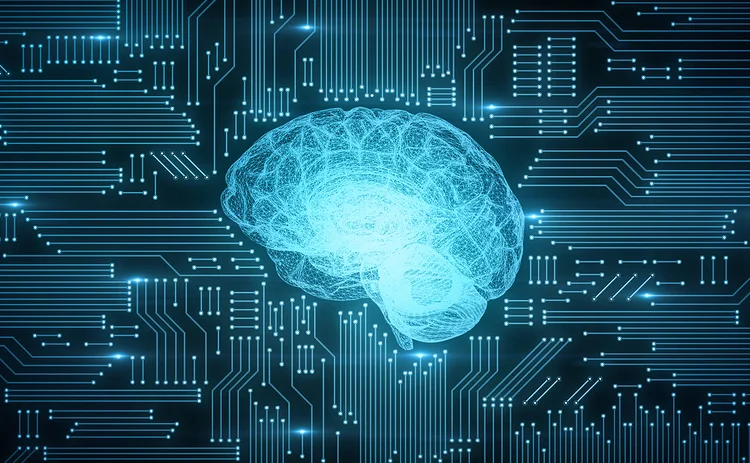
The new Morningstar Quantitative Rating—designated on Morningstar’s websites, data services and workstations by a “Q” beside the rating—allows the vendor to rate more than 10,000 open-end and exchange-traded funds, six times more than the 1,800 currently covered by its analysts in the US. This significantly expands the ability of investors, advisors, wealth managers and researchers to use Morningstar’s ratings for fund selection and to provide forward-looking insight that supports their investment decisions. The Quantitative Rating will only be used to rate funds not already covered by Morningstar analysts.
The rating was originally conceived in response to the continuing growth of the mutual fund industry and consumer demand for greater coverage. Just after launching its Analyst Rating for Funds, Morningstar realized that its “rigorous and intensive” manual approach could not be scaled in line with the growth in coverage required, and embarked on a project to replicate its analysts work using artificial intelligence.
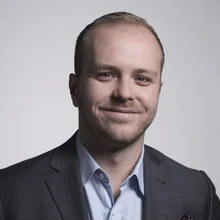
“So we were asking how we address that gap… and AI was one of the things we looked at. We had a hypothesis that it would be possible because we have a history of training new analysts to think the way we think, and to apply our rating to new funds—so it should be a process that’s repeatable,” says Lee Davidson, head of quantitative research at Morningstar, adding that the vendor judges the success of the rating not based on its ability to accurately predict returns, but rather its ability to replicate what an analyst would do.
Specifically, when a Morningstar analyst rates any fund, they must consider five key elements—process, people, price, parent, and performance—and produce expectations for each of these five areas before delivering a final overall rating that corresponds with the vendor’s existing Analyst Rating scale: Gold, Silver, Bronze, Neutral, and Negative.
When it comes to the data used as an input to the ratings, Morningstar also replicated the research process used by its analysts. “When it comes to data, we had a pretty good idea of what our people looked at, so we pulled in around 150 data points that our analysts had used in the past, then whittled those down to a select number of the most important decision points,” Davidson says.
The machine-learning aspect of the rating also allowed it to make its own decisions about what data to use, where appropriate. “Most modeling processes struggle with ‘conditions’—i.e., if A, B, C and D occur, then do E. AI is pretty good about figuring these out, even if the inputs and conditions are not explicitly made clear,” he adds.
Developing the rating was a challenging process that involved a lot of trial and error, Davidson says, adding that it wasn’t a foregone conclusion that it would ever see the light of day. “We would probably have pulled the plug if we hadn’t liked where it was going—that’s the sign of a good R&D department.”
The initial build took several months, then his team developed new iterations every few weeks, but it was still some two years before internal stakeholders felt it was worth testing. From that point, Morningstar has been running the rating internally, constantly vetting and refining it before eventually rolling it out in a “limited release” on the Morningstar Direct service in June last year, ahead of a full rollout on the vendor’s other data services by the end of March.
During the lengthy vetting and refinement period, Morningstar was constantly evaluating the rating against the “three pillars” of performance, stability, and accuracy to ensure it was accurately replicating how analysts would make decisions, and did not fluctuate from one rating in one month to the opposite position the next month for the same fund.
Ironically, though Morningstar developed the rating to deliver coverage far beyond the capacity of its analysts, Davidson says human expertise was the key component in building it. “There are many open-source and publicly available tools available to do this, such as Python, R, and SQL… but you have to know how to use them. The human capital is the most important piece,” he says.
Only users who have a paid subscription or are part of a corporate subscription are able to print or copy content.
To access these options, along with all other subscription benefits, please contact info@waterstechnology.com or view our subscription options here: http://subscriptions.waterstechnology.com/subscribe
You are currently unable to print this content. Please contact info@waterstechnology.com to find out more.
You are currently unable to copy this content. Please contact info@waterstechnology.com to find out more.
Copyright Infopro Digital Limited. All rights reserved.
As outlined in our terms and conditions, https://www.infopro-digital.com/terms-and-conditions/subscriptions/ (point 2.4), printing is limited to a single copy.
If you would like to purchase additional rights please email info@waterstechnology.com
Copyright Infopro Digital Limited. All rights reserved.
You may share this content using our article tools. As outlined in our terms and conditions, https://www.infopro-digital.com/terms-and-conditions/subscriptions/ (clause 2.4), an Authorised User may only make one copy of the materials for their own personal use. You must also comply with the restrictions in clause 2.5.
If you would like to purchase additional rights please email info@waterstechnology.com
More on Data Management
New working group to create open framework for managing rising market data costs
Substantive Research is putting together a working group of market data-consuming firms with the aim of crafting quantitative metrics for market data cost avoidance.
Off-channel messaging (and regulators) still a massive headache for banks
Waters Wrap: Anthony wonders why US regulators are waging a war using fines, while European regulators have chosen a less draconian path.
Back to basics: Data management woes continue for the buy side
Data management platform Fencore helps investment managers resolve symptoms of not having a central data layer.
‘Feature, not a bug’: Bloomberg makes the case for Figi
Bloomberg created the Figi identifier, but ceded all its rights to the Object Management Group 10 years ago. Here, Bloomberg’s Richard Robinson and Steve Meizanis write to dispel what they believe to be misconceptions about Figi and the FDTA.
SS&C builds data mesh to unite acquired platforms
The vendor is using GenAI and APIs as part of the ongoing project.
Aussie asset managers struggle to meet ‘bank-like’ collateral, margin obligations
New margin and collateral requirements imposed by UMR and its regulator, Apra, are forcing buy-side firms to find tools to help.
Where have all the exchange platform providers gone?
The IMD Wrap: Running an exchange is a profitable business. The margins on market data sales alone can be staggering. And since every exchange needs a reliable and efficient exchange technology stack, Max asks why more vendors aren’t diving into this space.
Reading the bones: Citi, BNY, Morgan Stanley invest in AI, alt data, & private markets
Investment arms at large US banks are taken with emerging technologies such as generative AI, alternative and unstructured data, and private markets as they look to partner with, acquire, and invest in leading startups.