On Democracy and Alt Data's Democratization: Preparing For the US Election
Advancements in modeling and the rise of alt data have made the process of prepping for the US presidential election more complex, but hopefully more accurate.
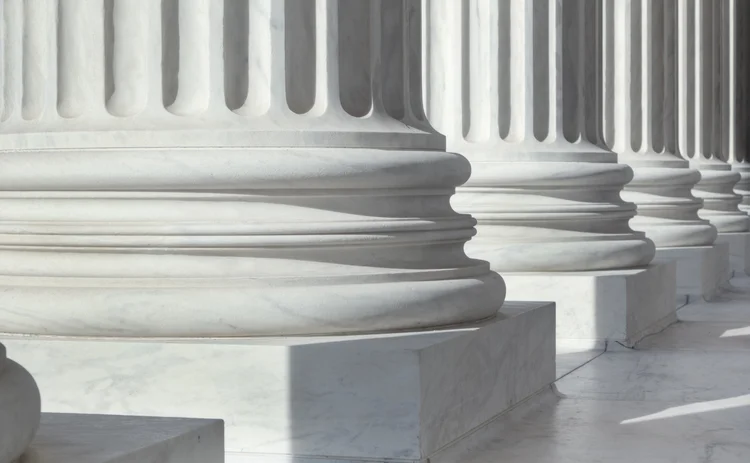
In hindsight, it seems easy now to dissect all the ways in which key election polls were wrong in the lead up to the US presidential race between Donald Trump and Hillary Clinton in 2016. Hindsight is 20/20, though, and this year’s election has only been further complicated due to the pandemic. As Tuesday, November 3, rapidly approaches, data providers and their clients are arming themselves with more sources of information and—hopefully—better forecasting models.
As alternative data and predictive-AI models become democratized—that is, no longer confined to the realm of only the most-sophisticated quant funds with the deepest pockets—a more diverse set of asset managers are looking to get ahead of the election curve using these new offerings. Four years ago, a small cluster of funds used the data they had available—vastly less than what can be scrounged up today—to pick a winner and loser, which, of course, made winners and losers out of the funds, depending on their bets.
Today, executives say, the preparation process is much less about the names of the victors this November; it’s about planning for the several different scenarios that can play out after Election Night, and trying to mitigate the associated risks that will result from those outcomes.
“Our user base is thinking much broader and differently than four years ago,” says Rado Lipuš, founder and CEO of Neudata, which seeks out alpha-generating datasets for buy-side firms. “Back then, it was mainly the quant funds who were using alternative data—very few discretionary. Today it’s probably half and half. … The market has just matured in the discretionary space so much more that there are many more specific use-cases around elections, and the implications that brings with it.”
In the last decade, the spread of information has colored nearly every corner of American life, and hedge funds are eager to tap into this potentially valuable information to derive unique insights. Today, there are more than 1,486 alternative data providers and 5,189 alternative datasets available for finance, according to Neudata statistics, which were last updated in July.
To be sure, Lipuš says, Neudata continues to receive more requests for election-related data than it did in 2016, the year of the company’s founding. While he could not disclose specific client requests, he said that the range of data they seek is much wider than the last time around, when clients were more focused on predicting a winner and making trades based off that immediate outcome. Now, those firms are asking more industry- and sector-specific questions, and testing different short- and long-term theses.
In other words, election-specific data, such as poll numbers and voter registration statistics, account for only a fraction of election-specific trading strategies being crafted and modeled. Seemingly unrelated datasets—Covid-19 cases, geolocation, and consumer spending, for examples—are just as important in constructing these scenarios.
Here’s one hypothetical example: How does one go about understanding implications for election outcomes for insurers?
A simple, one-faceted way to answer that question could be weighing the idea of former Vice President and Democratic nominee Joe Biden imposing a national mask mandate on all federal property, in addition to other strict coronavirus-related precautions, versus a continuation President Trump’s comparatively more lax approach to controlling the virus. Insurers might be dealt another blow if cases return to their peak levels from March and April and claims rise, particularly as flu season settles in.
In any outcome, the election will likely have a strong impact on small, local businesses. Stricter social-distancing measures passed down by the federal government could further hinder, say, a restaurant’s ability to remain open.
There is also the question of how long it might take for the winner to be decided due to the vast increase in mail-in voting ballots across the country. Hypothetically, if a state like Michigan—which was won by Trump in 2016 by the slimmest margin of any state—is slow to count these votes, that could create high market volatility if this year’s election is as close as 2016 and if it takes weeks to declare a presidential winner. One could also envision outcomes across the country that lead to contentious lawsuits, thus creating more market uncertainty.
Or, maybe 2020 throws us yet another unexpected curveball and the election goes smoothly, the presidential race is decided quickly and decisively, and market turmoil is relatively low.
But no matter what question you ask, there are both short-term and long(er)-term perspectives to consider. The short-term response is mainly driven by sentiment toward potential policies, while the longer-term response is driven by the likelihood of those policies actually being implemented.
“Slightly longer-term, you have to concede that not everything a political party states in its platform ultimately becomes a reality. When trying to understand this, you may turn to one of several vendors that model the likelihood of certain bills and regulations being passed in the US. This process takes into account more factors than just ‘who sits in the White House,’ but also considers political control in the House and Senate, external economic factors, and so on,” says a researcher at Neudata.
Boosted Interest
Boosted.ai, a Toronto-based company that uses machine learning to provide asset managers and hedge funds with portfolio insights, has seen a surge that Joshua Pantony—the company’s co-founder and CEO—calls an enhanced form of scenario analysis, or “what-if” analysis, on its platform, Boosted Insights. What that means is that users are essentially feeding their predictive models hypothetical questions, many of which include scenarios where their machines are purposely not confident about their outputs, in order to hedge against black-swan events that could arise following the election.
“In an example where the VIX [Volatility Index] drops 30% or where interest rates go up, that’s an example where, probably, the system is going to be fairly confident. Here’s the companies that are going to increase in ranking, and here’s [who’s] going to be the likely winners from that, and here’s [who’s] going to be the likely losers from that,” Pantony says. “But in a world where Treasury yields keep going down, or the VIX goes up, it could have less historical training data, and it’s maybe going to be less confident in those results.”
Expecting the unexpected and trying to plan for it isn’t a new practice, and some amount of it was still happening in 2016, but what has changed is that traders and PMs can now utilize complex, nonlinear models that can identify and understand more in-depth and harder-to-see connections that models even of the recent past could not.
For example, you’d historically look for stocks that perhaps correlate to your machine input. But a more sophisticated generation of models can understand that maybe in a world where corporate bonds are getting cheaper, or the Federal Reserve is providing unlimited liquidity, a company holding significant debt might not be in trouble at all.
This year, Pantony says, risk mitigation that works has to be non-standard, noting that many of the traditional risk modeling methods broke down with the onset of the pandemic. For instance, asset classes and industries that traders could normally rely on, such as fixed income and airlines, went practically belly-up. In unprecedented times, model explainability becomes key. When the machines are confident in their outputs, users are more likely to set up trades around the results; when they’re not, the portfolio managers start hedging.
In the grand scheme of democratized data and technology, Neudata’s Lipuš believes the industry—but really, the world—is hitting the point on the adoption curve that transitions from the early adoption stage to majority adoption. And after a year like 2020, the point where they become indispensable—in the way a simple computer or phone is used, in some capacity, for every job there is—might not be too far off.
“It feels like we are about to cross the chasm,” Lipuš says. “I think this year’s been a double whammy with the election and with the [Covid-19] lockdown—and with the focus on innovation, it’s just fast-forwarded everything.”
Only users who have a paid subscription or are part of a corporate subscription are able to print or copy content.
To access these options, along with all other subscription benefits, please contact info@waterstechnology.com or view our subscription options here: http://subscriptions.waterstechnology.com/subscribe
You are currently unable to print this content. Please contact info@waterstechnology.com to find out more.
You are currently unable to copy this content. Please contact info@waterstechnology.com to find out more.
Copyright Infopro Digital Limited. All rights reserved.
As outlined in our terms and conditions, https://www.infopro-digital.com/terms-and-conditions/subscriptions/ (point 2.4), printing is limited to a single copy.
If you would like to purchase additional rights please email info@waterstechnology.com
Copyright Infopro Digital Limited. All rights reserved.
You may share this content using our article tools. As outlined in our terms and conditions, https://www.infopro-digital.com/terms-and-conditions/subscriptions/ (clause 2.4), an Authorised User may only make one copy of the materials for their own personal use. You must also comply with the restrictions in clause 2.5.
If you would like to purchase additional rights please email info@waterstechnology.com
More on Trading Tech
Recent volatility highlights tech’s vital role in fixed income pricing
MarketAxess’ Julien Alexandre discusses how cutting-edge technology is transforming pricing and execution in the fixed income market amid periodic bouts of volatility
Banks fret over vendor contracts as Dora deadline looms
Thousands of vendor contracts will need repapering to comply with EU’s new digital resilience rules
Where have all the exchange platform providers gone?
The IMD Wrap: Running an exchange is a profitable business. The margins on market data sales alone can be staggering. And since every exchange needs a reliable and efficient exchange technology stack, Max asks why more vendors aren’t diving into this space.
This Week: Trading Technologies completes ANS deal; State Street; Equinix; and more
A summary of the latest financial technology news.
Interactive Brokers looks beyond US borders for growth opportunities
As retail trading has grown in volume and importance, Interactive Brokers and others are expanding international offerings and marketing abroad.
JP Morgan’s goal of STP in loans materializes on Versana’s platform
The accomplishment highlights the budding digitization of private credit, though it’s still a long road ahead.
As data volumes explode, expect more outages
Waters Wrap: At least for those unprepared—though preparation is no easy task—says Anthony.
This Week: ICE Bonds and MarketAxess plan to connect liquidity networks, TS Imagine, Bloomberg, and more
A summary of the latest financial technology news.