Ping An: NLP Takes on Greater Importance in Turbulent Times
The firm's chief scientist discusses how NLP is being used to prevent the spread of the coronavirus and how it can be applied for financial services.
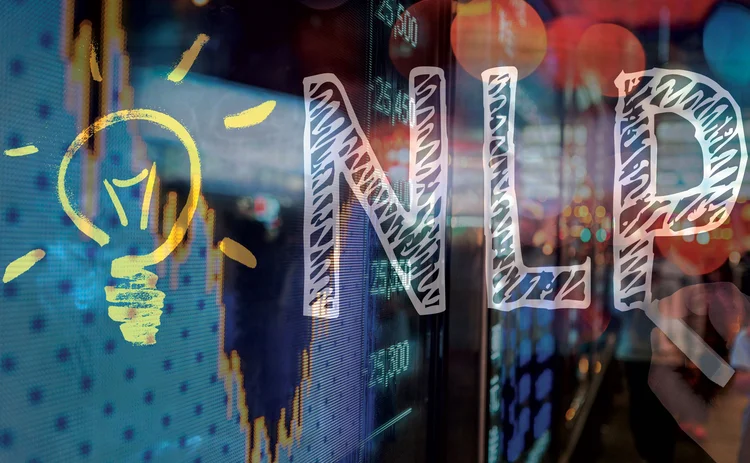
While not a household name in Western nations, the Ping An Insurance Company of China is the country’s largest firm of its kind. And it is becoming a leading company in developing natural language processing (NLP) models.
The firm’s core framework, called Omni-Sinitic, recently bested companies like Microsoft, Google, Alibaba, Huawei and Facebook in the General Language Understanding Evaluation (GLUE) benchmark, for evaluating natural language understanding systems.
The financial services firm also bested competitors in the latest Stanford Question Answering Dataset 2.0 challenge, which is, according to its website, a reading comprehension dataset that combines 100,000 questions with over 50,000 unanswerable questions “written adversarially by crowdworkers to look similar to answerable ones. To do well on SQuAD2.0, systems must not only answer questions when possible, but also determine when no answer is supported by the paragraph and abstain from answering.”
It is the second company, after Google, to achieve a top ranking in both tests.
The ripple effects of the coronavirus pandemic will be felt for years to come. One technology that could prove to be especially useful during these tumultuous times is NLP. Capital markets firms could increasingly lean on NLP for everything from chatbots to extracting information out of dense, unstructured reports from around the globe.
WatersTechnology spoke with Jing Xiao, chief scientist at Ping An, about how the insurance, banking and tech company has built out its NLP capabilities and how these methods are being used in today’s environment.
In one example, Ping An’s Smart Audio Robot is being used to help contain the spread of Covid-19 in Wuhan, China, which was the epicenter of the original outbreak. Using Omni-Sinitic, Ping An came up with the Ping An Covid-19 Smart Audio Robot. Ping An only spent two working days on the robot, equipping it with functions of investigation, follow-up alerts, and the ability to automatically send reminders.
The robot has a daily dial-out volume of over a million times to help with epidemic prevention work. Ping An launched the system on February 18, and as of April 7, it has completed over 1.16 million phone screenings in 47,223 households in 17 communities in Wuhan, and successfully identified more than 2,923 suspected cases for tracking, according to the firm.
To help curb the Covid-19 outbreak, the Wuhan Municipal Government instructed its local epidemic prevention personnel to screen suspected cases by making daily phone calls to gather information on symptoms and body temperatures of residents. Human operators are limited in the number of phone screenings they can make daily, and the accuracy of such calls can vary depending on the operator’s experience and judgment.
Ping An’s audio screening system has the capacity for up to 3,000 AI robots to work at the same time. Each AI robot can handle up to 500 auto-call screenings per day, for a total of 1.5 million calls daily.
On the launch day, the system screened more than 1,200 households in Wuhan, categorizing and reporting health information of citizens. It took five minutes to complete all 1,200 calls, including second attempts, where the first call attempt failed. The audio robot helped epidemic prevention personnel to focus their time and efforts on more important tasks, Xiao says.
A Growing Field
Banks are using new tools like transformer models to develop NLP further. For example, Allianz Global is using these to find signals in sell-side analyst reports. Brown Brothers Harriman is using NLP to transform its fund accounting service. And UBS Asset Management’s Quantitative Evidence and Data Science (QED) unit uses NLP for a variety of data extraction needs. As more tools become available and accessible, financial services firms, be they retail, wholesale, or in capital markets, will start to experiment and further develop their NLP models.
Although not at a capital markets level, Ping An has applied the Omni-Sinitic framework within its various businesses for investment research and risk management needs.
Omni-Sinitic—which has been used for research, telemarketing, training, and interviews—is comprised of a pre-trained language model (ALBERT); an internally-developed adaptive filter that augments the data in the model, otherwise known as a data augmentation by adaptive filtering (DAAF) algorithm; and it uses the neural architecture search (NAS) technique, which is used for designing artificial neural networks.
Based on ALBERT, Google’s “lite” version of its 2018 natural language understanding (NLU) pre-training method BERT—which stands for Bidirectional Encoder Representations from Transformers—Ping An developed its own NAS method. It uses NAS to conduct automatic selection to refine the neural network structure and to integrate the semantic features output by ALBERT into the syntactic features of the sentences.
It also developed DAAF, a model trading framework, to ensure the training data generated can effectively enhance the effects of the model training during the data augmentation process. DAAF contains algorithms that can absorb external data to enhance the model, as well as filter out data that isn’t useful.
As it requires greater calculation volume to determine if the data generated is effective or not, Ping An designed adaptive filtering, which can increase the calculation efficiency at the expense of a minimal loss in training efficiency.
Xiao says that in terms of algorithms, NAS and DAAF are still two independent parts in the current framework. Ping An is in the process of studying how to combine these two parts to form a unified and optimized unit. “In this way, the optimization of the model architecture search and data enhancement process can be performed simultaneously. We believe that there is still room for further improvement in model training,” he says.
Cutting Through the Noise
Xiao says the framework applies to all supervised learning tasks that require an intensive amount of labeled data, including the areas of investment research and risk management. These two scenarios also require a vast amount of text analysis, information extraction, semantic comprehension, news monitoring, and clue discovery to facilitate decision-making.
“DAAF can also be applied to scenarios where there is a large amount of unlabeled data. It can automatically and effectively choose the useful samples to enhance model training. While new samples usually require manual labeling, DAAF can enhance the effects of model training, significantly lowering the labeling cost and increasing training efficiency,” he says.
The key is to create automated tasks to reduce risk brought on by manual processes. During the training of models, such as for NLU—a subset of NLP—Xiao says there might be some errors or “noises” within the data annotation process caused by the different ways that humans label the data. DAAF can mitigate the impact from these types of labeling quality issues and further enhance the precision of the model.
DAAF can solve the problems of insufficient training data, high cost, and low efficiency, and is suitable for cold-start scenarios, where there is no history of information. The framework has been applied to Ping An’s Omni-Sinitic Smart Dialogue Platform, where users only need to consider the design of dialogue logic, leaving the semantic comprehension tasks up to the algorithm.
Omni-Sinitic allows users to take the best qualities of their top-performing service representatives and use those skills to build out the robot’s communication capabilities automatically.
“The robot will help complete the mechanical work, and improve production efficiency, thereby providing high-quality services for more customers,” he says.
Further reading
Only users who have a paid subscription or are part of a corporate subscription are able to print or copy content.
To access these options, along with all other subscription benefits, please contact info@waterstechnology.com or view our subscription options here: http://subscriptions.waterstechnology.com/subscribe
You are currently unable to print this content. Please contact info@waterstechnology.com to find out more.
You are currently unable to copy this content. Please contact info@waterstechnology.com to find out more.
Copyright Infopro Digital Limited. All rights reserved.
As outlined in our terms and conditions, https://www.infopro-digital.com/terms-and-conditions/subscriptions/ (point 2.4), printing is limited to a single copy.
If you would like to purchase additional rights please email info@waterstechnology.com
Copyright Infopro Digital Limited. All rights reserved.
You may share this content using our article tools. As outlined in our terms and conditions, https://www.infopro-digital.com/terms-and-conditions/subscriptions/ (clause 2.4), an Authorised User may only make one copy of the materials for their own personal use. You must also comply with the restrictions in clause 2.5.
If you would like to purchase additional rights please email info@waterstechnology.com
More on Data Management
New working group to create open framework for managing rising market data costs
Substantive Research is putting together a working group of market data-consuming firms with the aim of crafting quantitative metrics for market data cost avoidance.
Off-channel messaging (and regulators) still a massive headache for banks
Waters Wrap: Anthony wonders why US regulators are waging a war using fines, while European regulators have chosen a less draconian path.
Back to basics: Data management woes continue for the buy side
Data management platform Fencore helps investment managers resolve symptoms of not having a central data layer.
‘Feature, not a bug’: Bloomberg makes the case for Figi
Bloomberg created the Figi identifier, but ceded all its rights to the Object Management Group 10 years ago. Here, Bloomberg’s Richard Robinson and Steve Meizanis write to dispel what they believe to be misconceptions about Figi and the FDTA.
SS&C builds data mesh to unite acquired platforms
The vendor is using GenAI and APIs as part of the ongoing project.
Aussie asset managers struggle to meet ‘bank-like’ collateral, margin obligations
New margin and collateral requirements imposed by UMR and its regulator, Apra, are forcing buy-side firms to find tools to help.
Where have all the exchange platform providers gone?
The IMD Wrap: Running an exchange is a profitable business. The margins on market data sales alone can be staggering. And since every exchange needs a reliable and efficient exchange technology stack, Max asks why more vendors aren’t diving into this space.
Reading the bones: Citi, BNY, Morgan Stanley invest in AI, alt data, & private markets
Investment arms at large US banks are taken with emerging technologies such as generative AI, alternative and unstructured data, and private markets as they look to partner with, acquire, and invest in leading startups.