Quant shop preps NLP-powered index for physical climate risk
Sharp rise in extreme weather events prompts PGIM Quant to aim for better climate-risk pricing
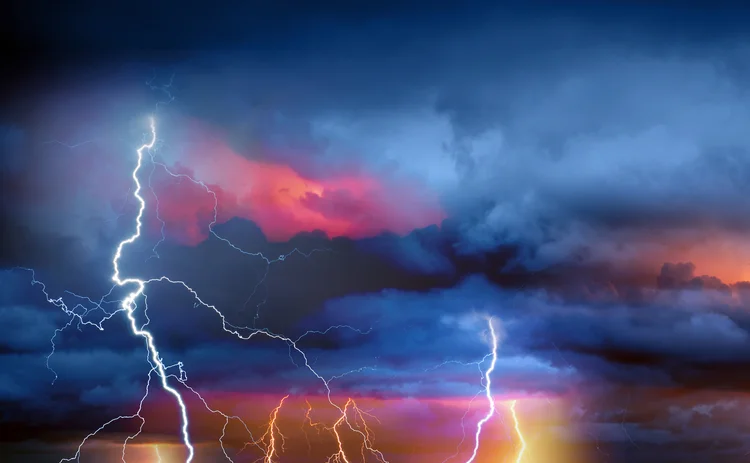
Prudential Investment Management Quantitative Solutions (PGIM Quant) is trying a new tack to price physical climate risk more accurately. It’s building an index to measure this specific subset of risks based on natural language processing (NLP)—the field of artificial intelligence in which computers interpret written or spoken language.
The AI initiative began in response to the rising frequency and intensity of extreme weather events across the globe, says the firm. In the US alone, data from the National Oceanic and Atmospheric Administration shows that from 1980 to 2022 the annual average number of such events was eight. But for 2018 to 2022—the past five years of data—this average has shot up to 18.
Unlike so-called transitional climate risks – which are associated with the shift to a lower-carbon economy and use carbon emissions as a proxy—physical climate risks include the risk of damage to assets, of operational interruptions and disruptions to supply chains due to climate change. They include short-term risks triggered by events like floods or storms, and longer-term risks stemming from prolonged shifts in climate patterns.
I fear there’s a lot of noise in this space and there’s been a lot of comment around differences in ESG ratings across vendors
Gavin Smith, PGIM Quant
Creating proxies for these physical risks is much more complex than it is for transitional risks, says Gavin Smith, head of equity research and sustainable investing at PGIM Quant. It was after finding significant divergence in third-party physical risk assessments that the group turned to NLP to create its own bespoke indicator that draws on news sentiment.
“I fear there’s a lot of noise in this space and there’s been a lot of comment around differences in ESG ratings across vendors,” says Smith, referring to the lack of uniform metrics for environmental, social and governance factors. “If you look at differences in these physical risk assessments… you can see large variations, so I’ve got some healthy skepticism towards those methodologies.”
Attempts to determine the value-at-risk associated with these physical risks are typically model-intensive, says Smith. When assessing the vulnerability of a construction company to extreme heat, for example, the approach might combine multiple elements—such as the potential number of working days that could be lost due to extreme heat, modeled temperature increases and predictions, the economic impact of days lost, and any number of other factors. It’s a process that introduces estimation errors, adds Smith.
“You have to go through and model each of these and then think of different scenarios and different modeling around different climates – whether it’s rainfall, snowfall, high and low temperature and so forth,” says Smith. “Model upon model. The estimation error around this is quite significant.”
PGIM Quant’s physical risk index attempts to rationalize this. Firstly, the AI identifies all the news stories associated with various kinds of weather events. Next, it determines whether the sentiment of the stories is positive or negative. This score is then used to create an index of companies’ physical risks and, finally, the firm looks at how sensitive these stock prices are to the index.
Smith acknowledges that the process relies on the wisdom of the markets in that the same news stories will inform investors, who will also be processing this information and moving stock prices accordingly.
“It’s still an estimation, so it’s still imperfect,” he says. “But we feel that it’s a little more parsimonious and transparent as well.”
The bigger picture
The index is part of a broader material risk framework created by PGIM Quant that captures an array of risks, including customer privacy issues, data security, labor practices and supply-chain management. The framework is integrated within the firm’s risk management process across its active quantitative strategies and embedded as a constraint in the portfolio optimization process. The aim is explicitly to minimize exposure to high-risk companies.
While the material risk framework weeds out unfavorable investments, it can also be integrated with other ML- and NLP-derived indicators that are specifically designed to identify companies actively developing green products or solutions that address climate change—an application, Smith says, that is particularly suitable for quantitative analysis.
PGIM Quant recently worked with fintech provider Util, for example, to leverage ML to analyze around 100 million peer-reviewed academic publications to identify companies with higher levels of revenue from products aligned with the UN’s Sustainable Development Goals.
This information is then cross-referenced with valuations to ensure investors aren’t overvaluing a company’s quality factor score – ensuring it hasn’t overinvested or overstretched its financial position when investing in developing new products and services. And, finally, a material risk framework helps assess whether any meaningful risk arises from how its products are produced.
“This is a really attractive alternative to the risk mitigation part of the discussion,” says Smith: “Because [it] opens up more secular growth.”
The index is currently in the research and development phase, say officials, with no information on its timing yet available.
Only users who have a paid subscription or are part of a corporate subscription are able to print or copy content.
To access these options, along with all other subscription benefits, please contact info@waterstechnology.com or view our subscription options here: http://subscriptions.waterstechnology.com/subscribe
You are currently unable to print this content. Please contact info@waterstechnology.com to find out more.
You are currently unable to copy this content. Please contact info@waterstechnology.com to find out more.
Copyright Infopro Digital Limited. All rights reserved.
As outlined in our terms and conditions, https://www.infopro-digital.com/terms-and-conditions/subscriptions/ (point 2.4), printing is limited to a single copy.
If you would like to purchase additional rights please email info@waterstechnology.com
Copyright Infopro Digital Limited. All rights reserved.
You may share this content using our article tools. As outlined in our terms and conditions, https://www.infopro-digital.com/terms-and-conditions/subscriptions/ (clause 2.4), an Authorised User may only make one copy of the materials for their own personal use. You must also comply with the restrictions in clause 2.5.
If you would like to purchase additional rights please email info@waterstechnology.com
More on Data Management
New working group to create open framework for managing rising market data costs
Substantive Research is putting together a working group of market data-consuming firms with the aim of crafting quantitative metrics for market data cost avoidance.
Off-channel messaging (and regulators) still a massive headache for banks
Waters Wrap: Anthony wonders why US regulators are waging a war using fines, while European regulators have chosen a less draconian path.
Back to basics: Data management woes continue for the buy side
Data management platform Fencore helps investment managers resolve symptoms of not having a central data layer.
‘Feature, not a bug’: Bloomberg makes the case for Figi
Bloomberg created the Figi identifier, but ceded all its rights to the Object Management Group 10 years ago. Here, Bloomberg’s Richard Robinson and Steve Meizanis write to dispel what they believe to be misconceptions about Figi and the FDTA.
SS&C builds data mesh to unite acquired platforms
The vendor is using GenAI and APIs as part of the ongoing project.
Aussie asset managers struggle to meet ‘bank-like’ collateral, margin obligations
New margin and collateral requirements imposed by UMR and its regulator, Apra, are forcing buy-side firms to find tools to help.
Where have all the exchange platform providers gone?
The IMD Wrap: Running an exchange is a profitable business. The margins on market data sales alone can be staggering. And since every exchange needs a reliable and efficient exchange technology stack, Max asks why more vendors aren’t diving into this space.
Reading the bones: Citi, BNY, Morgan Stanley invest in AI, alt data, & private markets
Investment arms at large US banks are taken with emerging technologies such as generative AI, alternative and unstructured data, and private markets as they look to partner with, acquire, and invest in leading startups.