Quants Use Nowcasting As Covid Crystal Ball
Experts from UBS, Unigestion, MIT and QuantConnect discuss the need for nowcasting, and what the alt data boom has made possible in trying to navigate today’s crisis.
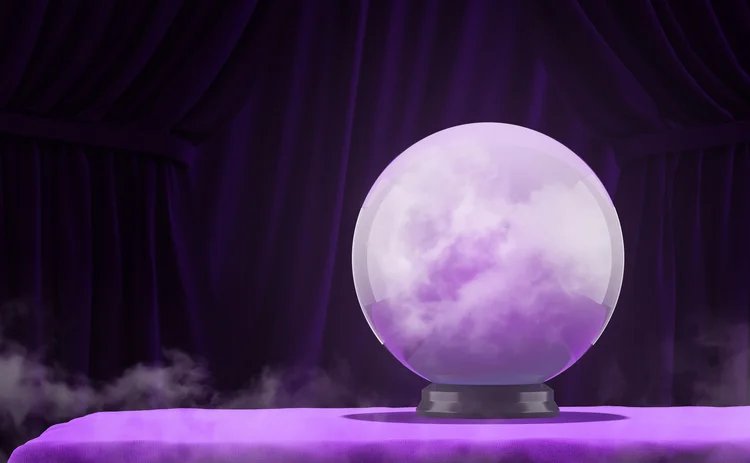
As the effects of the coronavirus continue to ripple through parts of the world and its economies, quants are navigating the pathogen and its effects with the help of nowcasting—a portmanteau of “now” and “forecasting” that uses unstructured and structured data to predict possible scenarios happening at present or in the very near future.
The concept of nowcasting isn’t new, but it’s gained notoriety throughout the crisis as investors, researchers, and analysts are hungry for real-time data on things that aren’t normally available in real-time, including GDP, employment, inflation, and other economic indicators.
Originating in 1980 and coined by British meteorologist Keith Browning, it has expanded into the fields of healthcare and finance—most notably, by central banks, such as the Federal Reserve Bank of New York, which in 2017, laid out its approach to nowcasting the economy using big data in a staff report. The Federal Reserve Bank of Atlanta is another such example, as it hosts a running estimate of real GDP, called GDPNow, which it says is similar to the one used by the US Bureau of Economic Analysis.
In 2015, CAIA Association member Alexander Ineichen published a report which argued nowcasting could be used as a new risk management tool, citing one of the downfalls of forecasting using data with longer lag times: “At the beginning of 2014, 72 out of 72 economists ‘predicted’ that U.S. interest rates would rise throughout the year. They fell.”
The coronavirus, however, has shed new light on prediction model. Its need is most pressing when conditions are shifting rapidly. That’s where alternative data has stepped in as the differentiator from past financial crises. Nowcasting married with the alt data boom—which has made metrics such as foot traffic, satellite imagery, and web-scraped sentiment commonplace—has added a useful tool to the quant arsenal.
Navigating the economic effects of the coronavirus has meant working with virtually no benchmarks or base rates, says Jeremy Brunelli, a data specialist in UBS’s Evidence Lab, which works with analysts, traders, and portfolio managers across asset management and UBS O’Connor, the bank’s multi-strategy hedge fund. It uses artificial intelligence (AI) to solve bespoke problems, and sources alternative datasets to drive excess returns for investors. The team has been occupied with understanding which countries are flattening their curves of new virus cases, how consumers have changed their behaviors, and whether previous behaviors are returning, relating to that deceleration.
“Right now, things are moving so fast. I would liken this period—and there are a lot of differences—to my experience when the [2008] financial crisis happened. But really, it’s very similar in the sense that traditional measures didn’t work anymore. Things were changing hour to hour, day to day. And so, I leveraged a lot of alternative datasets back then to try to get a grasp of what was going on,” he says.
The Evidence Lab Nowcasting Model uses 20,000 data sources to create signals for various markets—for example, automation equipment or farm fertilizer—which make up the broader economy. The lab creates 50 to 60 different market signals for North America, Europe, and Asia. It recently used foot traffic and public transit ridership, paired with pollution data, to understand which industries are contributing more pollutants, therefore indicating a return to ‘normalcy,’ and to paint the picture of how a recovery unfolds.
An important distinction is that Evidence Lab adheres to a Bayesian technique, which Brunelli describes as a mosaic. It involved working with a lot of different data points, none of which are meant to offer a discrete forecast, per se, but point to an increase or decrease of confidence in a given hypothesis. So, for example, they collect five data points related to one central idea but aren’t related to one another. Three of them may show a positive signal, while two are neutral—that shows that something positive is likely happening there.
The technique is especially useful when working with data points that don’t have long histories, which is the case for many alt datasets. There is a risk, though, as this process means that sometimes users have to accept more significant standard deviations, but it’s a way to make decisions when you have to make them quickly and with many factors, Brunelli says.
A Delicate Blend
Geneva-based asset manager Unigestion has relied on nowcasting in its investment process for several years. Its proprietary tool, Nowcaster, is used to create regimes in three categories—growth, inflation, and market stress—for strategic asset allocation and dynamic asset allocation, a long-term and a short-term active investment strategy, respectively.
The investment manager added an alt data extension to the tool, specifically using news sentiment, to measure and predict inflation, in tandem with data from RavenPack, which provides stock-specific and macro sentiment analysis and scores, says Robert Kosowski, head of quantitative research for Unigestion. They compared the impact of alt data to the traditional Nowcaster system, finding two interesting takeaways.
First, incorporating macro news sentiment into traditional nowcasting—Unigestion calls these “newscasters” internally to delineate between the two—were historically leading the actual time-series information. That was useful for asset allocation as it allowed the firm to anticipate market changes earlier. Second, after allocating across asset classes and within asset classes at different premia, they found that “newscaster” performance, on average, was actually superior to using Nowcaster as a standalone.
Is alt data important to nowcasting at Unigestion? The short answer is yes, but Kosowski stresses that it’s more useful to treat alt data as a separate category, and then figure out how to combine it with the traditional market and reference datasets.
“What I mean by that is imagine you have 100 time-series that you use for your nowcast—all of them traditional—and now comes along series 101,” he says. “Now one of the reasons why we find it doesn’t work if you just add it, is the averaging—you’ve got 100 time-series, one comes along—it would naturally have a lower weight.”
Unigestion, instead, overlays the news data on top of its first prediction that uses its historical time-series data, to determine how announcements might affect the predicted outcome.
Seeing the Future
In late March 2020, Marcos Lopez de Prado of Cornell University and Alexander Lipton, a connection science fellow at MIT, released a joint study, “Three Quant Lessons from Covid-19,” which argues the importance of nowcasting in quantitative finance going forward.
The study bases its argument on the grounds that quant funds could have been better prepared for the Covid-induced sell-off. The SARS-CoV-2 virus was isolated on Feb. 11, 2020; despite that, the market continued to rally, with the S&P 500 peaking at an all-time high eight days later. Nowcasting, the study contends, helped certain market-makers to handle the coming volatility, as these prediction models showed supply-chain disruption from government-led lockdowns earlier than traditional forecasting models.
“Days before the Covid-19 sell-off started, there were plenty of warning signs that the virus was disrupting critical supply chains in China,” the study states. “Thanks to their nowcasting of order-flow imbalance, very few market makers experienced losses during the sell-off.”
- Read More: This study is part of a broader project by Lipton and Lopez de Prado, in which they’re modeling an array of Covid-specific scenarios and their effects. See “The Shape of Covid” at the bottom of the page.
Lipton, a former executive at several financial institutions—including Bank of America, Merrill Lynch (and the combined company), and Citadel LLC—tells WatersTechnology that nowcasting, when done right, is about thinking outside the box. Time-series and market data aren’t and won’t be enough to get through this crisis. And it should teach firms that they need to be nimble and reactive enough to adjust their trading strategies as easily as winds change.
“Be agile and be omnivorous, yes,” Lipton says. “But having said that, there are good mushrooms in the forest and there are poisonous mushrooms, so you need to be able to figure out which is which. And it’s not always possible, but you need to have some guidance. And the guidance is: Don’t look for back-testing, per se, but look for more theories.”
By that, he doesn’t mean to build a whole theory of the economy but look for and determine causality between different data streams.
“Have a good idea why things happen, rather than just simply observing how they evolve,” he says.
Of course, some things cannot be predicted. Nowcasting cannot predict the time nor location of, say, an earthquake, though once one is detected, in a very short amount of time and with high accuracy, it can tell which regions will be impacted by the shock wave and which coastal sites will be impacted by the tsunami. The example translates to the markets. Nowcasting couldn’t predict that there would be a pandemic of this magnitude, but once it started to gain traction, nowcasting could have been used to identify areas that would be hit the hardest, as well as where ripple effects would be felt and to what degree of intensity.
By its very nature, and when used appropriately, nowcasting dictates that what works today will likely not work for tomorrow; that’s the beauty and the challenge of these models—they aim to predict the “now.” Undoubtedly, firms will use some of this time to create trading strategies that can help with a similar future scenario—but they shouldn’t, Lipton says. The next time around, there will be other foreign and unprecedented factors, and today’s pandemic trading strategies won’t fit the bill. That brings Lipton back to the key pillar of the study: the ability to pivot.
Nowcasting in Motion
The pandemic has been something of a proving ground for technologies and strategies, alike, as trading firms are literally having to put their money where their anthropomorphic mouths are. As the world becomes increasingly interconnected, Lipton says pandemics happen with “depressing periodicity, and in fact, this periodicity is increasing,” making the case that increased interest in nowcasting is more likely an emerging trend than a blip.
Jared Broad, CEO of crowd-sourced algo trading platform QuantConnect, says it’s been exciting to see nowcasting algos tick up in usage over the last few months. The vendor allows quants and programmers to test their hypotheses on its platform, then sell the algorithm’s licensing rights on its Alpha Streams marketplace, where quant funds and other proprietary trading shops can compete for those rights.
He adds that these nowcasting algos have allowed quants to perform reasonably well, compared to the market. Alpha Streams quants deploying a nowcasting algo have seen drawdowns between 10% and 20%, whereas the S&P 500 suffered 33.9% between its February peak and April 2, Broad says.
Nowcasting’s rise mirrors a discussion taking place in the value-versus-momentum space, Broad adds. Some investors—such as those who practice ESG, impact, or passive investing—are trading on value indicators—for example, carbon emissions or energy efficiency—that make more sense if you buy and hold. On the flip side, as the market is rife with volatility, the companies trading on momentum are doing well—sometimes better.
“Looking at what is and looking at what will be in the very short-term future is a way to sort of increase your chances of success by making a shorter-term prediction on where the world will be in the next hours, days, [or] maybe a week,” Broad says. “So with that core thesis, you can change the way you experiment with data.”
Nowcasting’s usefulness in portfolios, stock choice, and trading strategy largely revolves around volatility and outright crisis, so it’s certainly not a silver bullet for all markets all the time. But as geographies and economies become increasingly interconnected, volatility will be a constant, even when seas seem smooth.
As for what will happen tomorrow, the nowcasters can only guess.
“Now we’re getting a lot of questions about what’s next: ‘What is the new normal?’” says UBS’s Brunelli. “And that’s becoming more of a future-casting, kind-of-difficult thing to predict. And that’s where nowcasting probably doesn’t do well.”
The Shape of Covid
Professors Lipton and Lopez de Prado are collaborating on a large Covid-19 project. The report mentioned above is only one part. The two are using the study in their efforts to build models for other aspects of the disease beyond its ramifications in finance.
As our sister publication, Risk.net, previously reported, the duo is using options pricing and machine learning to gauge the wider cost of virus control measures and help leaders decide when to lift lockdowns. Additionally, and in collaboration with academics from the Hebrew University of Jerusalem—where Lipton is also a fellow—Lipton hopes to assess the effects of children returning to school.
In April, they released another study, “Exit Strategies for COVID-19: An Application of the K-SEIR Model.”
“In this study, we introduce a new mathematical model (called K-SEIR) to simulate the outcomes of lockdowns, and help evaluate various exit strategies. We demonstrate that targeted lockdowns can achieve better outcomes than universal lockdowns, in terms of (1) saving lives, (2) protecting the most vulnerable in society (the elderly, the poor), and (3) preventing the depletion of medical resources,” the abstract reads.
“K” stands for “K-groups,” and “SEIR” stands for “susceptible, exposed, infected and removed.”
When I call Lipton on June 1, he’s in the middle of writing the project’s most comprehensive study thus far, an examination of what he calls an “unprecedented failure of epidemiology as a science.”
Typically, epidemiologists react to pandemics and epidemics either through top-down approaches—looking at the population as a general whole and making indiscriminate mandates—or bottom-up approaches—which calls for self-motivated, community-led measures which rely on assumptions of what individuals’ habits and conditions are.
“What happened was both models proved to be completely wrong,” Lipton says. “And the predictions were really catastrophic. And governments acted on this. But the truth of the matter is that what they actually used was a uni-model approach to this particular epidemic, by which I mean they treated the entire population with the same brush, so to speak. This particular pandemic is actually distinctively bi-model in nature.”
By that, he means there are two large, distinct groups—older people and those with comorbidities, and younger, healthier people. By creating a model for each group, they have been able to analyze in much finer detail, what potentially should have been done instead and how those measures might have been carried out.
“That’s what we’ve been working on, and we drew some conclusions, not that those conclusions really had a tremendous impact on the powers that be, but at least we articulated what we felt is the right approach to the problems. … We will see. Time will tell. Time will tell who was right.”
Further reading
Only users who have a paid subscription or are part of a corporate subscription are able to print or copy content.
To access these options, along with all other subscription benefits, please contact info@waterstechnology.com or view our subscription options here: http://subscriptions.waterstechnology.com/subscribe
You are currently unable to print this content. Please contact info@waterstechnology.com to find out more.
You are currently unable to copy this content. Please contact info@waterstechnology.com to find out more.
Copyright Infopro Digital Limited. All rights reserved.
As outlined in our terms and conditions, https://www.infopro-digital.com/terms-and-conditions/subscriptions/ (point 2.4), printing is limited to a single copy.
If you would like to purchase additional rights please email info@waterstechnology.com
Copyright Infopro Digital Limited. All rights reserved.
You may share this content using our article tools. As outlined in our terms and conditions, https://www.infopro-digital.com/terms-and-conditions/subscriptions/ (clause 2.4), an Authorised User may only make one copy of the materials for their own personal use. You must also comply with the restrictions in clause 2.5.
If you would like to purchase additional rights please email info@waterstechnology.com
More on Trading Tech
Recent volatility highlights tech’s vital role in fixed income pricing
MarketAxess’ Julien Alexandre discusses how cutting-edge technology is transforming pricing and execution in the fixed income market amid periodic bouts of volatility
Banks fret over vendor contracts as Dora deadline looms
Thousands of vendor contracts will need repapering to comply with EU’s new digital resilience rules
Where have all the exchange platform providers gone?
The IMD Wrap: Running an exchange is a profitable business. The margins on market data sales alone can be staggering. And since every exchange needs a reliable and efficient exchange technology stack, Max asks why more vendors aren’t diving into this space.
This Week: Trading Technologies completes ANS deal; State Street; Equinix; and more
A summary of the latest financial technology news.
Interactive Brokers looks beyond US borders for growth opportunities
As retail trading has grown in volume and importance, Interactive Brokers and others are expanding international offerings and marketing abroad.
JP Morgan’s goal of STP in loans materializes on Versana’s platform
The accomplishment highlights the budding digitization of private credit, though it’s still a long road ahead.
As data volumes explode, expect more outages
Waters Wrap: At least for those unprepared—though preparation is no easy task—says Anthony.
This Week: ICE Bonds and MarketAxess plan to connect liquidity networks, TS Imagine, Bloomberg, and more
A summary of the latest financial technology news.