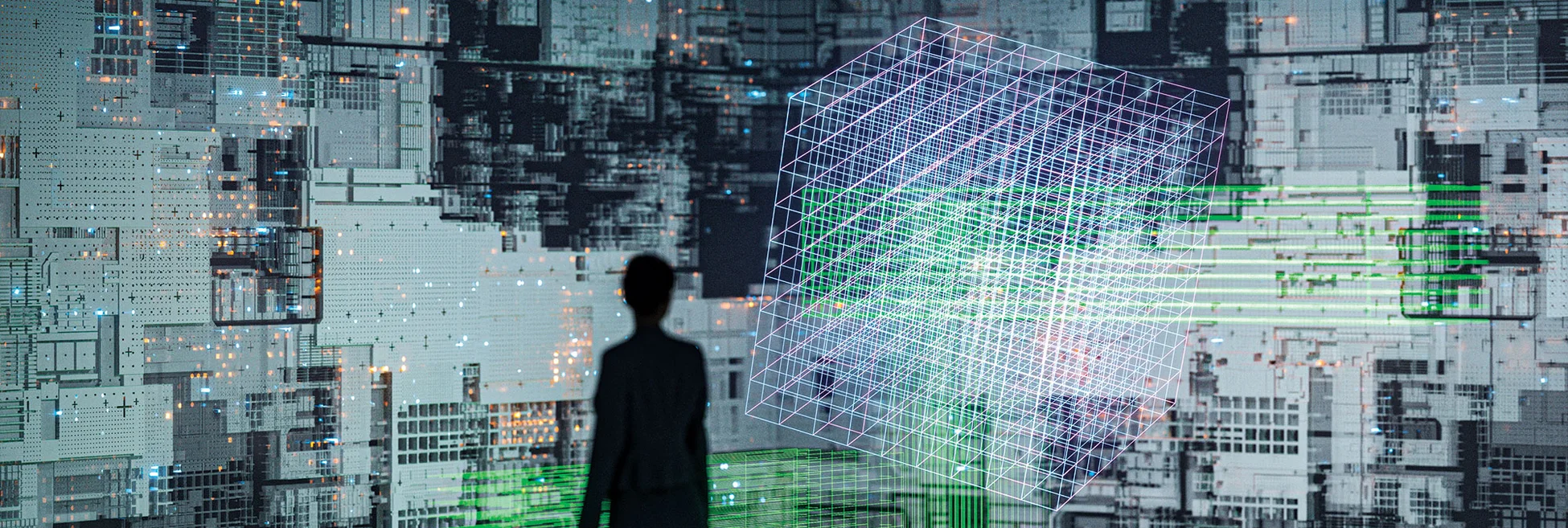
Science Friction: Some Tire of Waiting for Quantum’s Leap
Use cases for quantum computing are piling up—from CVA to VAR. But so are the obstacles
Need to know
- Embryonic quantum computers are being rented by banks keen to test the revolutionary new technology.
- In theory, the machines are perfect for complex, multivariate analysis. In practice, today’s devices lack the scale to handle real-life datasets.
- ABN Amro, Barclays, BBVA, NatWest, Nomura and JP Morgan are among the many banks experimenting with quantum tech.
- Applications explored so far include credit valuation adjustment, economic capital, regulatory stress-testing and value-at-risk.
- In one test, NatWest showed it would be 300 times quicker to use a quantum computer to calculate the optimal make-up of its liquidity buffers.
- But with off-the-shelf tech still distant, some banks are questioning their involvement. “Two years ago, I was a lot more bullish,” says the chief digital officer for Nomura’s wholesale businesses.
If qubits—the building blocks of a quantum computer—were humans, no-one would employ them. They would be unreliable. They would forget everything they learned. Only trivial jobs could be entrusted to them, and then only after painstakingly marshalling them into teams. On top of all that, they would insist on meticulously prepared and maintained workspaces.
The work itself, though, would happen at lightning speed.
That promise—of a literal quantum leap in computing power—has moved beyond the purely theoretical to the halfway practical, with banks and specialist developers now testing its use in everything from derivatives valuation to the management of liquidity buffers. Using the technology in the real world, though, will require fixes for all those qubit niggles—the behavioral quirks that make them so frustrating to work with.
Practical quantum computers can’t be used for a lot of tasks that we typically face in 98% of financial domain applications
Jezri Mohideen, Nomura
Lee Braine, director of research and engineering at Barclays, casts this as “a voyage of discovery that could lead to radically novel solutions for some of today’s greatest computational challenges”.
Others see it as a slog. Some banks are now reining in their expectations—and potentially their investment of time and money.
The head of digital at one investment bank says: “It always feels like we’re two years away from a real quantum use case. The order of magnitude of the efficacy of the processing just wasn’t where everybody thought it would be.”
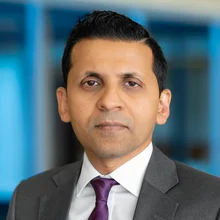
Jezri Mohideen, global chief digital officer for Nomura’s wholesale business has also become more sceptical, at least in the short term. He says the bank may wait before becoming an adopter: “Two years ago, I was a lot more bullish, and I felt the evolution would come through a lot faster. Practical quantum computers can’t be used for a lot of tasks that we typically face in 98% of financial domain applications.”
To tell this story—of quantum’s huge potential in the risk management and risk transfer markets, and of the barriers to it—WatersTechnology's sibling publication Risk.net has spoken to more than 20 sources, including banks that have tested the technology, hardware manufacturers of the kit and software developers devising uses for it. Everyone is in on the act, from domestic and regional players such as Spain’s BBVA, to global titans such as JP Morgan.
The choice each of them has to make is how and when to engage with technology that may still be a decade away from being useful to their businesses.
Qubit quirks
Understanding the practical obstacles in quantum computing requires some understanding of the qubit.
While a bit within a classical computer can be in one of two states—storing either a one or a zero—a quantum bit, or qubit, can exist in both states simultaneously, a phenomenon known as superposition. This means quantum computers can handle a vast number of calculations. The behavior of qubits can also be correlated—or ‘entangled’—so observing one qubit can tell you something about the state of others, cutting the time required to collect information from the system.
These qualities make quantum computers ideal for handling complex calculations with large numbers of variables. Or, they would, if qubits could be trusted.
Classical bits retain their states—zero or one—because the materials and electronics in classical computers provide stability. If a bit changes state in error, it can be detected and fixed. In quantum computing, errors can be caused if qubits get too warm, or as a result of stray microwaves and photons, or manufacturing defects. As a result, they have to be refrigerated and shielded from the outside world.
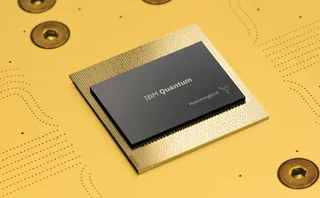
They also have to be knitted together—multiple ‘physical qubits’ are required to produce one ‘logical qubit’ that has a low error rate and can be reliably programmed.
IBM’s current machines max out at 65 physical qubits, in its Hummingbird device, debuted this year. In September, the IT giant laid out ambitious plans to build a 127-qubit processor next year, a 433-qubit device in 2022 and a 1,121-qubit machine by the end of 2023.
This would be dramatic progress, but still not enough for quantum machines to handle tasks that are beyond their classical cousins—for this, thousands of qubits will be required.
In addition, more robust quantum random access memory is required, to permit better storage of data during applications—essential for data-heavy use cases such as machine learning.
Until these upgrades arrive, today’s generation of machines—catchily known as noisy intermediate-scale quantum (Nisq)—have some pretty serious limitations.
“The algorithms we devise are still running on fictitious data,” says Marco Pistoia, head of research and engineering at JP Morgan.
“We’re not really analyzing the data of the bank, because the computer would not be able to perform a computation on such a large input. We have to wait for the hardware to make progress.”
These proofs of concept may be limited, but they can still be valuable.
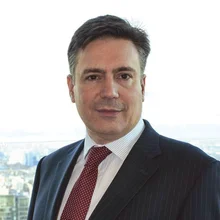
“It doesn’t mean the result is trivial—far from it,” says Braine at Barclays, which has run its own proofs in the area of netting and settlement, with work on front-office machine learning to come. “You’re actually performing often novel research with original contributions to knowledge. But moving from that to deploying it in industry is a very big step.” The hardware necessary to scale such solutions is five to 10 years away, he reckons.
This creates a conundrum: in order to capitalize on quantum technology, banks need some level of expertise—as well as answers to some basic questions about which applications to prioritize. But how much energy should they expend before the technology reaches its tipping point?
Dutch bank ABN Amro is pooling resources with two of its compatriots, ING and Rabobank, as a way to explore quantum—but limit the cost—as the technology develops.
“The amount of resources that you get for doing quantum projects are not sky-high—and, due to Covid-19, are even lower. So we are finding common ground and working together,” says Dimitri van Esch, quantum lead at ABN Amro. The trio has been looking for a quantum short-cut to regulatory stress-testing.
At the other end of the spectrum, JP Morgan created a new ‘lab’ late last year to explore quantum and other emerging technologies, poaching Pistoia to lead it. The 24-year IBM veteran left his former employer with the title ‘master inventor’ because of his record of producing valuable patents. At the US bank, he has been let loose on cutting-edge quantum hardware from Honeywell that seeks to make today’s little knots of qubits more productive—a technique known as mid-circuit measurement and reuse.
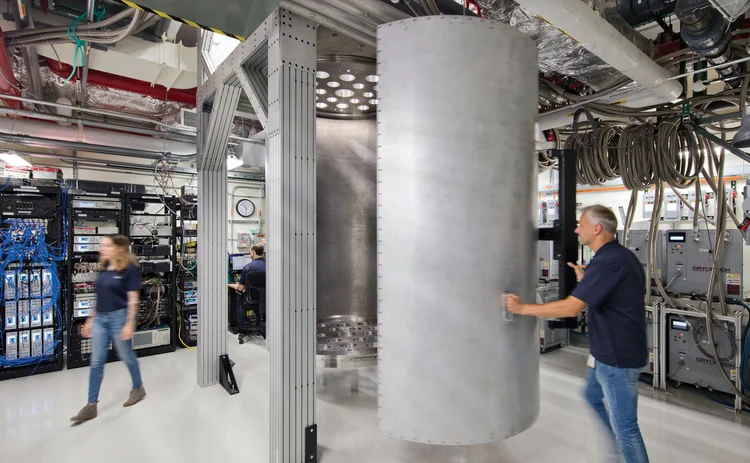
Pistoia says he was surprised, after arriving from IBM, that JP Morgan had access to such advanced technology. One of the early fruits of his labors is a co-authored research paper about how to write algorithms for use on the Honeywell device, published last month.
“JPMorgan Chase has been the first research organization worldwide, as far as we know, to devise an algorithm that takes advantage of this,” he says.
The advance means the bank “can now measure a qubit in the middle of a circuit and then reuse it. In the previous hardware environment, the measurement had to be done all at the end. So the qubits can yield more power than before, because they can be reused”.
Pistoia says the research, published on September 14, is particularly important in quantum algorithms for tasks ranging from options pricing to machine learning.
Inside the lab
With so many banks conducting research, the number of future applications for quantum computing is piling up. In most of these cases, banks are renting Nisq machines from a manufacturer—IBM has around two dozen—accessing them through the cloud, and applying them to toy datasets.
Some of the early results have been eye-popping.
NatWest discovered in one experiment that it was 300 times faster to optimize its £120 billion ($155.2 billion) high-quality liquid asset (HQLA) portfolio using a quantum algorithm. But the test could only be applied to a test portfolio of around 90 different assets, rather than the real-world HQLA book, containing in the region of 500.
Jas Oberoi, director of machine learning and quantum-inspired solutions at 1Qbit, NatWest’s Canada-based software developer partner, says: “We are waiting for the hardware to scale to a point where a practically useful problem can be solved better than traditional computing offers today.” Like Braine at Barclays, he believes this tipping point is five to 10 years away.
There are many other possible uses for the technology. Monte Carlo simulations appear throughout finance whenever a firm needs to derive a probability distribution from an array of possible paths—in stress-testing, derivatives pricing and portfolio risk models, for example. The equivalent of these calculations can be carried out on a quantum machine via what’s called an amplitude estimation algorithm, only far more rapidly.
JP Morgan’s Pistoia describes this as “low-hanging fruit”: “Imagine if you have to search through a million elements. With a quadratic improvement in complexity, the number of iterations of the algorithm is reduced to only 1,000.”
Imagine if you have to search through a million elements. With a quadratic improvement in complexity, the number of iterations of the algorithm is reduced to only 1,000
Marco Pistoia, JP Morgan
And time is money. If banks are able to obtain quicker answers to complex questions, then the resulting information can inform their decision-making, says Stefan Woerner, quantum applications research and software lead at IBM Quantum, a manufacturer of quantum computers.
He gives the example of economic capital calculations for a large portfolio of assets. A paper co-authored by Woerner last year showed that a yet-to-be-built, fault-tolerant quantum machine would estimate the risk and the required capital for a portfolio more precisely than a classical computer, while delivering results in less than a minute, rather than hours or days.
He tells Risk.net: “If a bank can do this risk analysis for its whole portfolio in real time, it can provide much more competitive prices.”
CogniFrame, a Canada-based algorithm developer that leverages quantum computing in a hybrid model with classical methods, has worked on a host of research projects and applications, including an attempt to improve margins on an energy trading desk, optimization of a hedging portfolio for a currency trader and exotic options pricing for a large information services provider.
CogniFrame founder and chief executive Vish Ramakrishnan says: “Pricing exotic options was] too much for the hardware that’s available today, so we’re only able to do it more as a proof of concept. Even a basic options basket would require hundreds of [logical] qubits to have reasonable accuracy. That’s definitely not going to be happening in the next three or four years. It’s probably 10 years away.”
CCAR, CVA and VAR
While quantum-powered options pricing may be out of reach for another decade, some experts argue Nisq devices can start picking up simpler work in the much shorter term.
US-based software developer Zapata Computing has applied for a patent on the application of its quantum software to the US Federal Reserve’s annual stress test of large banks, the Comprehensive Capital Analysis and Review (CCAR). This is essentially the same use case that is being explored by the trio of Dutch banks.
The developer’s chief executive, Christopher Savoie, claims Zapata can complete the tests “much more quickly and much more accurately” on today’s quantum devices.
“We figured out how to do [CCAR] on a Nisq computer,” he says. “And that’s significant because if you can do that, even a constant factor speed-up will significantly reduce the time that’s spent doing those simulations and the money that’s spent on compute.”
The key is to hand over some of the underlying components of the testing to Nisq machines, Savoie adds: “Some of the subroutines can have a quantum equivalent that can do these highly complex multivariate analyses quicker and with greater accuracy.”
In a separate experiment, Zapata has worked with Spanish bank BBVA, using quantum versions of Monte Carlo simulations to calculate derivatives prices that incorporate a counterparty risk adjustment—one of six separate applications the bank began exploring last year.
Savoie admits more qubits are needed to scale that particular use case. However, describing its work in July, BBVA’s global head of research and patents, Carlos Kuchkovsky, claimed some quantum applications could be mature enough to use within as little as two years.
As well as testing particular applications, banks can prepare for a quantum future in other ways. Algorithms that run on classical computers need to be rewritten for the radically different physics of a quantum machine. France-based firm QuantFi has trained quants at banks including BNP Paribas to write quantum algorithms that will solve risk management problems such as value-at-risk calculations.
Such sums can be completed in 100 steps on quantum technology, rather than 10,000 steps on a classical computer, claims QuantFi co-founder Paul Hiriart, “but none of the banks we know have decided yet to launch a project for go-live”.
Why? The integration of quantum technology into a bank’s internal systems alone would take at least two years, he says, adding that the pandemic has also had an impact on bank spending priorities: their investment in quantum technology has been postponed by a year in some cases.
Vendor landscape
For banks and investors that just can’t wait to get their hands on some quantum tech, there is an alternative to renting an embryonic machine. Quantum annealers are commercially available today and exploit quantum effects—Canada’s D-Wave Systems is the best-known manufacturer.
They are suited to a far narrower set of use cases than tomorrow’s ‘universal’ quantum computers—primarily optimization problems—but the first services to employ annealers are currently in the works.
US-based start-up Chicago Quantum says it can build an optimal portfolio out of a universe of 60 US listed equities using a D-Wave Systems quantum annealer that performs the task in just 21 seconds.
The firm plans to offer its services to individual investors and professional investment managers. But it admits classical methods can also find the ideal portfolio in under a minute.
Germany-based Zorc Finance is another fintech offering investors the opportunity to construct “minimum-risk highly diversified portfolios” through D-Wave equipment. But, by combining the annealer with classical computing, it is able to analyse more than 2,000 stocks, breaking the large problem into smaller pieces that are solved separately, then recombined.
Zorc Finance co-founder Oscar Javier Hernandez says the calculations arrive at more efficient portfolio solutions and take a few minutes, compared with up to an hour by classical methods alone. The company aims to launch a subscription service next year.
However, not everyone is sold on the advantages.
“Everything that is solvable right now on an annealer can be solved on a laptop in seconds,” says a quantum expert at one investment bank.
“Yes, annealers can be faster, but whether you spend one second customising your portfolio or 10 milliseconds, it doesn’t really matter, especially when you do it through the cloud. The time signal travels from a laptop to the cloud, and then from the cloud back to a laptop. That is probably more than the computational time on a laptop.”
Quantum computing consultants recognize the technology has not yet proven its advantage over the classical alternative – especially in terms of cost.
Quantum costs are “astronomical” compared with cloud-based classical alternatives, says Mike Biercuk, founder and chief executive officer of Australia-based Q-CTRL. A D-Wave annealer might set a firm back $15 million, and few other manufacturers are even offering kit for sale.
Simon Devitt, founder of Australia-based consultant h-bar, concurs: “The banks don’t really care if it’s a quantum computer calculating the stuff or a classical computer. They want the cheapest method possible to calculate the things that they’re interested in.”
That is not deterring bank interest, however. Q-CTRL’s Biercuk tells Risk.net: “A lot of large financial organizations have more or less taken a hedging position, where they say: ‘Well, if it doesn’t work, then it doesn’t work. But if it does work, we want to be there first.’”
The fear factor is present in other ways. As well as preparing for the advantages it may confer, banks must also be ready for what the worst of the quantum computing era may bring: the breaking of traditional public key cryptography through Shor’s algorithm.
Ali El Kaafarani, founder and CEO of post-quantum cryptography firm PQShield, says that, while many are looking forward to quantum computing solving a particular problem, by the time a quantum leap arrives for cyber security, “it’s going to be too late because quantum attacks work retrospectively. Whatever you’re encrypting now over the internet will effectively be broken. People can harvest that data now and decrypt it later when they have access to a quantum computer”.
Trading uses
More than two years ago, sources in the investment community told Risk.net that high-frequency trading (HFT) firms were experimenting with quantum computers.
At the time, those claims could not be substantiated and went unreported. A spokesman for one HFT company said it would not benefit from having a faster problem-solver: “We’re not doing matrix math. All we need to do is process the order book and understand the state of the market, and provide bids and offers. It’s hard to see how an increase in the speed of that is of incredible use until exchanges significantly improve their ability to process things sequentially.”
But more sources now talk of possible applications for quantum computing in investing and trading strategies that identify arbitrage opportunities ahead of other market participants. They say if an arbitrage opportunity was expected to persist for some time, it might justify the cost of building a custom quantum circuit to exploit it.
Henning Dekant, chief executive of Canada-based quantum software developer Artiste-qb, reckons if quantum hardware was installed in a data center next to a trading firm’s server, “if you have a really fast optimization engine, then it may easily justify putting $20 million down to find an arbitrage opportunity half a second earlier than any competitor”.
The banks speaking to Risk.net for this article say they aren’t targeting this type of use case, but admit it is becoming a more realistic application.
Lee Braine, director of research and engineering at Barclays, says the technology may be fit for HFT firms “when you’re able to get results back from quantum computers much faster”.
Japanese bank Nomura’s global chief digital officer, Jezri Mohideen, says he “wouldn’t be surprised” if HFT companies were testing out quantum kit.
And JP Morgan’s head of research and engineering, Marco Pistoia, says: “We haven't looked at an [HFT use case]. But I think it is in the foreseeable future.”
At 1Qbit, director of machine learning and quantum-inspired solutions Jas Oberoi says the firm has been approached by potential clients looking to exploit trading use cases. But he says advantages can only be conferred if the quantum hardware is installed “right on-premise, because if you are relying on cloud services to access hardware, then no matter how fast your internet service, you won’t be able to gain that edge”.
Oberoi tells Risk.net: “Sometimes, firms have proprietary techniques that they use to calculate certain things, look at combinations of metrics and deduce something from the information available. You might need to calculate that every hour or so. And you would rather calculate it using a quantum computer, or some other exotic hardware, and then use that information in your HFT algorithm.”
But he adds that the cost of implementing a quantum strategy would need to bear favorable comparison to building a custom classical chip, which would likely be cheaper.
Further reading
Only users who have a paid subscription or are part of a corporate subscription are able to print or copy content.
To access these options, along with all other subscription benefits, please contact info@waterstechnology.com or view our subscription options here: http://subscriptions.waterstechnology.com/subscribe
You are currently unable to print this content. Please contact info@waterstechnology.com to find out more.
You are currently unable to copy this content. Please contact info@waterstechnology.com to find out more.
Copyright Infopro Digital Limited. All rights reserved.
As outlined in our terms and conditions, https://www.infopro-digital.com/terms-and-conditions/subscriptions/ (point 2.4), printing is limited to a single copy.
If you would like to purchase additional rights please email info@waterstechnology.com
Copyright Infopro Digital Limited. All rights reserved.
You may share this content using our article tools. As outlined in our terms and conditions, https://www.infopro-digital.com/terms-and-conditions/subscriptions/ (clause 2.4), an Authorised User may only make one copy of the materials for their own personal use. You must also comply with the restrictions in clause 2.5.
If you would like to purchase additional rights please email info@waterstechnology.com
More on Data Management
New working group to create open framework for managing rising market data costs
Substantive Research is putting together a working group of market data-consuming firms with the aim of crafting quantitative metrics for market data cost avoidance.
Off-channel messaging (and regulators) still a massive headache for banks
Waters Wrap: Anthony wonders why US regulators are waging a war using fines, while European regulators have chosen a less draconian path.
Back to basics: Data management woes continue for the buy side
Data management platform Fencore helps investment managers resolve symptoms of not having a central data layer.
‘Feature, not a bug’: Bloomberg makes the case for Figi
Bloomberg created the Figi identifier, but ceded all its rights to the Object Management Group 10 years ago. Here, Bloomberg’s Richard Robinson and Steve Meizanis write to dispel what they believe to be misconceptions about Figi and the FDTA.
SS&C builds data mesh to unite acquired platforms
The vendor is using GenAI and APIs as part of the ongoing project.
Aussie asset managers struggle to meet ‘bank-like’ collateral, margin obligations
New margin and collateral requirements imposed by UMR and its regulator, Apra, are forcing buy-side firms to find tools to help.
Where have all the exchange platform providers gone?
The IMD Wrap: Running an exchange is a profitable business. The margins on market data sales alone can be staggering. And since every exchange needs a reliable and efficient exchange technology stack, Max asks why more vendors aren’t diving into this space.
Reading the bones: Citi, BNY, Morgan Stanley invest in AI, alt data, & private markets
Investment arms at large US banks are taken with emerging technologies such as generative AI, alternative and unstructured data, and private markets as they look to partner with, acquire, and invest in leading startups.