SF quant firm uses 'nearest neighbor' machine learning for equities predictions
Creighton AI is using a regression-based approach to machine learning to help make predictions about the excess return of a stock relative to the market.
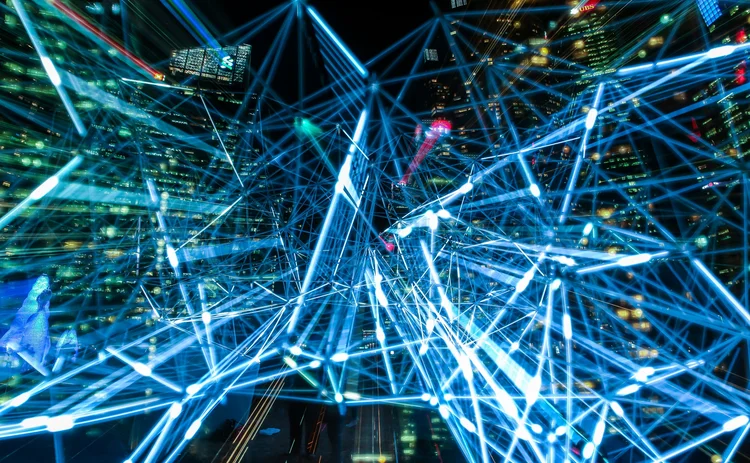
One of the bugbears for quantitative analysts and data scientists working in financial firms is the low signal-to-noise ratio in raw financial data. A lot of the work in this field is the unglamorous task of cleaning data and adjusting standard algorithms to fit financial use cases.
Developing models that can work the randomness and uncertainty associated with financial data has been core to the career of Jim Creighton, founder and chief investment officer at San Francisco-based Creighton AI, which has just under US$200 million in assets under management. Creighton AI is a hedge fund that specializes in using machine learning for systematic global equity long/short and long-only strategies.
“Trying to predict future returns of stocks is a really hard problem, even for machine learning or artificial intelligence,” Creighton tells WatersTechnology.
Creighton says he has friends who work in industries outside of finance, and use machine learning in contexts such as medical diagnostics, oil and gas discovery, and building self-driving cars. He says he jokes with them that their problems are simple to solve: the data they use has relatively high signal and low noise.
Take rocks, for example. It’s difficult to think of anything less mutable than rocks. “If you look at geological data, it does not change for millions of years—this is a very stable problem. When you start looking at financial data, it’s extremely noisy. You are searching for a little signal in this ocean of noise, and the fundamental conditions are changing all the time,” Creighton says.
Creighton, who has held senior investment roles at Barclays Global Investors, Deutsche Asset Management, and Northern Trust Asset Management, started his own fund in 2004. Initially, it did not use machine learning and focused on a set of unique factors to make stock-related predictions using regression-based methods. “Frankly, that did not work as well as I had hoped,” Creighton says.
The fund then started looking at rudimentary forms of machine learning. It first tried decision trees, which Creighton says gave better results, but fell short of what he thought could be achieved. After experimenting with different forms of machine learning, it settled on a customized version of a K-nearest neighbors (KNN) clustering strategy as its primary methodology. KNN can be used for both classification and regression methods of machine learning. Creighton AI takes a supervised regression approach to machine learning with KNN.
Creighton says KNN works much better than decision trees and random forests for their fund’s use case. “One of the nice attributes of KNN is that it is not a black box, you can really understand what it’s doing and why it’s making these decisions,” he says.
Reducing noise, enhancing signal
Creighton AI’s data scientists are trying to predict excess returns of a stock relative to a market; for example, the return on a British bank relative to the UK stock market.
“We would look at the excess return of [the bank] relative to the FTSE index. It is that excess return that we are trying to predict. What we are interested in knowing is which stocks are going to outperform the market, and which are going to underperform,” he says.
KNN methodologies can be used to predict a future value by examining similar use cases from historical data sets. These similar use cases are referred to as the “nearest neighbors”. In a standard KNN approach, there is a minimum threshold to how alike the historical use cases and the predicted use case must be, and the similarities must meet a set of parameters before the prediction process can occur. “In problems where you build high-signal, low-noise, that works okay. But in financial data, for a whole host of reasons, it doesn’t. One of the things we have done is we have created a radius—in other words, how far it will search from the point you are trying to predict—a variable. And that variable depends on a lot of things,” Creighton says.
For example, if an analyst is making a prediction for a stock in the US and there are millions of historical cases, there should be a vast number of ‘nearest neighbors’, within the defined radius, for making a prediction. If the same methodology was applied in a different geographic setting, there might be only 20 similar historical cases, and the analyst may have to look a lot of further back across the historical database.
“If you go too far away, the historical cases are not similar enough to be useful. You are introducing more noise than signal,” Creighton says.
Creighton AI uses data from Refinitiv, including historical prices of stocks, corporate actions, and the size of companies. The raw data is transformed, meaning the signal is enhanced and noise suppressed before it is used for machine learning.
One example of noisy data that needs to be removed, could be if a small energy explorer in the US makes a major discovery of a new oil field, Creighton says, and the stock will “pop a lot” on that announcement. It could go up as much as 50%, even if oil and gas prices and the share prices of energy companies generally are going down. Another example would be a small pharmaceutical company coming up with a successful vaccine for Covid, with the company’s stock going up a lot, which could be completely contrary to what is going on more generally in the field. Financial market data can’t predict idiosyncratic events like the discovery of oil fields or vaccines.
“If you don’t suppress that 50% move of the stock, the machine will have a data point that is misleading, not enlightening,” Creighton says.
Two-stage machine learning
Creighton AI is getting close to introducing a second form of machine learning at a different stage of the predictive process, as it wants better prediction accuracy. For that, it is looking at two different methodologies. One is a derivative of a support vector machine, a machine learning method that Creighton says can offer an “optimal separation” between stocks expected to outperform the market on one side and stocks that should underperform on the other side. The fund is also exploring the possibility of adding a neural network to the prediction process.
Creighton says he hasn’t yet decided which form to go with, or which part of the predictive process they will be applied to. “We are getting good results in both. We will decide some time over the next six months, and then we will have a two-stage application of machine learning to the prediction process. The first stage will continue to use clustering [KNN], the second stage is going to use one of these alternative techniques,” Creighton says.
Creighton says the fund measures prediction accuracy in every country where it makes predictions in financial markets.
The fund is currently looking at 14 factors to predict the movement of the price of a stock and plans to add more. A simple example of a factor the fund looks at is the size of a company. Creighton says investors treat big companies differently than small companies; using a small pharmaceutical firm to predict the performance of a large bank would not be very useful.
Creighton says the firm will probably never use more than 20 factors, as he is skeptical of models that employ too many. Most quant managers, he says, use factor playbooks with at least 50 or 60 factors—sometimes even more. Creighton believes that factors have diminishing returns on the quality of prediction accuracy. When considering what factors could explain why a stock’s price varies from day to day, the first factor to consider would be the market itself, which Creighton says could probably explain 50% of the price movement. The second factor might be related to the industry the stock belongs to, which would be less important.
“By the time you get down to the 14th explanatory variable for why a stock’s price is moving, the amount of variability it is explaining is going to be one or two percent. It is really at the margin. The 20th factor will explain maybe a half percent of the movement,” he says.
Factors that are on the margin are not that important, Creighton says, and complicate things without a satisfactory improvement in prediction accuracy.
Creighton says developing and adopting the fund’s machine learning model is a task that will never end. “Every time we learn something new and incorporate it, that opens up another door where we learn more new things. And so we are continually building on the knowledge we are developing,” he says.
Further reading
Only users who have a paid subscription or are part of a corporate subscription are able to print or copy content.
To access these options, along with all other subscription benefits, please contact info@waterstechnology.com or view our subscription options here: http://subscriptions.waterstechnology.com/subscribe
You are currently unable to print this content. Please contact info@waterstechnology.com to find out more.
You are currently unable to copy this content. Please contact info@waterstechnology.com to find out more.
Copyright Infopro Digital Limited. All rights reserved.
As outlined in our terms and conditions, https://www.infopro-digital.com/terms-and-conditions/subscriptions/ (point 2.4), printing is limited to a single copy.
If you would like to purchase additional rights please email info@waterstechnology.com
Copyright Infopro Digital Limited. All rights reserved.
You may share this content using our article tools. As outlined in our terms and conditions, https://www.infopro-digital.com/terms-and-conditions/subscriptions/ (clause 2.4), an Authorised User may only make one copy of the materials for their own personal use. You must also comply with the restrictions in clause 2.5.
If you would like to purchase additional rights please email info@waterstechnology.com
More on Emerging Technologies
This Week: Startup Skyfire launches payment network for AI agents; State Street; SteelEye and more
A summary of the latest financial technology news.
Waters Wavelength Podcast: Standard Chartered’s Brian O’Neill
Brian O’Neill from Standard Chartered joins the podcast to discuss cloud strategy, costs, and resiliency.
SS&C builds data mesh to unite acquired platforms
The vendor is using GenAI and APIs as part of the ongoing project.
Chevron’s absence leaves questions for elusive AI regulation in US
The US Supreme Court’s decision to overturn the Chevron deference presents unique considerations for potential AI rules.
Reading the bones: Citi, BNY, Morgan Stanley invest in AI, alt data, & private markets
Investment arms at large US banks are taken with emerging technologies such as generative AI, alternative and unstructured data, and private markets as they look to partner with, acquire, and invest in leading startups.
Startup helps buy-side firms retain ‘control’ over analytics
ExeQution Analytics provides a structured and flexible analytics framework based on the q programming language that can be integrated with kdb+ platforms.
The IMD Wrap: With Bloomberg’s headset app, you’ll never look at data the same way again
Max recently wrote about new developments being added to Bloomberg Pro for Vision. Today he gives a more personal perspective on the new technology.
LSEG unveils Workspace Teams, other products of Microsoft deal
The exchange revealed new developments in the ongoing Workspace/Teams collaboration as it works with Big Tech to improve trader workflows.