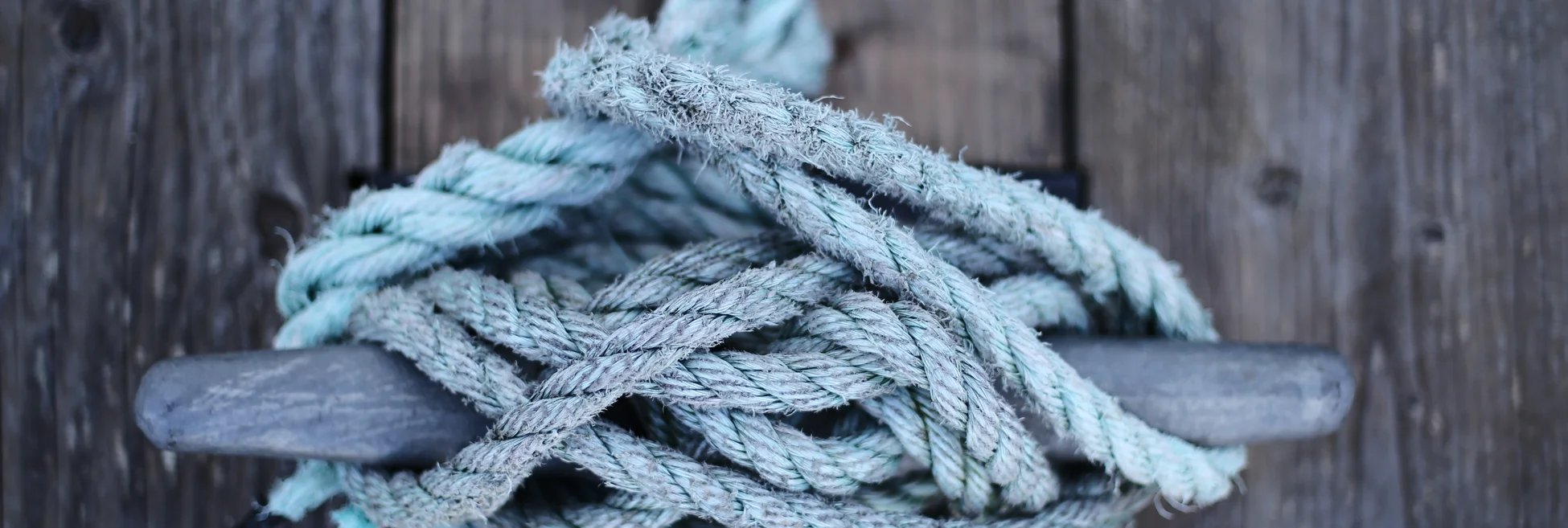
The AI Ethics Dilemma: Banks Find a Fine Line Between New Tech and 'What's Right'
Finance firms and regulators are beginning to assess the ethical implications of artificial intelligence.
While more than a half-century old, artificial intelligence (AI) is still an emerging field in finance, and with its growth have come concerns about the ethical implications of what is essentially the outsourcing of decision-making to machines.
Banks have always worried that the data they feed to their algorithms is not of sufficient quantity and quality to run effective models; this is a difficult but established problem. Now, though, the industry must deal with the far more nebulous problem of the socioeconomic ramifications of data—and get there before the regulators do.
“Folks are just beginning to recognize that even if you have great data, when you put it into these new technologies you have to interrogate the outcomes to understand how your decisions are affecting the socioeconomic or cultural situation,” says Diana Ascher, director of the Information Studies Research Lab at UCLA.
Ascher is also the founder of the Information Ethics and Equity Institute under the Enterprise Data Management (EDM) Council. The EDM Council is one of the many organizations starting to think about data ethics, along with regulators, trade associations, and banks themselves. The Royal Bank of Canada (RBC) and Dutch bank ING are among an increasing number of firms with initiatives formally considering data ethics. The European Commission (EC) formed a high-level expert group on the topic and consulted on “ethics guidelines for trustworthy AI” to guide European companies. Last December, the Monetary Authority of Singapore (MAS) put out its own set of principles.
Clearly, the industry is worried about understanding the machines.
Systemic Bias
Currently, the majority of AI in financial institutions is limited to relatively simple techniques, from robotic process automation (RPA) to low-level decision trees and basic linear regressions. But firms are innovating more and more in the quest for more sophisticated uses in risk management and forecasting. As these technologies become more advanced—such as deep neural networks—not only their inner workings but also their outcomes become more complicated to understand, and the stakes become higher.
Data management is a federated responsibility around the organization. And I would put ethics right into that model.
John Bottega, EDM Council
Industry bodies say their members are currently most worried about the implications of AI that assess creditworthiness and lending, says John Bottega, an executive director at the EDM Council.
“If an organization in the capital markets or retail sector is looking to sell their products, they want to understand the creditworthiness of their customers or where they have the opportunity to sell products. The typical input into that that kind of algorithm would be FICO [credit] scores, looking at levels of education, historical buying patterns and things of that nature.”
This is all good, clean, accurate data, says Bottega, but “what is happening in these examples is that the outcome of that analytic was saying, ‘This part of town is ideal for selling these products, this part of town isn’t.’ And that implies that we have gone back to redlining—we are now basing decisions on socioeconomic, racial divides.”
This is not a theoretical worry; there is real evidence that this kind of discrimination exists in markets. A research paper jointly written by academics at several US universities and published in 2018 shows how historically black colleges in the US find it harder to raise money in the secondary markets, as they pay higher underwriting fees to issue tax-exempt bonds compared to colleges that were not historically black. The researchers found that credit quality played no role, as these colleges were AAA-rated and insured. Bonds issued by historically black colleges were more expensive to trade and when they did, sat in dealer inventory longer.
The research paper doesn’t mention AI. But this is the kind of inequity that could be perpetuated, completely unwittingly, by financial institutions plugging historical data into a machine-learning model without interrogating either the data going in, or the data coming out. And apart from the social impact, this kind of discrimination is a blind spot for a bank that could cause it to miss out on untapped or underserviced markets.
Horns of a Dilemma
Underlying ethics considerations is a major dilemma: When do you make the inevitable trade-off between the amazing products or tools you can create with huge datasets combined with AI, and the ethics of the products or tools? Where is that line and when is it crossed?
The creepy line is when you approach a customer with some kind of marketing or offering and the customer feels uneasy with that.
Umar Latif, ING
Umar Latif, head of data governance at ING, says his firm takes a customer-centric view, and customers’ comfort is its yardstick. ING could build innovative products with the data it has on its customers that would be completely legal from, say, a General Data Protection Regulation (GDPR) standpoint, but would not necessarily be ethical. At some point, customers or stakeholders will become uncomfortable with how their data is being used. ING calls that point of discomfort the “creepy line,” Latif says.
“Where things get tricky is where we approach the creepy line for our customer, or any other stakeholder. We have data about ratings of customers or maybe transactional data about them—we can do a lot with that. But we need to be careful that we do it in an ethical manner, that we don’t intrude on the ethical rights of our stakeholders,” he says. “The creepy line is when you approach a customer with some kind of marketing or offering and the customer feels uneasy with that: ‘OK, you have my data and you are probably allowed to approach me in that manner, but am I comfortable with you doing that?’”
Latif says ING tells its staff to be responsible when using data and consider doing the right thing for people and society. “We ask: ‘Could you explain to family and friends and even elders what you are doing with this data?’” Latif says. “The data usage needs to be ethical. These are the key components we use from a values-based perspective.”
Leveraging the CDO
From an enterprise standpoint, data ethics is a responsibility that should reside in the C-suite—but exactly with whom, and how the values or standards developed at the top permeate down into the bank, are topics that are beginning to be discussed.
Regulators have begun to define where the responsibility for ethical data and AI lies. The EC’s guidelines for trustworthy AI, for example, include suggestions for governance frameworks, including the appointment of a person in charge of AI ethics, or an internal or external ethics panel or board that could provide oversight or advice. The MAS’s principles illustrate a number of possible frameworks for internal and external accountability.
David Ostojitsch, technology and operations committee director at the Association for Financial Markets in Europe (AFME), says individual organizations will figure out what works best for them. “All banks have different business models, product lines and organization structures. Some are cross-border. Everyone needs to look at it in their own way. Understanding its use needs to permeate from the board down in appropriate ways. But who exactly is responsible will be a mix guided by what people think is the right model,” he says.
The kind of structures and regulation of boards to facilitate this are already in place, like the Senior Managers Regime in the UK, he adds.
The EDM Council’s Bottega says that exactly who will take responsibility for data ethics is still an evolving consideration within financial firms, but from his perspective, one possibility is to leverage the model of the chief data officer (CDO).
Bottega himself was CDO of both Bank of America and the Federal Reserve Bank of New York. He says one of the reasons the CDO role came into being was that while there were individuals with responsibility for technology, there was no individual responsible for the use of information. But the CDO was not ultimately responsible for the implementation of the data—that included business and technology people within the enterprise.
“Data management is a federated responsibility around the organization. And I would put ethics right into that model,” Bottega says.
While the CDO can be a champion as an executive in the C-suite and bring awareness, understanding, education and some type of policy or guidelines for the organization, “the accountability falls to everybody in the organization: the people running the models, the people running the businesses, the marketing teams that sell the products—everybody is involved,” Bottega says.
ING has tried to build its own data ethics model around this idea. ING’s framework, which it began to develop in 2016 and has been operational since last year, is founded on the bank’s existing corporate culture.
“The primary responsibly for data ethics lies with each and every employee within ING. We rolled out our data ethics framework as something that is clearly linked to our organizational culture,” Latif says. “We have values and principles and these tie into data ethics as well. We translated our corporate code into these data ethical values and principles.”
In practice, the framework rests on data ethics councils in ING’s regional banks all over the world. When an employee in one of these branches encounters an ethical dilemma in the course of their work, perhaps while developing new marketing practices, algorithms or products, they send it to their relevant council. These are collected in a repository in the central data ethics council in the banks’ Netherlands headquarters, says Carmen Gomez, a data governance specialist at ING and coordinator of the data ethics council.
“We have a data ethics council in each country. And next to that, we have one global data ethics council where we safeguard the data ethics framework and collect and assess all the ethical dilemmas that have been discussed in the councils,” Gomez says. “We collect them to create consistency and to make sure we are aligned.”
The councils are intended to include a diverse array of people. “In each country where these councils are established, we have people from different areas involved,” Gomez adds. “So it’s not only architects, not only IT people or data management people. We have people from sustainability, we have people from legal, from compliance, people from AI and from the business itself. Together they advise on an ethical issue that an employee or department can have.”
Regulatory Balancing Act
Firms and industry bodies see this as a good time to get in ahead of the regulators and be part of the conversation as it develops. A major concern for banks is that while there are obviously ethical issues at stake, overly prescriptive regulation will stifle innovation.
AFME says in its consultation response on the EC’s guidelines for trustworthy AI that “too quickly prescribing formal requirements and assessment criteria may fail to capture, or limit the maturity and continued adoption of AI.”
The EC’s consultation had the right focus and the Commission is moving early on this topic, which is a good thing, Ostojitsch says. “But we found that the guidelines could be quite restrictive if they were implemented, certainly in the earlier versions,” he adds.
In the consultation paper, Ostojitsch lays out some concerns that the industry body had, such as with the EC’s assertion that the more autonomy given to an AI system, the more extensive testing and stricter governance is required.
You want to have folks that can look at an algorithm and say, ‘I wonder what is going to happen to this population if we use this particular proxy variable to make the decision.
Diana Ascher, UCLA
Greater human oversight might be appropriate for systems that interact directly with humans, rather than by the AI system’s overall level of autonomy, the paper says.
Ostojitsch explains: “If, for example, you are using AI for something that a bank has determined is very low risk—it doesn’t touch any counterparties, it doesn’t touch any clients, it has got very limited internal focus—then in essence you should be able to have limited human oversight on it, even if that AI was automated.”
Another issue AFME had with the EC’s conceptualizing of the guidelines was its idea of explainability, which is the ability to explain the technical processes of an AI system and the related human decisions. Explainability has its limits, Ostojitsch says.
“The simpler uses of AI are very explainable. However, when you start getting to more sophisticated uses, or when you start to be more innovative, that is where explainability gets more difficult,” he says. “If an explanation is required at a detailed level for every use of AI, that could put the brakes on how these technologies develop over the next few years.”
Neural networks, for example, he says, are essentially black boxes when it comes to interpreting data and making decisions.
“To try and provide an explanation of even a basic neural network is very complicated because of all the different factors that the neural network might be considering,” he says. “You could also argue in some cases, like the use of AI in catching financial crime or anti-money laundering, the less that’s known about the inner workings of that AI the better. Otherwise, people would be able to circumnavigate those rules.”
Ostojitsch says the MAS’s principles—formally named the Principles to Promote Fairness, Ethics, Accountability and Transparency in the use of AI and data analytics in Singapore’s financial sector—are the kind of non-prescriptive, principles-based guidelines that the financial industry would like to see.
Actual rules and regulations of AI driven by ethical concerns are a long way off, and there are other regulations that touch on these issues that are in place already, whether data privacy laws or governance standards like BCBS 239. But there are many measures banks can take now to future-proof against reputational and regulatory risk.
They can start ethics committees and working groups, like ING has. They can promote the awareness at every level that decisions can have far-flung consequences, and run that education program under the auspices of the CDO’s office.
And, perhaps most importantly, they can build diversity into their organizations. Diverse experiences and opinions within the company make it easier to understand the ripple effects of an algorithm’s decision, says the UCLA’s Ascher. “You want to have folks that can look at an algorithm and say, ‘I wonder what is going to happen to this population if we use this particular proxy variable to make the decision,’” she says. “Getting these different perspectives is essential.”
Further reading
Only users who have a paid subscription or are part of a corporate subscription are able to print or copy content.
To access these options, along with all other subscription benefits, please contact info@waterstechnology.com or view our subscription options here: http://subscriptions.waterstechnology.com/subscribe
You are currently unable to print this content. Please contact info@waterstechnology.com to find out more.
You are currently unable to copy this content. Please contact info@waterstechnology.com to find out more.
Copyright Infopro Digital Limited. All rights reserved.
As outlined in our terms and conditions, https://www.infopro-digital.com/terms-and-conditions/subscriptions/ (point 2.4), printing is limited to a single copy.
If you would like to purchase additional rights please email info@waterstechnology.com
Copyright Infopro Digital Limited. All rights reserved.
You may share this content using our article tools. As outlined in our terms and conditions, https://www.infopro-digital.com/terms-and-conditions/subscriptions/ (clause 2.4), an Authorised User may only make one copy of the materials for their own personal use. You must also comply with the restrictions in clause 2.5.
If you would like to purchase additional rights please email info@waterstechnology.com
More on Emerging Technologies
This Week: Startup Skyfire launches payment network for AI agents; State Street; SteelEye and more
A summary of the latest financial technology news.
Waters Wavelength Podcast: Standard Chartered’s Brian O’Neill
Brian O’Neill from Standard Chartered joins the podcast to discuss cloud strategy, costs, and resiliency.
SS&C builds data mesh to unite acquired platforms
The vendor is using GenAI and APIs as part of the ongoing project.
Chevron’s absence leaves questions for elusive AI regulation in US
The US Supreme Court’s decision to overturn the Chevron deference presents unique considerations for potential AI rules.
Reading the bones: Citi, BNY, Morgan Stanley invest in AI, alt data, & private markets
Investment arms at large US banks are taken with emerging technologies such as generative AI, alternative and unstructured data, and private markets as they look to partner with, acquire, and invest in leading startups.
Startup helps buy-side firms retain ‘control’ over analytics
ExeQution Analytics provides a structured and flexible analytics framework based on the q programming language that can be integrated with kdb+ platforms.
The IMD Wrap: With Bloomberg’s headset app, you’ll never look at data the same way again
Max recently wrote about new developments being added to Bloomberg Pro for Vision. Today he gives a more personal perspective on the new technology.
LSEG unveils Workspace Teams, other products of Microsoft deal
The exchange revealed new developments in the ongoing Workspace/Teams collaboration as it works with Big Tech to improve trader workflows.