The haves and the ‘have bots’: can AI give vol forecasters an edge?
Quants at Acadian, Man Group, Northern Trust and PGIM are investigating the use of machine learning and natural language processing to improve volatility forecasts.
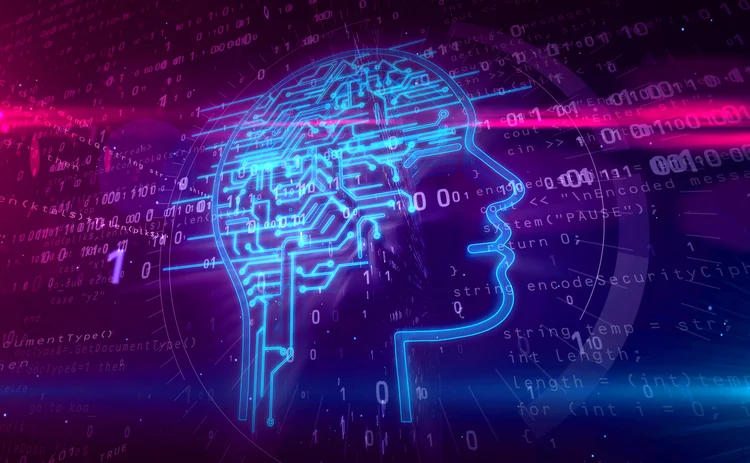
Need to know
- Man Group says it improved the accuracy of short-term forecasting models by 20–30% in some cases.
- Quants believe that standard approaches fail to account for exogenous macro shocks and can miss non-linearities in vol.
- More exact estimates of vol can help firms to size positions better. “It’s essential for any risk management system,” says Man’s Stefan Zohren.
Nearly three months after its launch, ChatGPT is still generating a flurry of headlines. The artificial intelligence chatbot—whose answers to complex questions are almost indistinguishable from those offered by humans—is prompting a range of professionals to wonder, rightly or wrongly, if the underlying technology will make some of their work redundant. And among those who might be considering what ChatGPT can do for them are quants—particularly in the area of volatility forecasting.
Man Group, the hedge fund with $142 billion of assets under management, has spent years looking at how AI might be incorporated into vol forecasting. Its research has involved tapping into high frequency data, natural language processing (NLP) and ‘deep learning’, a form of machine learning that teaches computers to replicate human thinking.
Stefan Zohren, principal quant in the firm’s central trading team in London and a research fellow at the Oxford-Man Institute of Finance, says the work has supercharged the accuracy of some of its vol forecasts.
“One measure to consider is the loss function used in the forecasting model,” he says. “There, improvements can be quite large just by adding high frequency data. Then, if you add some modern deep learning techniques… the accuracy you had before can be further improved by some 20–30%.” The loss function is a measure of the model’s accuracy.
In the old models, it was only about historical prices… Using AI is much more about conditioning on actual events within the markets and economy
Michael Hunstad, Northern Trust Asset Management
Proponents of using AI in vol forecasting say standard methodologies—such as generalised autoregressive conditional heteroskedasticity (Garch) and its variants—only consider past volatility. They are known as autoregressive because past behaviour is used to forecast future behaviour. By assuming that what has gone before offers a reliable guide to what is yet to come, and by not taking into account external factors, these models can miss non-linear patterns in how volatility unfolds.
Michael Hunstad, chief investment officer for global equities at Northern Trust Asset Management, has been watching the developments closely. He points to an increased spikiness in volatility in the equity space over the past 18 months, which he attributes to macroeconomic factors. Volatility has jumped and then subsided, he says; the shocks have not been predictive of what has followed; and the old models do not take into account the kinds of macroeconomic events that have prompted these spikes.
“In the old models, it was only about historical prices, basically looking at what happened yesterday, the day before yesterday and so on,” he says. “You’d then try to figure out how that influences today. Using AI is much more about conditioning on actual events within the markets and economy. We know there are exogenous forces, such as macroeconomic events, that have an influence on volatility clustering. But we don’t have truly effective ways to identify and specify the influence of these types of events. AI can help with both.”
Acadian Asset Management, which manages more than $100 billion in assets, also believes that AI could help investors to incorporate the kinds of influences that today’s volatility models fail to consider. Vladimir Zdorovtsov, director of global equity research at the quant shop, says the current models have a glaring weakness: their failure to take into account the way risk is propagated in inherently non-linear ways, with different risk drivers interacting with and amplifying each other.
“A boat that stays afloat with five occupants can suddenly sink when another boards,” he says. “Weather conditions or a particularly unruly passenger rocking a boat can cause it to capsize, even with a lighter load. These complex interactions are present in financial risks as well, and most conventional approaches tend to assume them away.
“Just as Hurricane Katrina showed that claims on flood, health, car and other insurance policies are far from independent during tail events, so did the global financial crisis with a host of debt obligations. Existing risk modelling approaches attempt to tackle these intricate nuances piecemeal—incorporating those effects which one is aware of, usually based on ‘lessons learned’ the hard way.”
Given the state of the markets over the past year, tail events are at the forefront of investors’ minds. With Russia’s invasion of Ukraine, eye-watering inflation, interest rate hikes, supply chains in disarray and social discontent across the globe, 2022 was a choppy year—the repercussions of which will continue to play out over the coming months.
The 2023 JP Morgan E-Trading Edit, which surveys 835 institutional traders, found volatility had overtaken liquidity as the primary worry for the first time. In such circumstances, accurate vol forecasts are urgently required.
The world according to Garch
Vol forecasting has historically been performed using Garch models and their variants, which were developed in the 1980s by US economist Robert Engle. These models capture certain features of volatility observed in financial markets, including heteroskedasticity (the tendency of vol to cluster, so that high vol is often followed by more high vol, and low vol by more low vol).
Heterogeneous autoregressive (HAR) models are another popular framework. HAR-RV models use realised variances instead of moving averages and build simple regression models on them, such as linear regression models. The variances can be used as inputs over different time horizons, such as weekly or daily, and the models can be extended to incorporate higher frequency data.
Vol forecasting is a key component of pricing instruments and of estimating correlations between instruments. It also feeds into vol targeting, where asset managers might adjust exposure to risk—if volatilities are high, positions might be downscaled, and vice versa. The more accurate the estimates of vol, “the better volatility targeting can be performed,” says Man Group’s Zohren. “It’s essential for any risk management system.”
Models that forecast volatility over days or weeks use daily data. AI does not work when data is sparse, and Man Group says that for models operating over horizons longer than a few weeks it has not been possible to generate forecasts better than those provided by the old models. For high-frequency volatility models, though, the firm says improvements are possible. Man Group found one to five minutes to be the best interval between measurements taken of the market; the firm says the data can be added as an input to models that aggregate forecasts in order to generate better daily forecasts. Traditionally, when making a daily prediction of tomorrow’s volatility using only daily data, much of the intraday price fluctuations are excluded.
“[With the higher-frequency data] you can do quite a bit more in terms of using modern techniques,” Zohren adds. “Deep learning models or other modern models become more amenable and easier to apply.”
Dilated causal convolutions—the deep neural networks used in WaveNet, the architecture invented by Google to generate realistic synthetic sound—are one such model. These supercharged versions of existing models read and combine trends in volatility over different frequencies—daily, weekly or monthly.
In existing models, quants code in these look-back periods manually. However, dilated causal convolutions perform as if multiple models were stacked on top of each other. They first consider the fastest frequency patterns; features in the data beyond this frequency are fed into another layer within the model to read the next frequency, and so on. This enables the model to learn patterns over a wide range of frequencies.
Only connections
Another AI-based indicator used as an input to Man Group’s volatility models is the autoencoder reconstruction ratio (ARR). This uses an artificial neural network called an autoencoder, as well as covariance, to in effect measure the connectedness of the market.
As we’ve seen before, if you use a risk model that is based on your past data, you might miss a lot
Marco Aiolfi, PGIM Quantitative Solutions
The auto-encoder learns how to ‘reconstruct’ the hypothetical returns of a large number of financial instruments using a small number of latent variables. If markets are more connected and moving more in sync, it is easier to reconstruct the returns because they are mostly explained by a dominant market move and a small number of other factors. However, as markets become less connected, it becomes harder to reconstruct the original returns.
The accuracy of the auto-encoder in reconstructing returns can be used as an indicator of market stability. A small loss, meaning high levels of accuracy in reconstruction, implies markets are more connected and thus riskier; a larger loss, meaning ‘less good’ reconstruction, implies more stable and less connected markets.
“When markets become more connected, the market is more fragile and you’re more likely to see spikes in volatility because everything moves in sync,” Zohren says. “Whereas if markets are more diverse and less correlated, then those spikes are less likely to occur.”
Northern Trust’s defensive equity strategies are designed to avoid volatility, and specifically the spikes observed over the past year. Hunstad says there are lots of vol characteristics that are not included in standard models: “You can have all sorts of different things, theoretically involved in a Garch framework—many of which are best identified through a machine learning or AI approach.”
Discontinuities are one example of this. It may be that vol does not cluster in equities until interest rates are above 1%; whereas if they are below 1%, nothing is observed. Hunstad says this is a “very different” kind of variable to the ones traditional regression or econometric models usually look at.
Another example is piecewise linearity. If interest rates are between, say, 0% and 1%, there may be no vol spikes; at between 1% and 4%, the level of spikes may be moderate; and at between 4% and 10%, the level of spikes may be extreme. “That kind of impact could be very real, but you’re never going to pick that up through just a traditional approach,” Hunstad adds.
Quants can train machine learning models to learn the correct functional form—the mathematical representation of these kinds of non-linear patterns that occur in volatility. The new maths can then be incorporated into more conventional models, though Northern Trust has yet to try these approaches in live trading.
Mind your language
NLP has become something of a buzzword in markets, and its proponents say it holds much promise in the area of vol forecasting.
Marco Aiolfi, co-head of the multi-asset team at PGIM Quantitative Solutions, says that existing data-driven approaches to vol forecasting—including implied volatility approaches—tend to be backward-looking. This, he believes, can be a huge risk.
“As we’ve seen before, if you use a risk model that is based on your past data, you might miss a lot,” he says. Aiolfi cites the example of Switzerland’s currency: “In early 2015, the Swiss National Bank removed the peg of the Swiss franc versus the euro, and that created havoc in the market and volatility went to unprecedented levels. Your forecasting model would have no chance to incorporate anything like that. And even if you have the ability of incorporating information from implied volatilities, again, you would have been late.”
One way to tackle the problem is to have a framework that looks to model the risks in the economy. This is where NLP comes in.
“In our context, we are looking at quantifying information that tells us something about the state of the economy, and we’re trying to triangulate that from different perspectives,” says Aiolfi. “So one perspective is ‘what are the central banks doing?’—looking at the words in their statement, and the speeches. This tells us something about potential behaviour or biases of the central banks towards growth and inflation.” These perspectives are then incorporated in the firm’s risk models alongside traditional vol forecasts.
Acadian is also using NLP for vol forecasting. Zdorovtsov says advances in machine learning and NLP can help identify and assess the complex interplay between the various drivers of risk: “ML can help find new or refine existing drivers of expected risk by gleaning relevant insights from features which don’t lend themselves well to typical quantitative techniques—for processing text or other non-numeric data to render it into metrics relevant for future risk outcomes.”
It's a model and it’s looking good
Although some firms have reported success in applying AI to vol forecasting, others have hit a wall. “We tried machine learning for estimating volatilities but so far nothing beats our existing model,” says an executive at one large US asset manager. “Sometimes the compromise is to have the volatility estimation not perhaps using the most optimal parameters, because they may introduce too much turnover [the percentage of a portfolio that is sold per month or year]. There’s a trade-off between turnover and the best models. So far, the traditional models work best because they really capture the structure of volatility well.”
Others are more positive about AI’s potential.
“Given the exponential rise in the amounts of relevant data and computing power, as well as continued steady improvements—and a number of significant breakthroughs—in the modelling techniques, it is no surprise that data science has started to play an increasing role in a number of fields,” says Acadian’s Zdorovtsov. “Any field of human inquiry has followed a steady march from eminence-based to evidence-based approaches. Data science at its core is turning input data into information, knowledge and prescriptive insights.”
Man Group currently uses multiple approaches from across the spectrum of available vol forecasting tools, from simple statistical learning models through to modern ML and deep learning models. Its next foray will involve efforts to construct ML models that will pay special attention to specific points in the past, and thereby learn long-term dependencies.
“The problem is, with many of these models, they are only ever able to look back a short period of time,” says Zohren. “So they have already long forgotten about the crisis in 2008, because they only pay attention to things like over the last month.”
Meanwhile, Northern Trust’s Hunstad is excitedly watching the progress that is being made in academia on incorporating AI into vol forecasting: “I think there’s a lot of very, very interesting things from a data perspective, but also from a methodology perspective, that hopefully will lead to some much more accurate forecasts.”
Only users who have a paid subscription or are part of a corporate subscription are able to print or copy content.
To access these options, along with all other subscription benefits, please contact info@waterstechnology.com or view our subscription options here: http://subscriptions.waterstechnology.com/subscribe
You are currently unable to print this content. Please contact info@waterstechnology.com to find out more.
You are currently unable to copy this content. Please contact info@waterstechnology.com to find out more.
Copyright Infopro Digital Limited. All rights reserved.
As outlined in our terms and conditions, https://www.infopro-digital.com/terms-and-conditions/subscriptions/ (point 2.4), printing is limited to a single copy.
If you would like to purchase additional rights please email info@waterstechnology.com
Copyright Infopro Digital Limited. All rights reserved.
You may share this content using our article tools. As outlined in our terms and conditions, https://www.infopro-digital.com/terms-and-conditions/subscriptions/ (clause 2.4), an Authorised User may only make one copy of the materials for their own personal use. You must also comply with the restrictions in clause 2.5.
If you would like to purchase additional rights please email info@waterstechnology.com
More on Emerging Technologies
This Week: Startup Skyfire launches payment network for AI agents; State Street; SteelEye and more
A summary of the latest financial technology news.
Waters Wavelength Podcast: Standard Chartered’s Brian O’Neill
Brian O’Neill from Standard Chartered joins the podcast to discuss cloud strategy, costs, and resiliency.
SS&C builds data mesh to unite acquired platforms
The vendor is using GenAI and APIs as part of the ongoing project.
Chevron’s absence leaves questions for elusive AI regulation in US
The US Supreme Court’s decision to overturn the Chevron deference presents unique considerations for potential AI rules.
Reading the bones: Citi, BNY, Morgan Stanley invest in AI, alt data, & private markets
Investment arms at large US banks are taken with emerging technologies such as generative AI, alternative and unstructured data, and private markets as they look to partner with, acquire, and invest in leading startups.
Startup helps buy-side firms retain ‘control’ over analytics
ExeQution Analytics provides a structured and flexible analytics framework based on the q programming language that can be integrated with kdb+ platforms.
The IMD Wrap: With Bloomberg’s headset app, you’ll never look at data the same way again
Max recently wrote about new developments being added to Bloomberg Pro for Vision. Today he gives a more personal perspective on the new technology.
LSEG unveils Workspace Teams, other products of Microsoft deal
The exchange revealed new developments in the ongoing Workspace/Teams collaboration as it works with Big Tech to improve trader workflows.