The IMD Wrap: Discoverability and visibility, the new challenge of alt data overload
There’s a novel kind of data tsunami on the horizon: the volume of new datasets, and trying to discern what’s out there—and how much of it is useful.
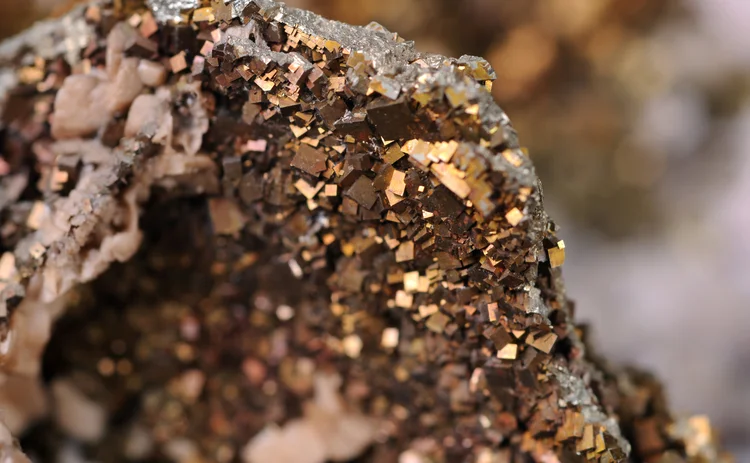
“There’s a data tsunami coming! We’re all going to drown!” How many times have we heard this warning over the years? And usually, it was a warning about capacity: the ability of firms’ servers and systems, and network providers, to carry and store and process the ever-increasing volumes of market data from exchanges.
Decimalization, volatility, high-frequency trading, algorithms that can generate actions faster and more voluminous than a human, not to mention “flash crashes”—all of these things contributed to a rising tide of data. And while technical innovations such as data compression, and factors like cheap and readily available bandwidth have solved some of these challenges, the sheer volume of data available still remains.
In some cases, that challenge remains technical in nature: checking for upgrades, optimized capacity, and monitoring your service-level agreements to ensure peak performance. This is especially true in the case of “traditional” market data—exchange prices, and the like. If an exchange experiences drastically higher volumes, the amount of data generated may overwhelm those who are unprepared. It may slow down their ability to process it and respond in a timely manner.
They may miss trade opportunities—or worse, execute late at unfavorable prices. But for the most part, that’s not an issue—and if it is, it’s a technical issue: buy a newer server, upgrade your network capacity. And besides, it’s not like you’re being overwhelmed by multiple different data sources: an exchange is still an exchange; its data is still one exchange’s data. We aren’t being overwhelmed by new exchanges popping up every week.
But outside the clean, corporate realm of traditional data, in the grimy, gold-rush frontier town of alternative data, the deluge is real, and the problem is twofold: not only must the consumers of this data among traders and—more often than not—data scientists make sense of it and extract insight from it; they also—just like the grizzled prospectors of the Yukon Trail—have to find it and get it out of the ground.
‘There’s gold in them hills! Gold, I tell ya!’
It may seem incongruous to compare data—a commodity of which there is an overwhelming amount, much of which may be noise—to gold, a rare and precious metal. But bear with me. The processes that produce both can be broken down into similar tasks. First, there’s the discovery process, then the extraction, then the smelting process that turns raw materials into something beautiful and valuable.
Twenty years ago, while hiking the West Highland Way through Scotland, on the northern outskirts of Tyndrum, I met a man panning for gold. Every year in the springtime, he would book time off work, drive his camper van into the highlands, park up on the roadside or in a campsite next to a river, and spend two weeks thigh-deep in icy water hunting for the precious metal. One week in, he had panned enough gold dust to half-fill a small glass vial—perhaps enough to make a gold tooth or two.
But with gold trading around $350 an ounce in 2003, it was enough to pay for his gas, food, and maybe a couple of therapy sessions to counteract the terrifying impact of nightly attacks on his van by herds of marauding Haggis. That’s the discovery process: it’s about exploration and extraction. It’s hard, dirty work—and that’s not even talking about industrial mining, the heavy machinery involved, not to mention the explosives.
Having extracted the gold dust (or, if you’re lucky, nuggets), it then goes through several chemical processes to refine and cleanse it, and is then smelted into bars, bought, sold, and eventually turned into something tiny yet exquisite that you may have to sell a kidney for in return for an “I do.”
Those who work on either end of the gold process realize that it’s hard, if not impractical, to do both yourself. And while I’ve used gold as an example, the same is true in many industries. For example, the farmer raising pigs is rarely the same person as the butcher or the supermarket where you buy your sausages.
Some are taking a new approach: instead of focusing their efforts on selling data, they are focusing on surfacing datasets, and making them more visible to a financial audience
People working with alt data have come to the same realization: it’s not easy to create data and sell it. It’s even harder to operate a marketplace of alternative datasets, and harder still to map those datasets to each other and to other market data such as stocks in a way that makes the alt data useful. So, some are taking a new approach: instead of focusing their efforts on selling data, they are focusing on surfacing datasets, and making them more visible to a financial audience.
That’s precisely what Matt Ober is doing with his new venture, Initial Data Offering. The former co-head of data strategy at WorldQuant and chief data scientist at investment manager Third Point founded Initial Data Offering to provide a centralized resource to raise the visibility of new and interesting datasets.
IDO won’t be a repository of data itself or a marketplace, but rather will provide curated descriptions of datasets with links to their creators’ websites, as well as a forward-looking calendar of new datasets, which would allow data consumers to budget better and alert them to when they should call their vendor account reps about upcoming datasets.
“I always thought Product Hunt was cool for Silicon Valley… and then there’s G2,” Ober says, referring to websites that cover new technologies and provide peer reviews of business software, respectively. “But in the data space, you’ve never had that place where a vendor can announce to the world that they have new products or updates. And just like how there are calendars for IPOs, there should be a calendar for data.”
Discoverability is also a key tenet of another startup, Peer Data. Founded by former senior IHS Markit executive Kiet Tran and Kat Tatochenko, who has previously held senior sales and business development roles at MarketAxess, Bloomberg, and Thomson Reuters, Peer Data’s website says the company will help improve data discoverability, allow companies to unlock the value of the data they produce and help them monetize it, and—intriguingly—allow investors to invest directly in the data itself. I’m not 100% clear on that last part yet, but it sounds intriguing. More to come on that soon—stay tuned.
And speaking of well-known industry faces, you might be interested to learn what Jeremy Baksht is up to now: we last wrote about the former global head of alternative data at Bloomberg when he joined Walmart’s Data Ventures business line as head of strategy. Now, he’s the co-founder and CEO of Catena Clearing, a startup backed by VC firm Shaper Capital, which aims to make the supply chain for retail goods and stores as automated and data-rich as the supply chain for data in financial markets.
“Corporations still have lots of data gaps, and they don’t know how to fill them,” Baksht says. More importantly, retailers may have big gaps on their shelves and may not know why, or when new stock will arrive to fill them. And, especially relating to perishable groceries, if you don’t know where your bananas are, or how long they’ve spent at a storage facility or in transit, you’re going to end up needing to throw them all out anyway.
Catena aims to build a portfolio of repeatable connectors to interconnect all elements of the retail supply chain, from manufacturers, logistics firms to retailers.
“We want to connect all these elements to give retailers a view of ‘Where’s my stuff’,” says Catena chief technology officer Mike Goynes. “For example, we’ll get data from all shipping companies and networks, pull that in and normalize it, so that a company can understand where all its inventory is, and I handle the ‘munging.’ So, I can get them the information faster, they can make better decisions, and they can get stuff on the shelves.”
Sharp-eyed readers will have no doubt discerned that Catena’s ambition isn’t to fuel financial models—indeed, its immediate financial uses could be enabling companies to use tracking applications to understand where goods are before issuing a letter of credit—but rather to optimize supply and demand of retail goods, keep the shelves stocked and the popular brands front and center.
However, that doesn’t mean there isn’t a play for the data exhaust from this initiative in capital markets. If you look at a supermarket shelf and find it empty, does that mean the store has successfully sold out all of that product, or that they haven’t restocked or ordered—or ordered but haven’t received a timely delivery of—fresh supplies?
Would it be useful for analysts, traders and portfolio managers to know the status of that supermarket shelf? Even better, would it be useful for traders to know in advance which store is going to sell out first, and which will or won’t receive replacement stock in time, based on real-time inventory and delivery supply chain information?
And Baksht isn’t the only one targeting data clients beyond capital markets. Ober is aiming for a 50/50 split between financial and non-financial consumers, and says the majority of datasets on IDO so far derive 95% of their revenues from outside financial services—though he adds that hedge funds are interested in all datasets.
Indeed, though not focusing on it, Baksht isn’t ruling out interest from the financial world. “In the world of alternative data, there are still so many challenges to solve,” he says. “Hedge funds would rather go to a shipping or logistics conference and find something new than go to one of these big alt data events and see the same thing that everyone else sees.”
If you’re using a new or unusual dataset, we’d love to hear about it. You can email me at max.bowie@infopro-digital.com.
Only users who have a paid subscription or are part of a corporate subscription are able to print or copy content.
To access these options, along with all other subscription benefits, please contact info@waterstechnology.com or view our subscription options here: http://subscriptions.waterstechnology.com/subscribe
You are currently unable to print this content. Please contact info@waterstechnology.com to find out more.
You are currently unable to copy this content. Please contact info@waterstechnology.com to find out more.
Copyright Infopro Digital Limited. All rights reserved.
As outlined in our terms and conditions, https://www.infopro-digital.com/terms-and-conditions/subscriptions/ (point 2.4), printing is limited to a single copy.
If you would like to purchase additional rights please email info@waterstechnology.com
Copyright Infopro Digital Limited. All rights reserved.
You may share this content using our article tools. As outlined in our terms and conditions, https://www.infopro-digital.com/terms-and-conditions/subscriptions/ (clause 2.4), an Authorised User may only make one copy of the materials for their own personal use. You must also comply with the restrictions in clause 2.5.
If you would like to purchase additional rights please email info@waterstechnology.com
More on Data Management
New working group to create open framework for managing rising market data costs
Substantive Research is putting together a working group of market data-consuming firms with the aim of crafting quantitative metrics for market data cost avoidance.
Off-channel messaging (and regulators) still a massive headache for banks
Waters Wrap: Anthony wonders why US regulators are waging a war using fines, while European regulators have chosen a less draconian path.
Back to basics: Data management woes continue for the buy side
Data management platform Fencore helps investment managers resolve symptoms of not having a central data layer.
‘Feature, not a bug’: Bloomberg makes the case for Figi
Bloomberg created the Figi identifier, but ceded all its rights to the Object Management Group 10 years ago. Here, Bloomberg’s Richard Robinson and Steve Meizanis write to dispel what they believe to be misconceptions about Figi and the FDTA.
SS&C builds data mesh to unite acquired platforms
The vendor is using GenAI and APIs as part of the ongoing project.
Aussie asset managers struggle to meet ‘bank-like’ collateral, margin obligations
New margin and collateral requirements imposed by UMR and its regulator, Apra, are forcing buy-side firms to find tools to help.
Where have all the exchange platform providers gone?
The IMD Wrap: Running an exchange is a profitable business. The margins on market data sales alone can be staggering. And since every exchange needs a reliable and efficient exchange technology stack, Max asks why more vendors aren’t diving into this space.
Reading the bones: Citi, BNY, Morgan Stanley invest in AI, alt data, & private markets
Investment arms at large US banks are taken with emerging technologies such as generative AI, alternative and unstructured data, and private markets as they look to partner with, acquire, and invest in leading startups.