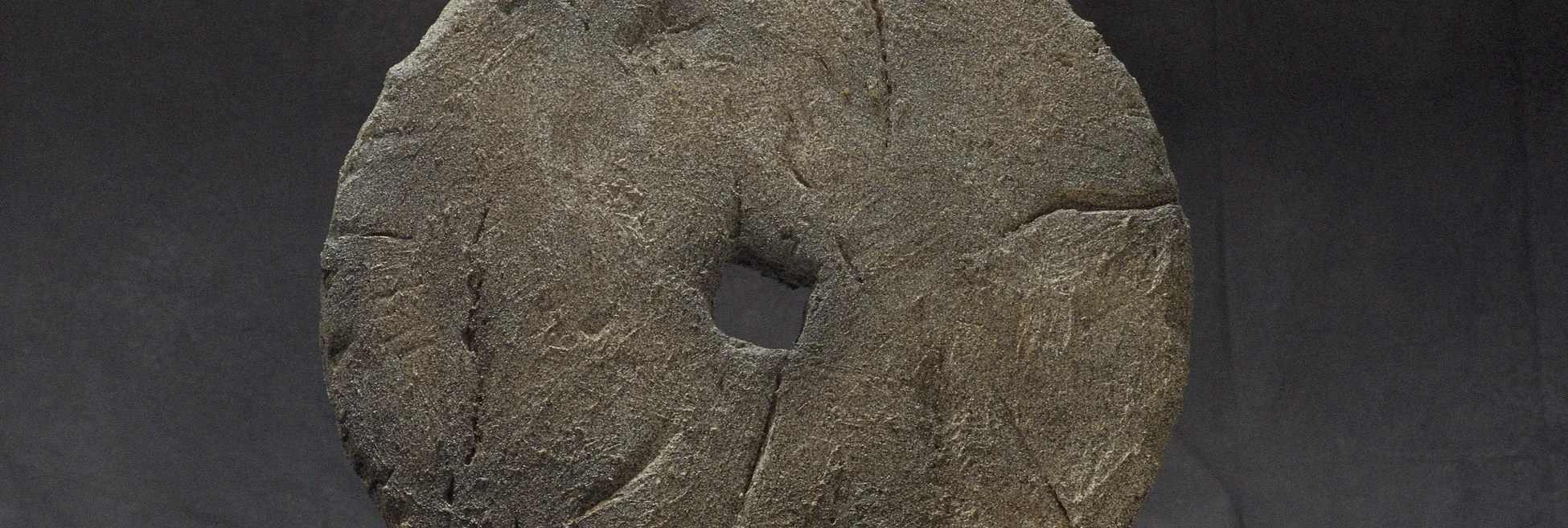
The Rise of the Algo Wheel
An examination as to how the buy side is embracing algo wheels and where the challenges still remain.
Buy-side firms are evolving the techniques they use to select and validate broker-dealers and their algorithms to improve execution performance while providing evidence of their effectiveness. As a result, an increasing number of asset managers and quantitative funds are looking to algo wheels to automate the routing process and determine where is best to send their easier-to-execute orders, thus allowing traders to focus on more complex trades for execution.
One of the key drivers for using algo wheels or developing technologies for broker routing is regulation. Over the last two years, buy-side firms have taken on greater responsibility for best execution, where they are mandated to justify their broker selection more transparently. A top example of this is the RTS 28 requirement under Europe’s Mifid II overhaul, where investment firms must publish and report their best execution practices.
But regulation isn’t the only driver. Others include cost pressures, the need to improve efficiency and to optimize execution strategies, all while managing an increasing number of complex sell-side algorithms. These factors have all contributed to the growing investment in independent technologies that can analyze the performance of a broker’s algorithms and then use that data to train its algo wheel.
The days of clients relying on banks to tell them how things perform are, broadly speaking, long gone.
Bank executive
“The days of clients relying on banks to tell them how things perform are, broadly speaking, long gone,” a head of execution services at a tier-1 bank says. “Because of best-execution requirements that our clients have to their end investors, they typically need to have a pretty robust, independent process for evaluating broker performance.”
According to a report published by Greenwich Associates in April 2019, less than a quarter of buy-side traders in Europe use algo wheels, but that number is rapidly growing, particularly as traders look to shift meaningful amounts of their trading from traditional high-touch trades to algorithmic trading.
Others believe many of the advancements and uptake in broker-routing technologies have also been helped along by cloud computing and the availability of data. Today, buy-side firms are more data-driven and systematic in their approach to execution.
Yet algo wheels and broker-routing technologies are very much at the early stages of development for buy-side firms and much of the activity is still taking place behind closed doors. Many are reluctant to discuss the mechanics of their algo wheel development as they continue to fine-tune their mathematical models. Additionally, a growing number of third-party vendors—such as FlexTrade, ITG and Itiviti—are offering algo wheel services. Vendors tend to couple these services with other broker-performance analytics, such as transaction-cost analysis (TCA).
This move toward more sophisticated broker analysis means there is increasing pressure on sell-side firms to prove their worth and stand out in an already competitive market. One example of this is UBS. Over the last two years, the Swiss bank has been building out its algo validation team focused on strengthening its algo inventory and reducing the risk of flawed models.
“Since brokers algos are continuously reviewed for performance, and there are now more sophisticated, systematic means to do so, pressure on the sell side has increased to ensure their algorithms are increasingly optimised so that they capture as much flow as possible,” says Alex Brown, head of product for principal trading at Itiviti.
But, as the algo wheels and broker-routing technologies continue to evolve, a variety of challenges are beginning to emerge. For this piece, WatersTechnology spoke to several tier-1 banks, vendors and buy-side experts to gain a deeper understanding of some of the lead concerns in this space.
Not an Exact Science
An algo wheel is a piece of software that uses a performance-weighted model of broker-routing. It is a measurement tool that can be built into an execution management system (EMS) or order management system (OMS) to help determine the most optimal broker or sell-side algorithm at any given time. It uses decision-making logic, meaning that it automates order-routing flow according to a set of rules and mathematical models. Using quantitative data such as TCA reports, raw trade data and other real-time market data factors, the algo wheel ranks an interchangeable list of brokers based on the inputted logic—considering metrics such as market cap, spread capture, percentage average volume (ADV), volatility and time of day.
Once the process is completed, the algo wheel generates a report explaining and justifying the reasons for selecting the broker algo. It provides a best execution audit trail making it valuable for complying with buy-side execution requirements.
Algo wheels can also be divided into two categories: a tactical algo wheel and a performance-based algo wheel. A tactical algo wheel follows a defined set of if/then instructions, whereas a performance-based algo can adapt to real-time data and market conditions, modifying routing strategies accordingly throughout the day.
The challenges, however, come down to the technical engineering of the algo wheel. According to the bank head of execution services, there are many moving parts to consider when building models for execution analysis.
“The problem with execution analysis is that it can be more of an art than a science,” says the source. “Arriving at statistically meaningful conclusions on trading performance of your brokers is difficult—the datasets are often too small to be statistically significant, and if you extend the time horizon, you can end up mixing performance in different market environments.”
In many cases, buy-side firms require several months’ worth of data on a broker’s algo performance to sufficiently draw a conclusion, but often the results can be skewed by changing market conditions and outliers. So, for example: Broker A trades an order on a day with optimal market conditions, whereas Broker B trades the same order on a volatile day. This example inaccurately reflects the brokers’ overall performance because it only looks at a single day. When building algo wheels, firms need to consider multiple complex factors that can influence the result of the execution analysis, including uncontrollable market conditions, benchmarks, order-routing objectives, statistical interpretation and slippage calculations.
Some buy-side participants only want the granular raw data of the execution, so they ingest that raw data themselves or give this data to a third-party company that evaluates it and makes the evaluation of the performance about our algo and others.
Paris Pennesi, HSBC
According to one former head of trading at a tier-1 bank, many algo wheels being developed are flawed because of the complexity of trying to create mathematical instructions for each outlier.
They add: “If the slippage numbers are valid, the routing algo will work perfectly well, but because most slippage calculations are flawed because they include market noise—typically an order of magnitude larger than the actual slippage—the routing algo will be working off bad data.”
Other considerations point to the type of data used to inform the algo wheels or execution analysis. For example, there are those who believe that some TCA reports include data that is biased or data that only shows a fraction of a broker’s algo performance. The former head of trading explains that TCA reports are based on distorted calculations, as a lot of market noise is captured in the process.
In some cases, buy-side firms are now requesting raw data from agency brokers to produce more accurate reflections of execution performance, adds Paris Pennesi, head of quant strategies for foreign exchange and commodities for HSBC’s eRisk unit. “Some buy-side participants only want the granular raw data of the execution, so they ingest that raw data themselves or give this data to a third-party company that evaluates it and makes the evaluation of the performance about our algo and others,” he says.
To add to the complexity, subjectivity and customization requirements also play a role. Each buy-side trader will have different order types, specifications, trading techniques, and interpretations of factors, such as outliers, execution success and benchmarks—and translating those into a performance-measurement tool can be incredibly difficult.
“I don’t think it is an exact science and clearly whatever your results are, they do determine how your automated algo wheel will allocate those orders,” the head of execution services says. “So, I think it is important for clients to be aware that it is not perfect and there are subjective factors that need to be brought into the equation.”
According to one execution services specialist who is based in Europe, it is also at a major disadvantage to the US from a data perspective. The reason is that there is no consolidated tape or holistic view of a European best bid and offer (EBBO) in the EU, firms are having to construct their own tape or gain access to the data through multiple sources—in which case the prices may be inconsistently calculated across other firms or vendors.
“Comparing apples with oranges becomes a little bit difficult at times,” they say.
Best Execution
Historically, much of the pressure of best execution has largely been on sell-side firms. But that changed in January 2018 with the implementation of Mifid II and its RTS 28 requirements, in which buy-side firms are mandated to evidence best execution. The obligations require investment firms to categorize the top five execution venues and entities they use across all asset classes, as well as publish and report their execution policy to regulators in a machine-readable format.
The point of the regulation was to provide a new level of accountability and transparency to the buy side. In its design, however, RTS 28 did not envisage the uptake of automated broker-routing or algo wheels—where some believe that buy-side firms are effectively offloading their best execution responsibilities to the sell side as brokers look to be ranked favorably in their models. There is also growing concern about the complexity of building an algo wheel and offering best-execution reports to investors and regulators that can be easily understood and consumed.
“It is too complex for the buy side to do it right; it will be even more complex for the regulator to figure out if it is right or not,” says the former head of trading.
With the pressure buy-side firms face to ensure quality execution and justify each decision, leaving that up to an automated algo can cause a few hurdles of its own. One example, in particular, is if there is a loss in tech talent, traders or knowledge within the firm, which are used to program the machine’s routing orders. Other future consideration are the application of machine learning to make for more intelligent broker routing. Where that fits into the equation of explainability and best-execution reports has yet to be seen.
It is unclear if algo wheels or execution analysis systems will become more scrutinized in the future by regulators, at least in the EU. As much of Mifid execution regulation is principles-based, regulators may accept execution policies and reports that incorporate algo wheels as part of their best execution practices and regulatory reports.
But the whims of regulators have been known to change.
Only users who have a paid subscription or are part of a corporate subscription are able to print or copy content.
To access these options, along with all other subscription benefits, please contact info@waterstechnology.com or view our subscription options here: http://subscriptions.waterstechnology.com/subscribe
You are currently unable to print this content. Please contact info@waterstechnology.com to find out more.
You are currently unable to copy this content. Please contact info@waterstechnology.com to find out more.
Copyright Infopro Digital Limited. All rights reserved.
As outlined in our terms and conditions, https://www.infopro-digital.com/terms-and-conditions/subscriptions/ (point 2.4), printing is limited to a single copy.
If you would like to purchase additional rights please email info@waterstechnology.com
Copyright Infopro Digital Limited. All rights reserved.
You may share this content using our article tools. As outlined in our terms and conditions, https://www.infopro-digital.com/terms-and-conditions/subscriptions/ (clause 2.4), an Authorised User may only make one copy of the materials for their own personal use. You must also comply with the restrictions in clause 2.5.
If you would like to purchase additional rights please email info@waterstechnology.com
More on Data Management
New working group to create open framework for managing rising market data costs
Substantive Research is putting together a working group of market data-consuming firms with the aim of crafting quantitative metrics for market data cost avoidance.
Off-channel messaging (and regulators) still a massive headache for banks
Waters Wrap: Anthony wonders why US regulators are waging a war using fines, while European regulators have chosen a less draconian path.
Back to basics: Data management woes continue for the buy side
Data management platform Fencore helps investment managers resolve symptoms of not having a central data layer.
‘Feature, not a bug’: Bloomberg makes the case for Figi
Bloomberg created the Figi identifier, but ceded all its rights to the Object Management Group 10 years ago. Here, Bloomberg’s Richard Robinson and Steve Meizanis write to dispel what they believe to be misconceptions about Figi and the FDTA.
SS&C builds data mesh to unite acquired platforms
The vendor is using GenAI and APIs as part of the ongoing project.
Aussie asset managers struggle to meet ‘bank-like’ collateral, margin obligations
New margin and collateral requirements imposed by UMR and its regulator, Apra, are forcing buy-side firms to find tools to help.
Where have all the exchange platform providers gone?
The IMD Wrap: Running an exchange is a profitable business. The margins on market data sales alone can be staggering. And since every exchange needs a reliable and efficient exchange technology stack, Max asks why more vendors aren’t diving into this space.
Reading the bones: Citi, BNY, Morgan Stanley invest in AI, alt data, & private markets
Investment arms at large US banks are taken with emerging technologies such as generative AI, alternative and unstructured data, and private markets as they look to partner with, acquire, and invest in leading startups.